Physically Consistent Neural ODEs for Learning Multi-Physics Systems
IFAC PAPERSONLINE(2023)
摘要
Despite the immense success of neural networks in modeling system dynamics from data, they often remain physics-agnostic black boxes. In the particular case of physical systems, they might consequently make physically inconsistent predictions, which makes them unreliable in practice. In this paper, we leverage the framework of Irreversible port-Hamiltonian Systems (IPHS), which can describe most multi-physics systems, and rely on Neural Ordinary Differential Equations (NODEs) to learn their parameters from data. Since IPHS models are consistent with the first and second principles of thermodynamics by design, so are the proposed Physically Consistent NODEs (PC-NODEs). Furthermore, the NODE training procedure allows us to seamlessly incorporate prior knowledge of the system properties in the learned dynamics. We demonstrate the effectiveness of the proposed method by learning the thermodynamics of a building from the real-world measurements and the dynamics of a simulated gas-piston system. Thanks to the modularity and flexibility of the IPHS framework, PC-NODEs can be extended to learn physically consistent models of multi-physics distributed systems.
更多查看译文
关键词
Machine Learning,Neural networks,Multi-physics,Thermodynamics,Data-driven Modelling,Irreversible port-Hamiltonian systems
AI 理解论文
溯源树
样例
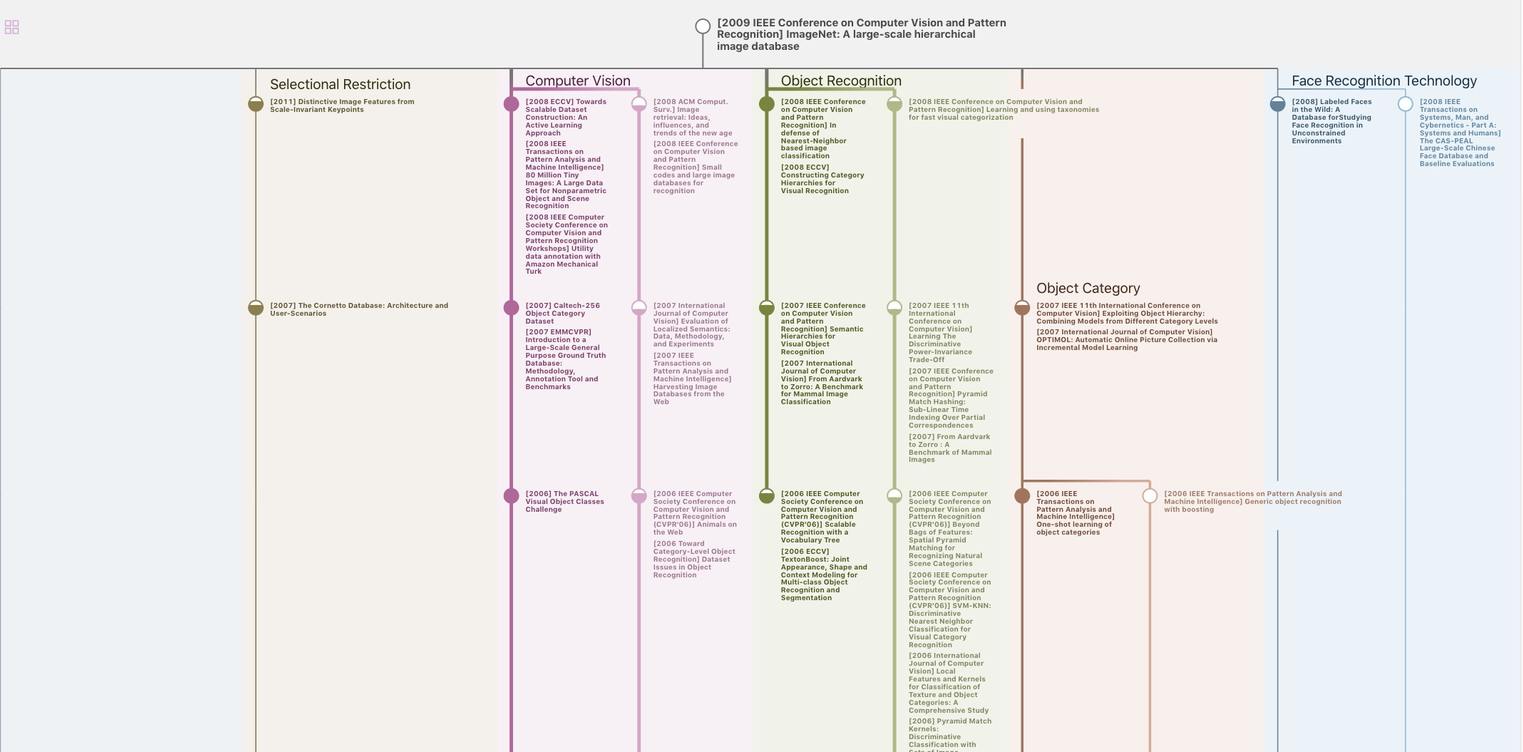
生成溯源树,研究论文发展脉络
Chat Paper
正在生成论文摘要