The Role of Local Alignment and Uniformity in Image-Text Contrastive Learning on Medical Images
arxiv(2023)
摘要
Image-text contrastive learning has proven effective for pretraining medical image models. When targeting localized downstream tasks like semantic segmentation or object detection, additional local contrastive losses that align image regions with sentences have shown promising results. We study how local contrastive losses are related to global (per-sample) contrastive losses and which effects they have on localized medical downstream tasks. Based on a theoretical comparison, we propose to remove some components of local losses and replace others by a novel distribution prior which enforces uniformity of representations within each sample. We empirically study this approach on chest X-ray tasks and find it to be very effective, outperforming methods without local losses on 12 of 18 tasks.
更多查看译文
关键词
local alignment,medical images,learning,image-text
AI 理解论文
溯源树
样例
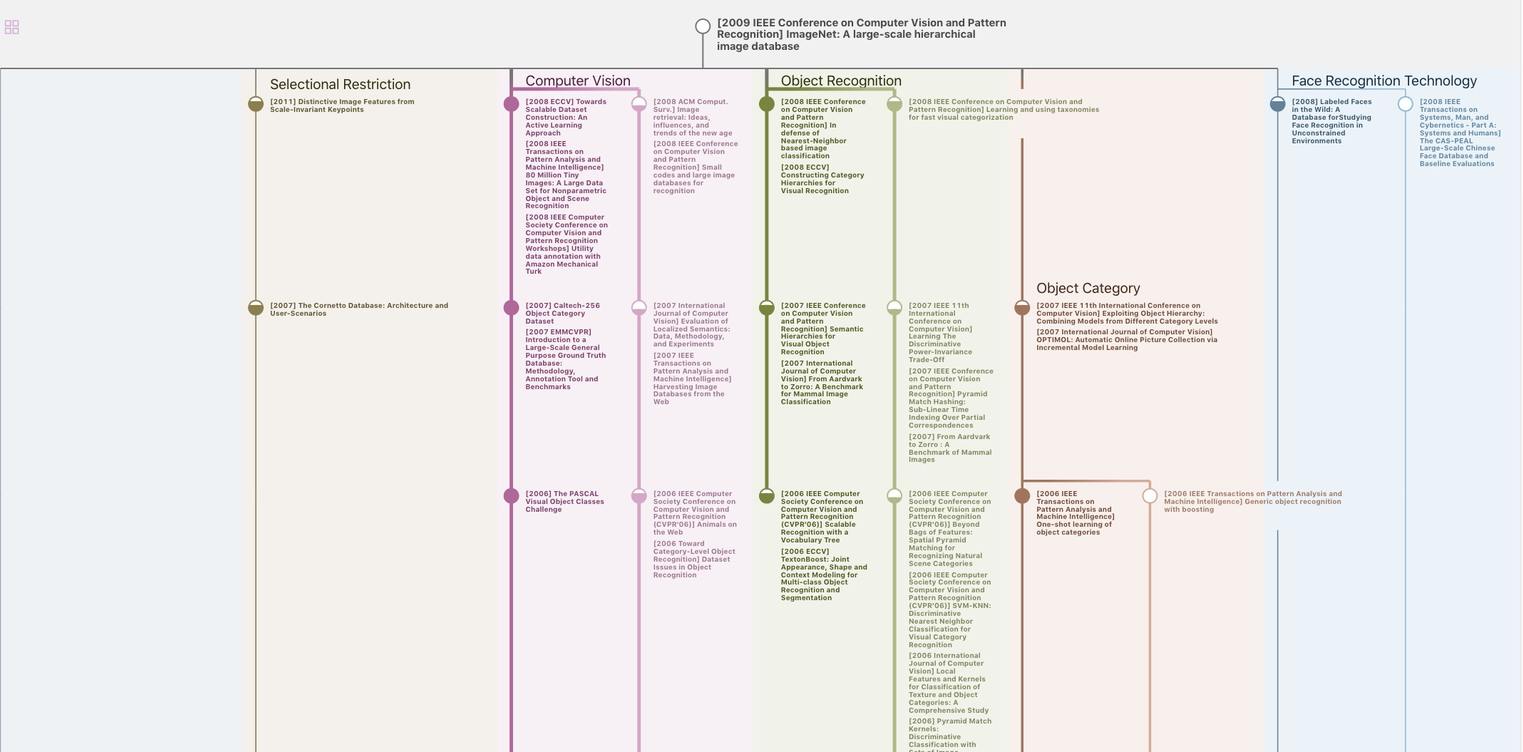
生成溯源树,研究论文发展脉络
Chat Paper
正在生成论文摘要