Controlling Commercial Cooling Systems Using Reinforcement Learning
arxiv(2022)
摘要
This paper is a technical overview of DeepMind and Google's recent work on reinforcement learning for controlling commercial cooling systems. Building on expertise that began with cooling Google's data centers more efficiently, we recently conducted live experiments on two real-world facilities in partnership with Trane Technologies, a building management system provider. These live experiments had a variety of challenges in areas such as evaluation, learning from offline data, and constraint satisfaction. Our paper describes these challenges in the hope that awareness of them will benefit future applied RL work. We also describe the way we adapted our RL system to deal with these challenges, resulting in energy savings of approximately 9% and 13% respectively at the two live experiment sites.
更多查看译文
关键词
reinforcement learning,commercial cooling systems
AI 理解论文
溯源树
样例
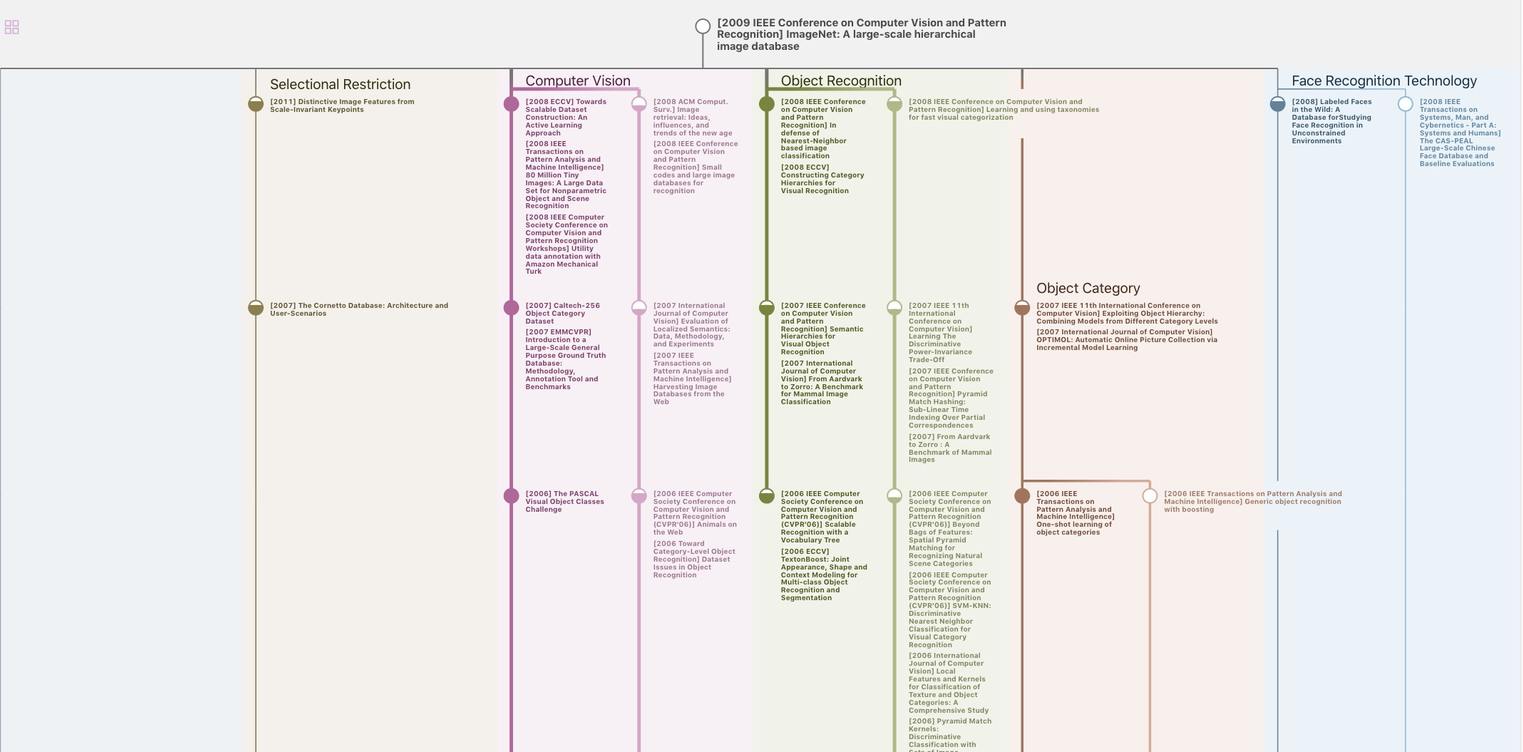
生成溯源树,研究论文发展脉络
Chat Paper
正在生成论文摘要