C3: Cross-instance guided Contrastive Clustering
arxiv(2022)
摘要
Clustering is the task of gathering similar data samples into clusters without using any predefined labels. It has been widely studied in machine learning literature, and recent advancements in deep learning have revived interest in this field. Contrastive clustering (CC) models are a staple of deep clustering in which positive and negative pairs of each data instance are generated through data augmentation. CC models aim to learn a feature space where instance-level and cluster-level representations of positive pairs are grouped together. Despite improving the SOTA, these algorithms ignore the cross-instance patterns, which carry essential information for improving clustering performance. In this paper, we propose a novel contrastive clustering method, Cross-instance guided Contrastive Clustering (C3), that considers the cross-sample relationships to increase the number of positive pairs. In particular, we define a new loss function that identifies similar instances using the instance-level representation and encourages them to aggregate together. Extensive experimental evaluations show that our proposed method can outperform state-of-the-art algorithms on benchmark computer vision datasets: we improve the clustering accuracy by 6.8%, 2.8%, 4.9%, 1.3% and 0.4% on CIFAR-10, CIFAR-100, ImageNet-10, ImageNet-Dogs, and Tiny-ImageNet, respectively.
更多查看译文
关键词
clustering,cross-instance
AI 理解论文
溯源树
样例
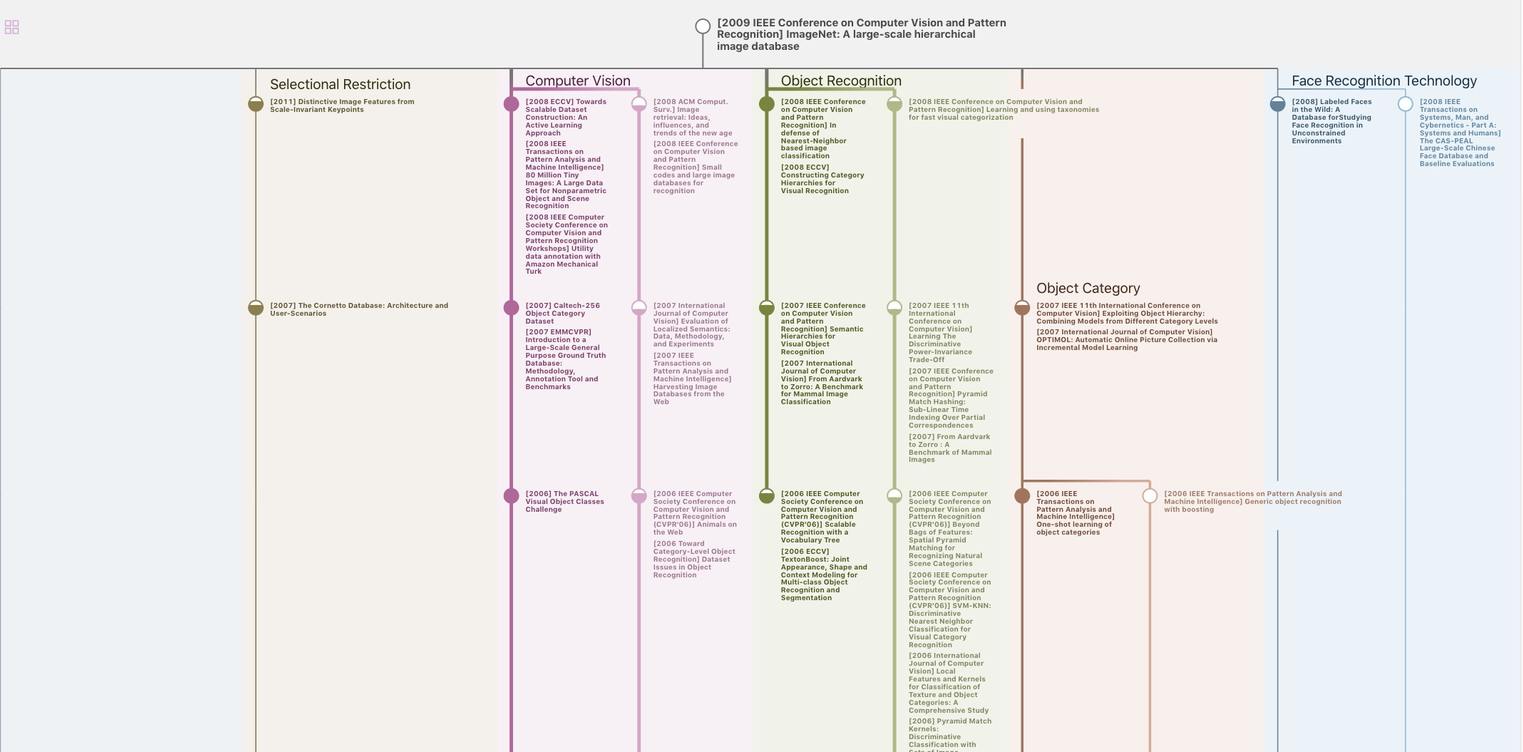
生成溯源树,研究论文发展脉络
Chat Paper
正在生成论文摘要