Stackelberg Meta-Learning Based Control for Guided Cooperative LQG Systems
IFAC PAPERSONLINE(2023)
摘要
Guided cooperation allows intelligent agents with heterogeneous capabilities to work together by following a leader-follower type of interaction. However, the associated control problem becomes challenging when the leader agent does not have complete information about follower agents. There is a need for learning and adaptation of cooperation plans. To this end, we develop a meta-learning-based Stackelberg game-theoretic framework to address the challenges in the guided cooperative control for linear systems. We first formulate the guided cooperation between agents as a dynamic Stackelberg game and use the feedback Stackelberg equilibrium as the agent-wise cooperation strategy. We further leverage meta-learning to address the incomplete information of follower agents, where the leader agent learns a meta-response model from a prescribed set of followers offline and adapts to a new coming cooperation task with a small amount of learning data. We use a case study in robot teaming to corroborate the effectiveness of our framework. Comparison with other learning approaches also shows that our learned cooperation strategy provides better transferability for different cooperation tasks. Copyright (c) 2023 The Authors.
更多查看译文
关键词
Learning for control,Dynamic games,Linear systems,Intelligent robotics,Data-driven control,Adaptive control of multi-agent systems
AI 理解论文
溯源树
样例
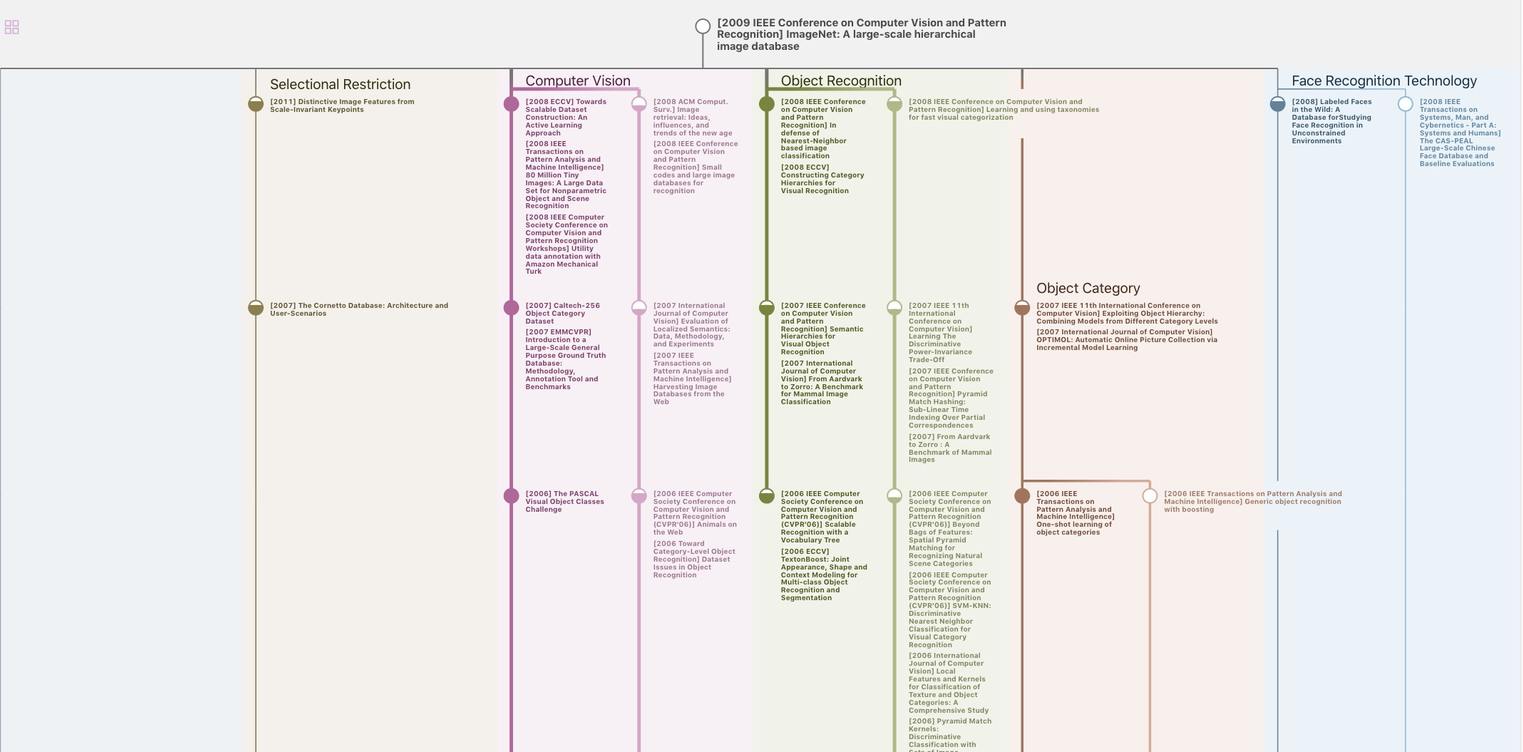
生成溯源树,研究论文发展脉络
Chat Paper
正在生成论文摘要