Malware classification based on visualization and feature fusion
2021 IEEE Sixth International Conference on Data Science in Cyberspace (DSC)(2021)
摘要
With the advancement of computer technology and the continuous development of networks, resulting in a growing number of malware variants, it has become particularly important to classify malware quickly and in a timely manner. In this paper, we propose a visual malware family classification based on deep learning by presenting a binary file as an image and clustering the malware using texture features in the image. Specifically, the bytes and opcodes in the malware binaries are converted into Markov images based on the transfer probability matrix. In this paper, we use deep convolutional neural networks to fuse the features of Markov images generated from bytes and opcodes, and then classify them. The experiments were conducted on the Microsoft malware dataset, and the results show that the accuracy and F-value of the method based on Markov image and opcode image feature fusion are 99.76% and 98.91%, respectively, and effective and information-enhanced classification features can be obtained.
更多查看译文
关键词
malware variants,visual malware family classification,deep learning,binary file,texture features,bytes,malware binaries,Markov image,transfer probability matrix,deep convolutional neural networks,Microsoft malware dataset,opcode image feature fusion,information-enhanced classification features,visualization,computer technology,malware clustering
AI 理解论文
溯源树
样例
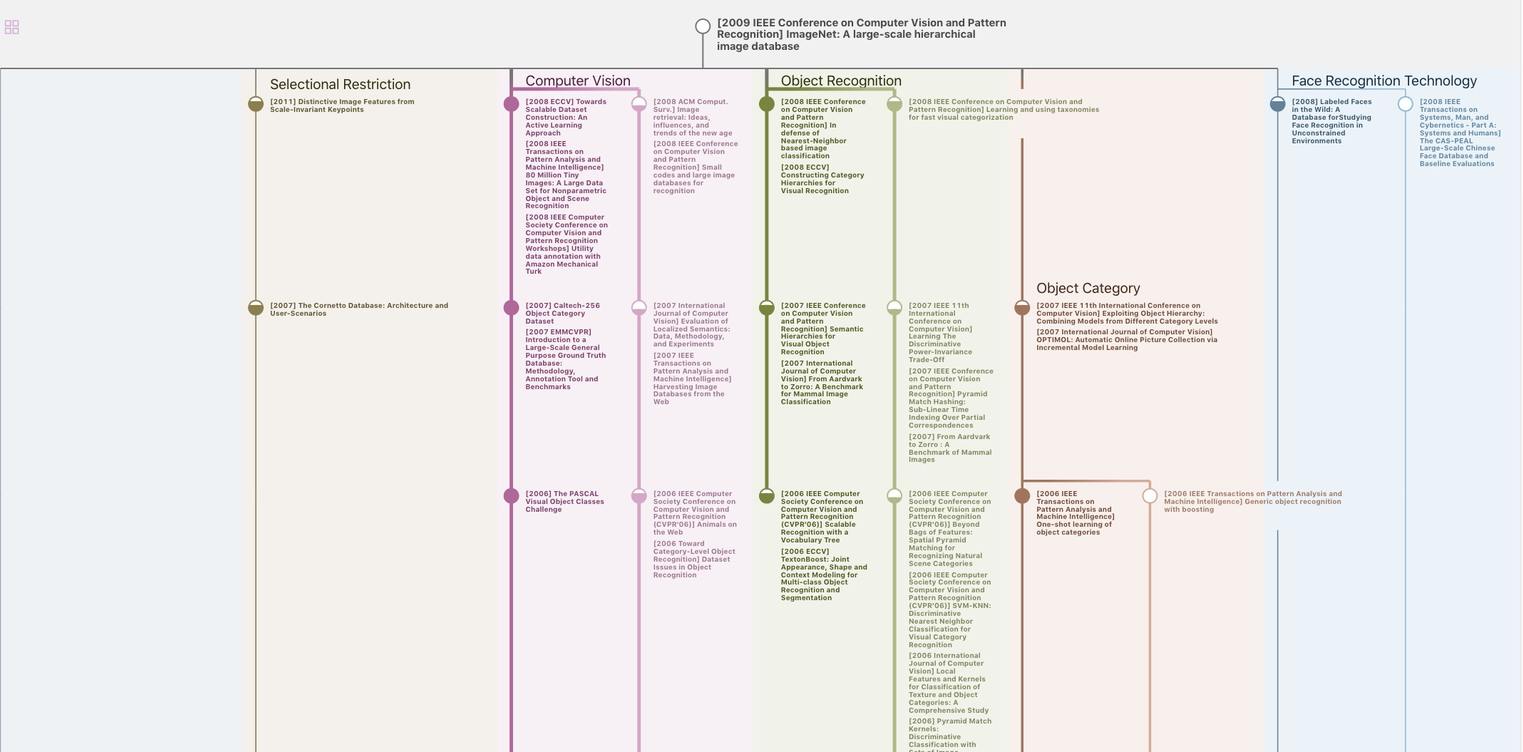
生成溯源树,研究论文发展脉络
Chat Paper
正在生成论文摘要