Accurate Ito-Taylor-Discretization-Based State Estimation in Stochastic Neural Field Equations with Infinite Signal Transmission Rate
2022 EUROPEAN CONTROL CONFERENCE (ECC)(2022)
摘要
In this paper, we explore the Dynamic Neural Fields (DNFs) in the presence of model uncertainties, i.e. the stochastic DNF equations. The model describes a neural tissue affected by external stimulus where the interaction of neurons population is treated as a continuum. This yields a stochastic nonlinear integro-differential equation. The working memory mechanism modeled by the DNFs should allow the system to cope with missing sensors' information. The goal of this paper is to design an accurate reconstruction methodology of the pattern formation in a neural tissue from an incomplete data available from the sensors. We assume that the measurements are partially observed in both domains that are the time and the spatial domains. For that, we explain how the state-space representation can be adopted for the stochastic DNFs and, next, we derive the filtering method based on the Ito-Taylor expansion of order 1.5 within the Extended Kalman Filter. The numerical experiments are also provided.
更多查看译文
关键词
accurate Itô-Taylor-discretization-based state estimation,stochastic Neural field equations,infinite signal transmission rate,Dynamic Neural Fields,stochastic DNF equations,neural tissue,external stimulus,neurons population,stochastic nonlinear integro-differential equation,working memory mechanism,state-space representation,stochastic DNFs,Itô-Taylor expansion
AI 理解论文
溯源树
样例
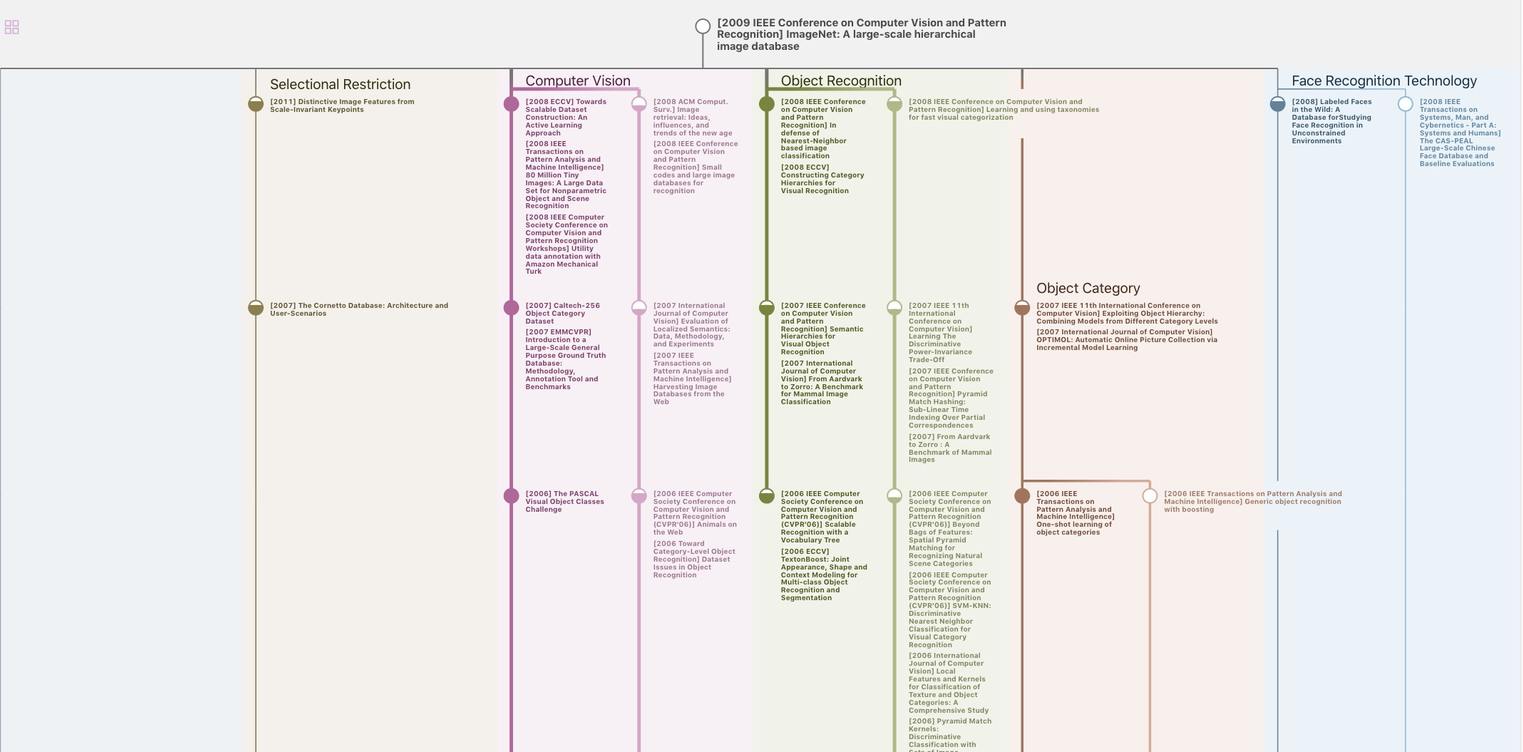
生成溯源树,研究论文发展脉络
Chat Paper
正在生成论文摘要