Multi-encoder U-Net for Oral Squamous Cell Carcinoma Image Segmentation
2022 IEEE INTERNATIONAL SYMPOSIUM ON MEDICAL MEASUREMENTS AND APPLICATIONS (MEMEA 2022)(2022)
摘要
Oral tumors are responsible for about 170,000 deaths every year in the World. In this paper, we focus on oral squamous cell carcinoma (OSCC), which represents up to 80-90% of all malignant neoplasms of the oral cavity. We present a novel deep learning-based method for segmenting whole slide image (WSI) samples at the pixel level. The proposed method is a modification of the well-known U-Net architecture through a multi-encoder structure. In particular, our network, called Multi encoder U-Net, is a multi-encoder single decoder network that takes as input an image and splits it in tiles. For each tile, there is an encoder responsible for encoding it in the latent space, then a convolutional layer is responsible for merging the tiles into a single layer. Each layer of the decoder takes as input the previous up-sampled layer and concatenate it with the layer made by merging the corresponding layers of the multiple encoders. Experiments have been carried out on the publicly available ORal Cancer Annotated (ORCA) dataset, which contains annotated data from the TCGA repository. Quantitative experimental results, obtained using three different quality metrics, demonstrate the effectiveness of the proposed approach, which achieves 82% Pixel-wise Accuracy, 0.82 Dice similarity score, and 0.72 Mean Intersection Over Union.
更多查看译文
关键词
Medical imaging, tumor segmentation, deep learning
AI 理解论文
溯源树
样例
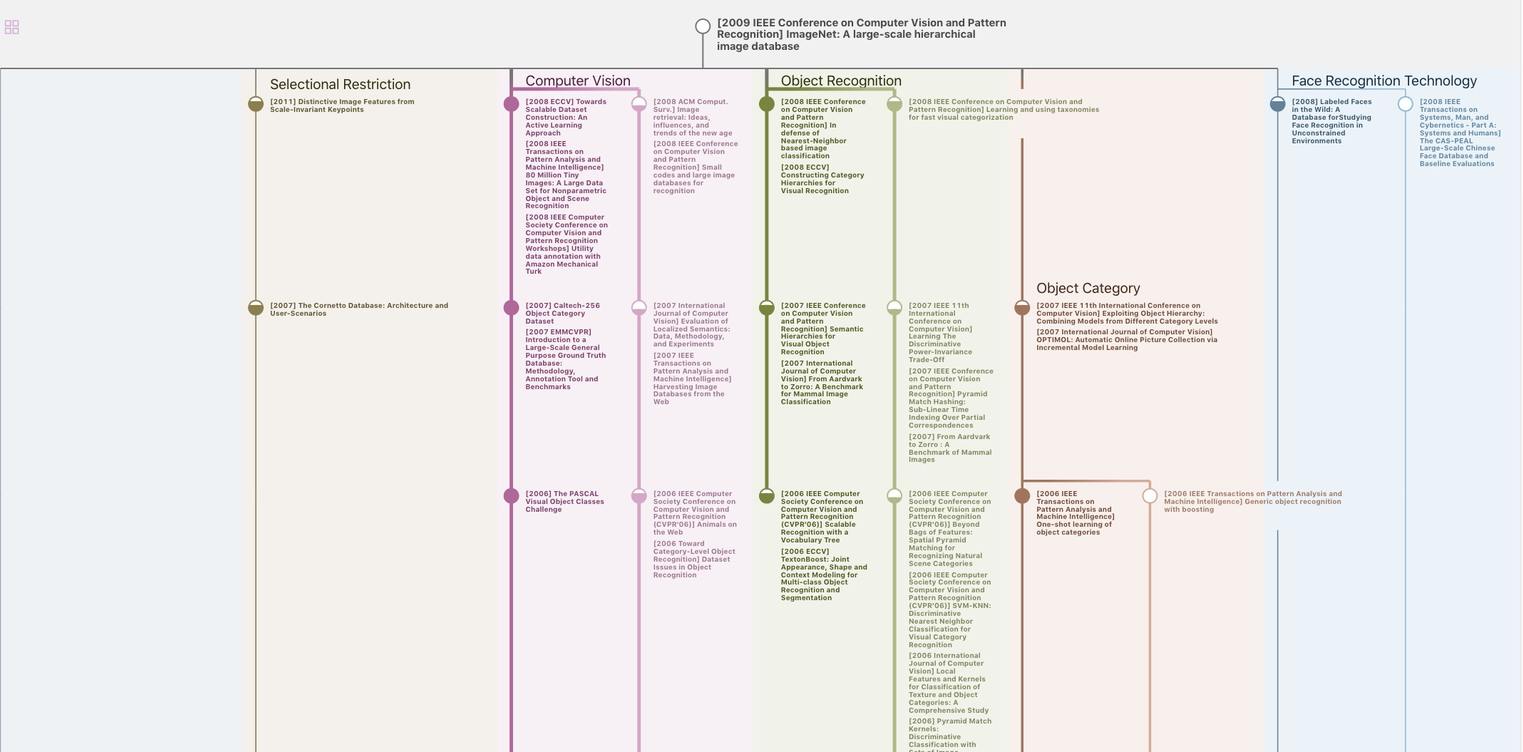
生成溯源树,研究论文发展脉络
Chat Paper
正在生成论文摘要