Multi-task Learning Model based on Multiple Characteristics and Multiple Interests for CTR prediction
2022 IEEE Conference on Dependable and Secure Computing (DSC)(2022)
摘要
In the era of big data, the acquisition and utilization of information becomes difficult with the skyrocketing amount of data. It is often difficult for ordinary users to find the in-formation or items they need, and personalized recommendation systems can solve this problem well. Currently, recommendation systems increasingly adopt models based on deep learning. The most critical issue in using deep learning for recommendation systems is how to use neural networks to accurately learn user representation vectors and item representation vectors. Many deep learning models used a single vector to represent users, but users' interests were often diverse. Therefore, some researchers consider using multiple vectors to represent user interests, and each interest vector corresponds to a category of items. This method sounds more scientific. However, these models still have problems. Their interpretation of user interests stays at the item level, and does not go deep into the item feature level. In order to solve this problem, we consider user interests from the perspective of item characteristics, and propose 3M (Multi-task, Multi-interest, Multi-feature) model. The 3M model trains multiple interest vectors for each user and extracts multiple characteristic vectors for each item at the same time, then uses a multi-task learning model to connect the characteristic vectors with the interest vectors and train them to obtain multiple interest scores. According to the multiple interest scores, the user click probability can be obtained. Experiments show that our model performs significantly better than the classic CTR(Click - Through Rate) prediction model on the experimental dataset.
更多查看译文
关键词
Recommendation algorithm,CTR prediction,multi-interest,multi-task learning
AI 理解论文
溯源树
样例
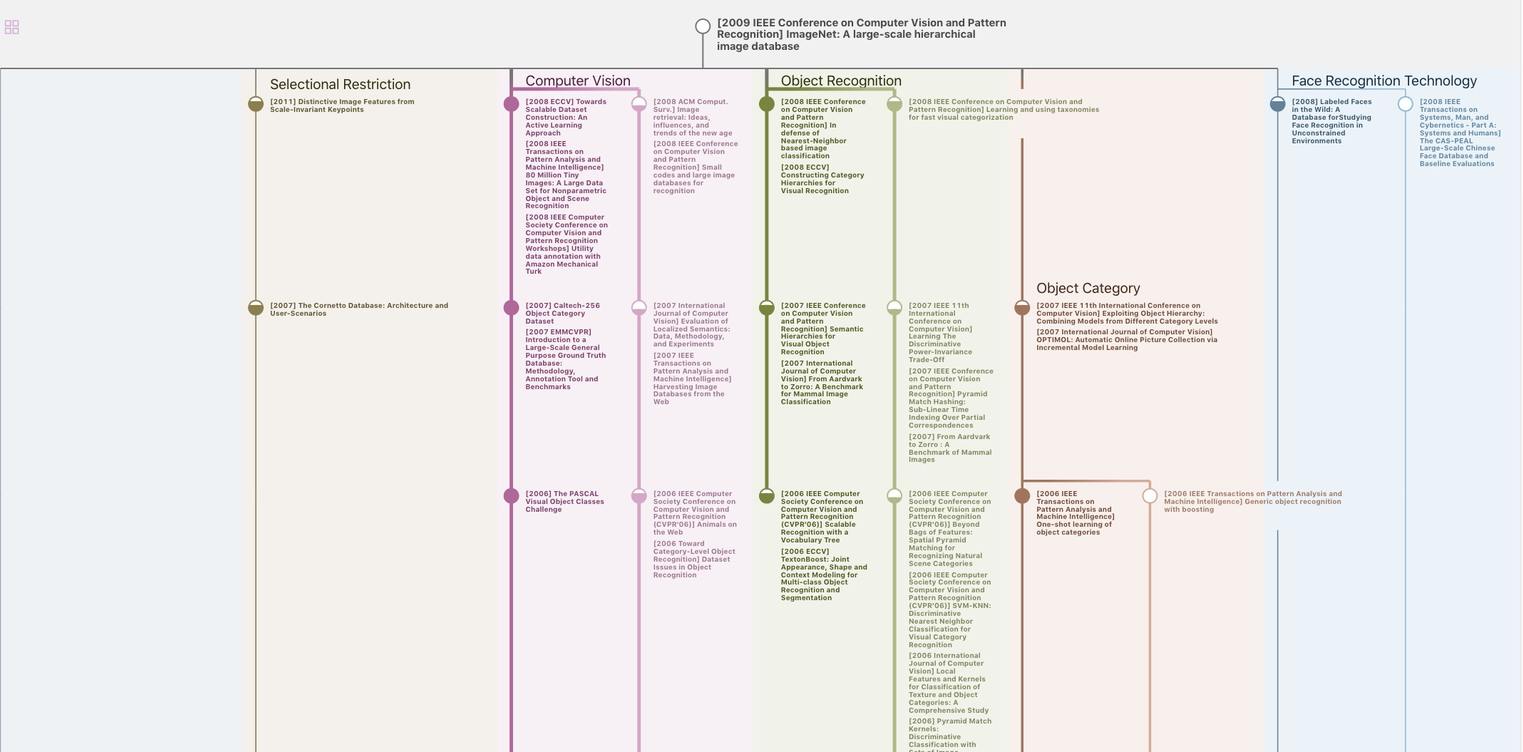
生成溯源树,研究论文发展脉络
Chat Paper
正在生成论文摘要