Learning-based Local Path Planning for UAV in Unknown Environments
2022 EUROPEAN CONTROL CONFERENCE (ECC)(2022)
摘要
This paper develops a novel learning-based local path planning method for Unmanned Aerial Vehicles (UAVs) in unknown environments. We establish a neural network (NN) with two fully connected hidden layers, where the distances from the UAV to the hit points of the locally detected obstacles, a strategic temporary goal and the direction to the final destination are selected as the input of the NN, and the reference velocity for the UAV to track is chosen as the output. To collect the training data, we propose a local path planning method, which repeatedly constructs a local Laplacian Potential Field (LPF) only based on the UAV's real-time obstacle detections of limited scope, and requires the UAV to track the negative gradient direction of the resulting potential function. Then, the UAV follows the reference velocity generated by the trained NN path planner to safely approach the final destination. Simulations demonstrate the effectiveness, adaptability, and efficiency of the proposed learning-based path planning method, which outperforms the above LPF-based path planning method and, unlike many other learning-based methods, does not need to re-train the NN parameters when changed to new maps.
更多查看译文
关键词
trained NN path planner,final destination,learning-based path,learning-based methods,learning-based local path planning method,Unmanned Aerial Vehicles,fully connected hidden layers,locally detected obstacles,reference velocity,local Laplacian Potential Field,UAV's real-time obstacle detections
AI 理解论文
溯源树
样例
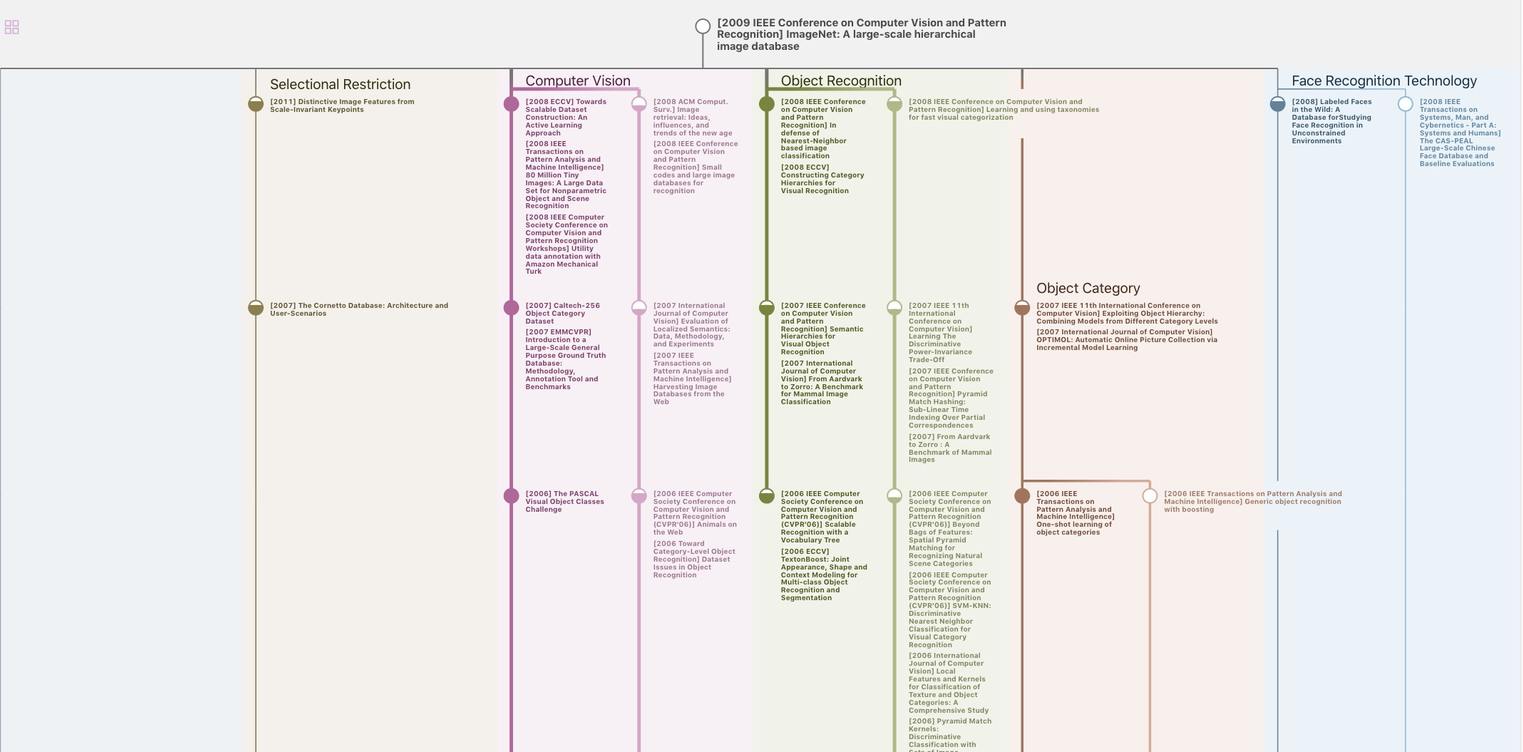
生成溯源树,研究论文发展脉络
Chat Paper
正在生成论文摘要