MixUp-MIL: Novel Data Augmentation for Multiple Instance Learning and a Study on Thyroid Cancer Diagnosis
MEDICAL IMAGE COMPUTING AND COMPUTER ASSISTED INTERVENTION, MICCAI 2023, PT VI(2023)
摘要
Multiple instance learning is a powerful approach for whole slide image-based diagnosis in the absence of pixel- or patch-level annotations. In spite of the huge size of whole slide images, the number of individual slides is often rather small, leading to a small number of labeled samples. To improve training, we propose and investigate novel data augmentation strategies for multiple instance learning based on the idea of linear and multilinear interpolation of feature vectors within and between individual whole slide images. Based on state-of-the-art multiple instance learning architectures and two thyroid cancer data sets, an exhaustive study was conducted considering a range of common data augmentation strategies. Whereas a strategy based on to the original MixUp approach showed decreases in accuracy, a novel multilinear intra-slide interpolation method led to consistent increases in accuracy.
更多查看译文
关键词
Histopathology,Data augmentation,MixUp,Multiple Instance Learning
AI 理解论文
溯源树
样例
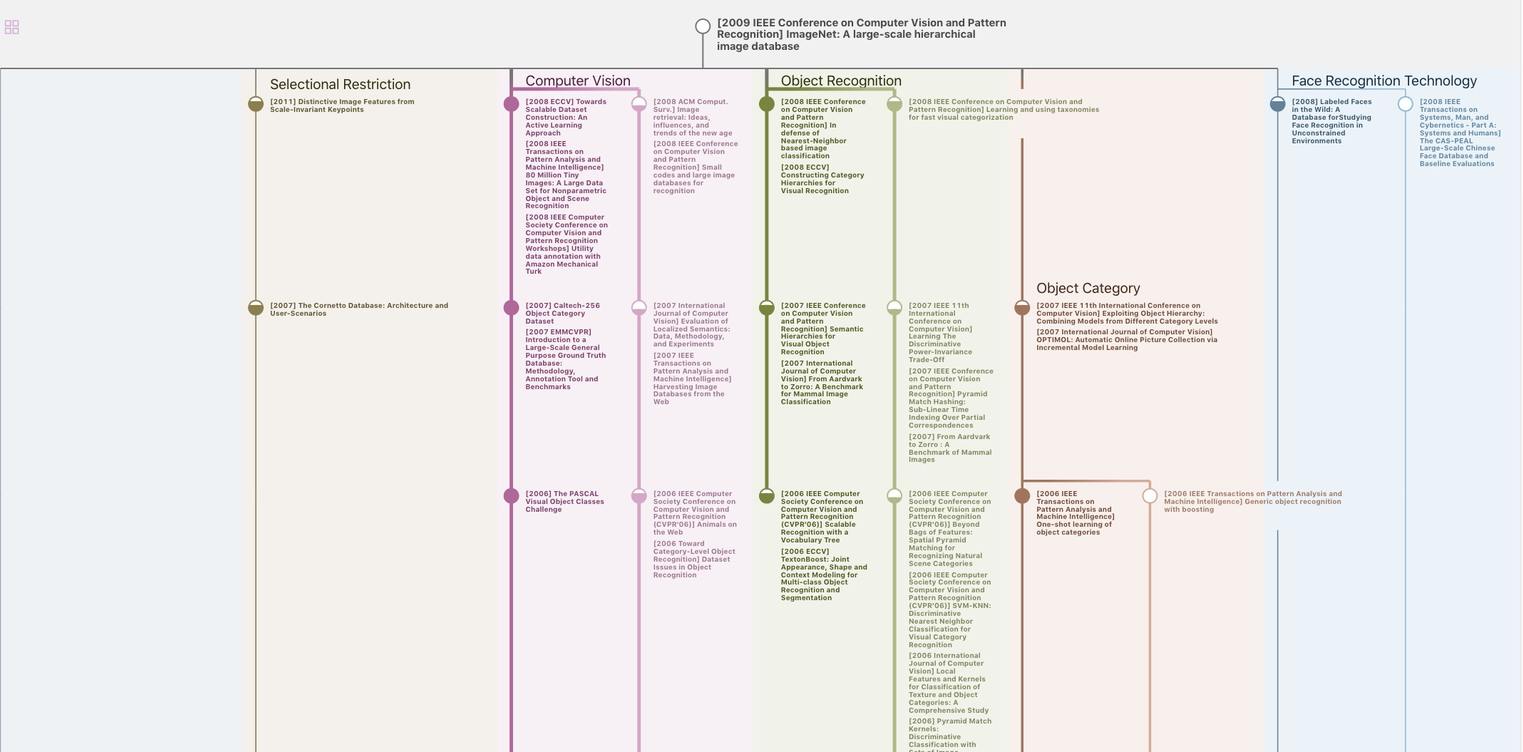
生成溯源树,研究论文发展脉络
Chat Paper
正在生成论文摘要