Unsupervised domain adaptive tumor region recognition for Ki67 automated assisted quantification
International journal of computer assisted radiology and surgery(2022)
摘要
Purpose Ki67 is a protein associated with tumor proliferation and metastasis in breast cancer and acts as an essential prognostic factor. Clinical work requires recognizing tumor regions on Ki67-stained whole-slide images (WSIs) before quantitation. Deep learning has the potential to provide assistance but largely relies on massive annotations and consumes a huge amount of time and energy. Hence, a novel tumor region recognition approach is proposed for more precise Ki67 quantification. Methods An unsupervised domain adaptive method is proposed, which combines adversarial and self-training. The model trained on labeled hematoxylin and eosin (H&E) data and unlabeled Ki67 data can recognize tumor regions in Ki67 WSIs. Based on the UDA method, a Ki67 automated assisted quantification system is developed, which contains foreground segmentation, tumor region recognition, cell counting, and WSI-level score calculation. Results The proposed UDA method achieves high performance in tumor region recognition and Ki67 quantification. The AUC reached 0.9915, 0.9352, and 0.9689 on the validation set and internal and external test sets, respectively, substantially exceeding baseline (0.9334, 0.9167, 0.9408) and rivaling the fully supervised method (0.9950, 0.9284, 0.9652). The evaluation of automated quantification on 148 WSIs illustrated statistical agreement with pathological reports. Conclusion The model trained by the proposed method is capable of accurately recognizing Ki67 tumor regions. The proposed UDA method can be readily extended to other types of immunohistochemical staining images. The results of automated assisted quantification are accurate and interpretable to provide assistance to both junior and senior pathologists in their interpretation.
更多查看译文
关键词
Unsupervised domain adaption,Tumor region recognition,Breast cancer,Deep learning
AI 理解论文
溯源树
样例
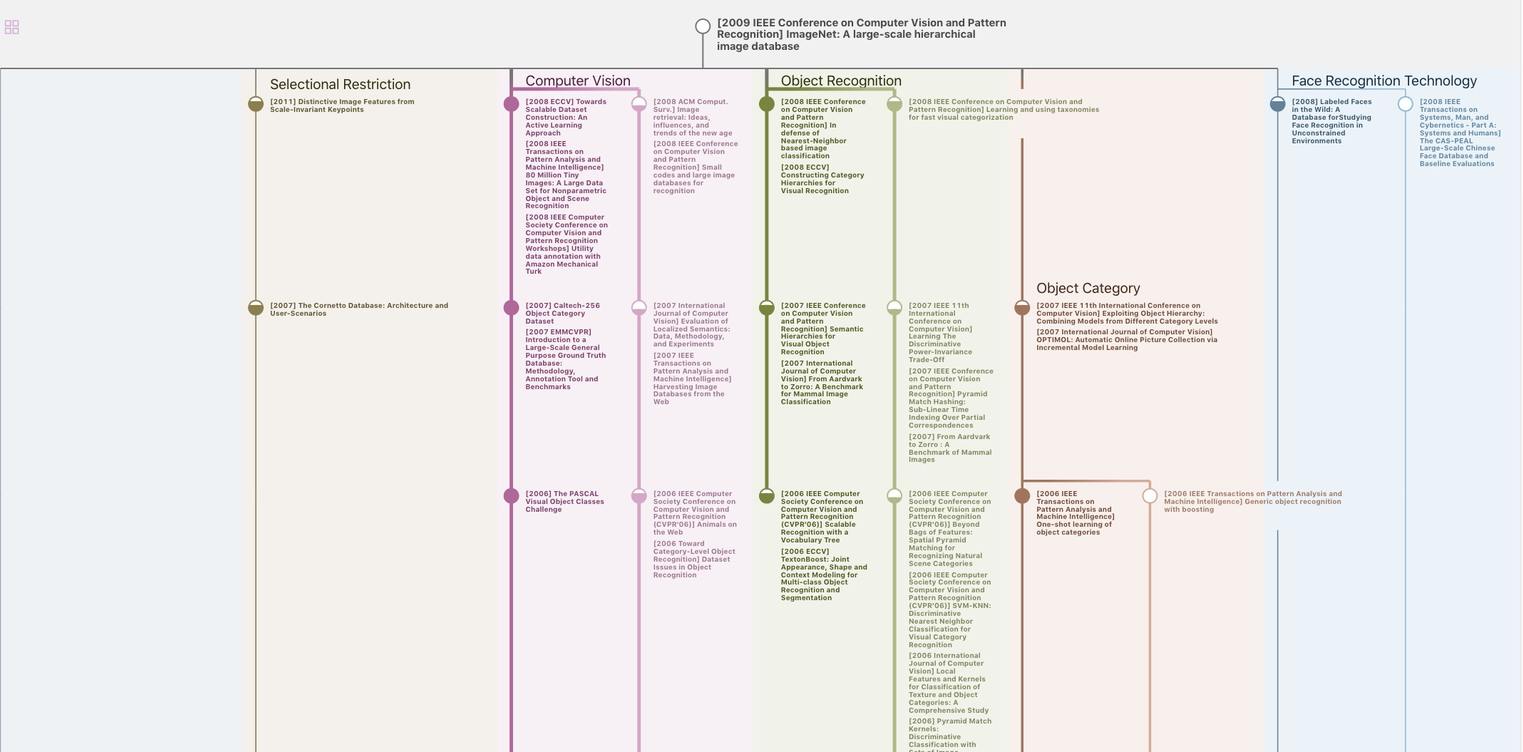
生成溯源树,研究论文发展脉络
Chat Paper
正在生成论文摘要