A Sparse Bayesian Learning Algorithm for Channel Estimation in Wideband mmWave MIMO-OFDM Systems
2022 International Conference on Culture-Oriented Science and Technology (CoST)(2022)
摘要
For millimeter wave (mmWave) multiple-input multiple-output (MIMO) systems, several existing beamspace channel estimation schemes for wideband systems tend to assume that the beamspace channels have common support in the frequency domain. In this case, the validity of the results obtained is limited due to the beam squint effect caused by wideband in practice. In this paper, a multiple-sparse Bayesian learning (M-SBL) algorithm is proposed for channel estimation of wideband mmWave MIMO orthogonal frequency division multiplexing (OFDM) systems. In the absence of a common supporting hypothesis, an empirical Bayesian prior is used to estimate a convenient posterior distribution candidate basis vector to estimate the channel. Simulation analysis shows that the proposed method based on M-SBL can accurately estimate the low-complexity beamspace channel and has smaller normalized mean square error (NMSE) performance than the traditional algorithms. The method based on M-SBL still has better estimation performance when the number of users is large and the number of pilots is small.
更多查看译文
关键词
mmWave,channel estimation,wideband,Bayesian,sparse
AI 理解论文
溯源树
样例
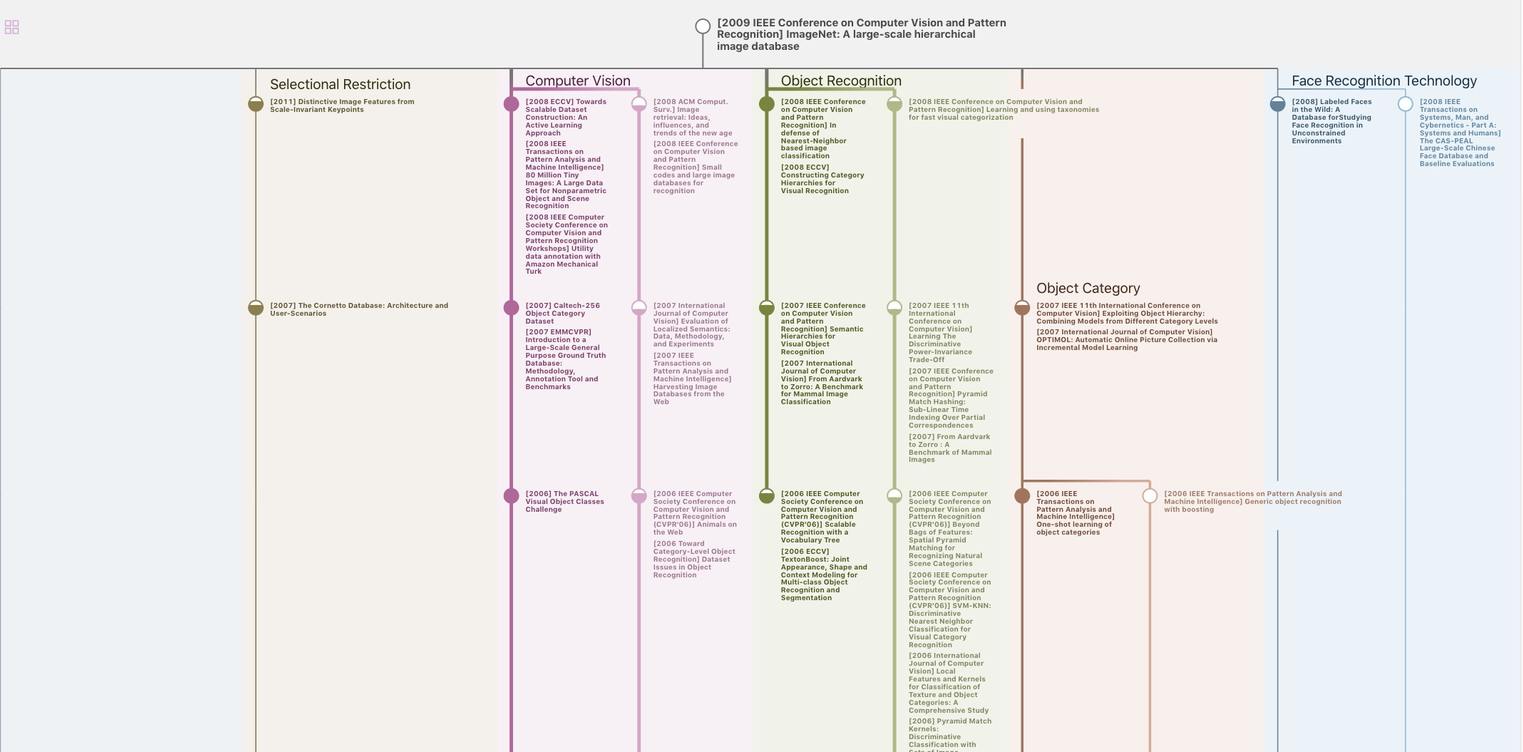
生成溯源树,研究论文发展脉络
Chat Paper
正在生成论文摘要