Curse of System Complexity and Virtue of Operational Invariants: Machine Learning based System Modeling and Attack Detection in CPS
2022 IEEE Conference on Dependable and Secure Computing (DSC)(2022)
摘要
Cyber Physical Systems (CPS) security has gained a lot of interest in recent years. Different approaches have been proposed to tackle the security challenges. Intrusion detection has been of most interest so far, involving design-based and data-based approaches. Design-based approaches require domain expertise and are not scalable, on the other hand, data-based approaches suffer from the lack of real-world datasets available for specific critical physical processes. In this work, a data collection effort is made on a realistic Water Distribution (WADI) test-bed. Collected data consists of both the normal operation as well as a range of attack scenarios. Next, machine learning-based system-modeling techniques are considered using the data from WADI. It is shown that the accuracy of system model-based intrusion detectors depends on the model accuracy and for non-linear processes, it is non-trivial to obtain accurate system models. Moreover, an operational invariants-based attack detection technique is proposed using the system design parameters. It is shown that using a simple rule-based anomaly detector performs better than the complex black-box data-based techniques.
更多查看译文
关键词
data collection effort,machine learning-based system-modeling techniques,system model-based intrusion detectors,operational invariants-based attack detection technique,system design parameters,simple rule-based anomaly detector,black-box data-based techniques,system complexity,CPS,cyber physical systems security,security challenges,intrusion detection,data-based approaches,design-based approaches,realistic water distribution test-bed
AI 理解论文
溯源树
样例
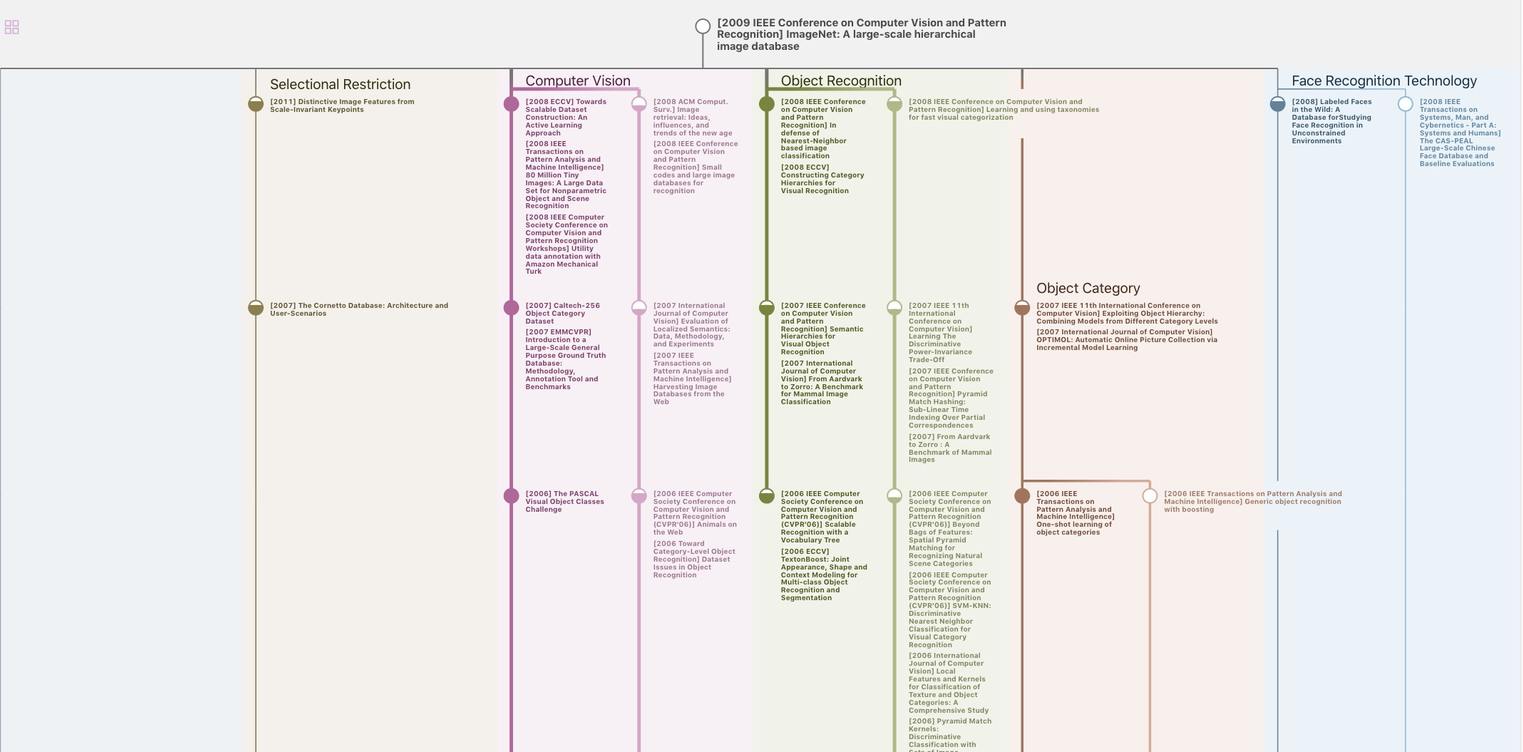
生成溯源树,研究论文发展脉络
Chat Paper
正在生成论文摘要