A class of doubly stochastic shift operators for random graph signals and their boundedness
Neural Networks(2023)
摘要
A class of doubly stochastic graph shift operators (GSO) is proposed, which is shown to exhibit: (i) lower and upper L2-boundedness for locally stationary random graph signals, (ii) L2-isometry for i.i.d. random graph signals with the asymptotic increase in the incoming neighbourhood size of vertices, and (iii) preservation of the mean of any graph signal - all prerequisites for reliable graph neural networks. These properties are obtained through a statistical consistency analysis of the proposed graph shift operator, and by exploiting the dual role of the doubly stochastic GSO as a Markov (diffusion) matrix and as an unbiased expectation operator. For generality, we consider directed graphs which exhibit asymmetric connectivity matrices. The proposed approach is validated through an example on the estimation of a vector field. (C) 2022 Elsevier Ltd. All rights reserved
更多查看译文
关键词
Graph signal processing,Doubly stochastic matrix,Shift operator,Statistical consistency,Boundedness analysis,Graph neural networks
AI 理解论文
溯源树
样例
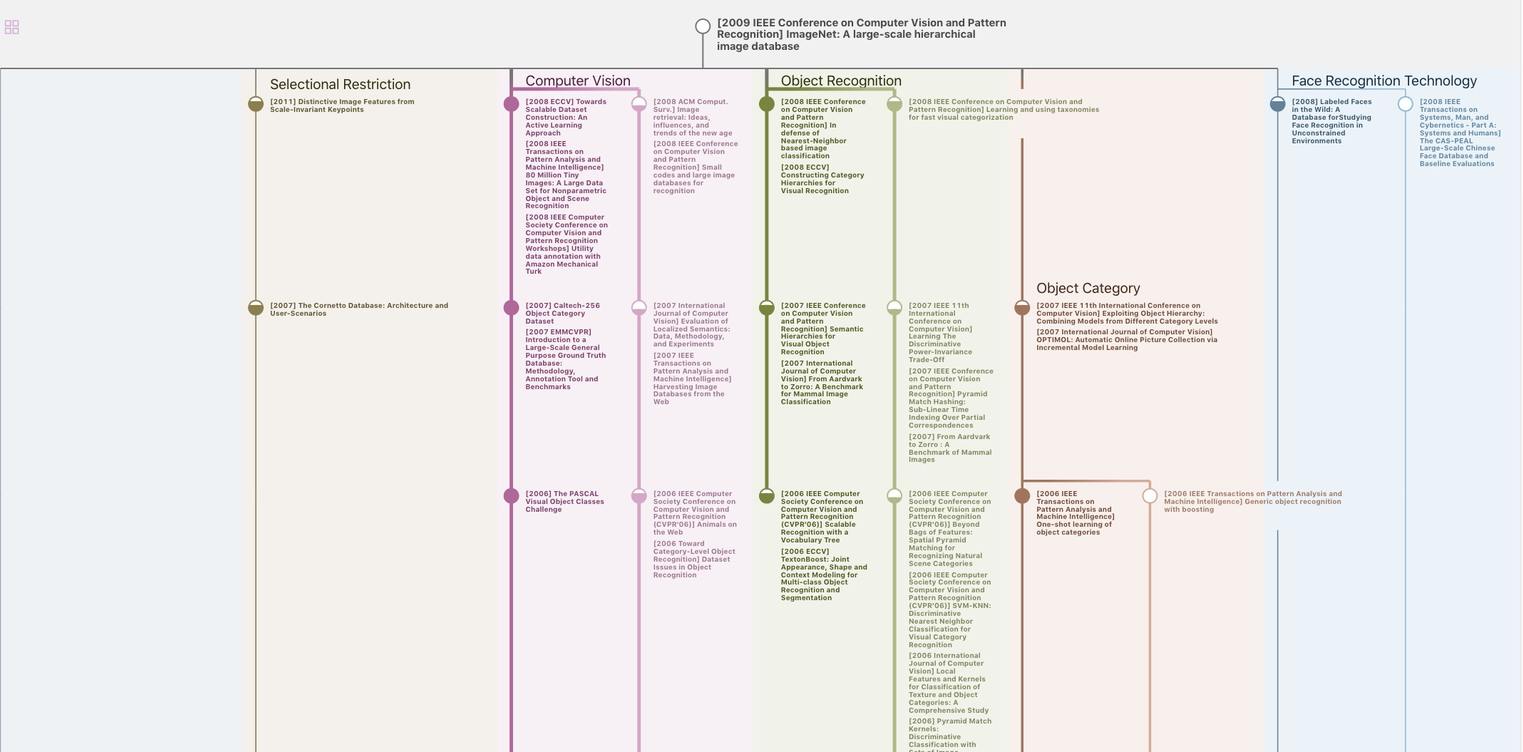
生成溯源树,研究论文发展脉络
Chat Paper
正在生成论文摘要