Fully connected neural network-based serum surface-enhanced Raman spectroscopy accurately identifies non-alcoholic steatohepatitis
Hepatology international(2022)
摘要
Background/purpose of the study There is a need to find a standardized and low-risk diagnostic tool that can non-invasively detect non-alcoholic steatohepatitis (NASH). Surface enhanced Raman spectroscopy (SERS), which is a technique combining Raman spectroscopy (RS) with nanotechnology, has recently received considerable attention due to its potential for improving medical diagnostics. We aimed to investigate combining SERS and neural network approaches, using a liver biopsy dataset to develop and validate a new diagnostic model for non-invasively identifying NASH. Methods Silver nanoparticles as the SERS-active nanostructures were mixed with blood serum to enhance the Raman scattering signals. The spectral data set was used to train the NASH classification model by a neural network primarily consisting of a fully connected residual module. Results Data on 261 Chinese individuals with biopsy-proven NAFLD were included and a prediction model for NASH was built based on SERS spectra and neural network approaches. The model yielded an AUROC of 0.83 (95% confidence interval [CI] 0.70–0.92) in the validation set, which was better than AUROCs of both serum CK-18-M30 levels (AUROC 0.63, 95% CI 0.48–0.76, p = 0.044) and the HAIR score (AUROC 0.65, 95% CI 0.51–0.77, p = 0.040). Subgroup analyses showed that the model performed well in different patient subgroups. Conclusions Fully connected neural network-based serum SERS analysis is a rapid and practical tool for the non-invasive identification of NASH. The online calculator website for the estimated risk of NASH is freely available to healthcare providers and researchers ( http://www.pan-chess.cn/calculator/RAMAN_score ).
更多查看译文
关键词
NAFLD (non-alcoholic fatty liver disease),NASH (non-alcoholic steatohepatitis),Raman,SERS (surface-enhanced Raman spectroscopy)
AI 理解论文
溯源树
样例
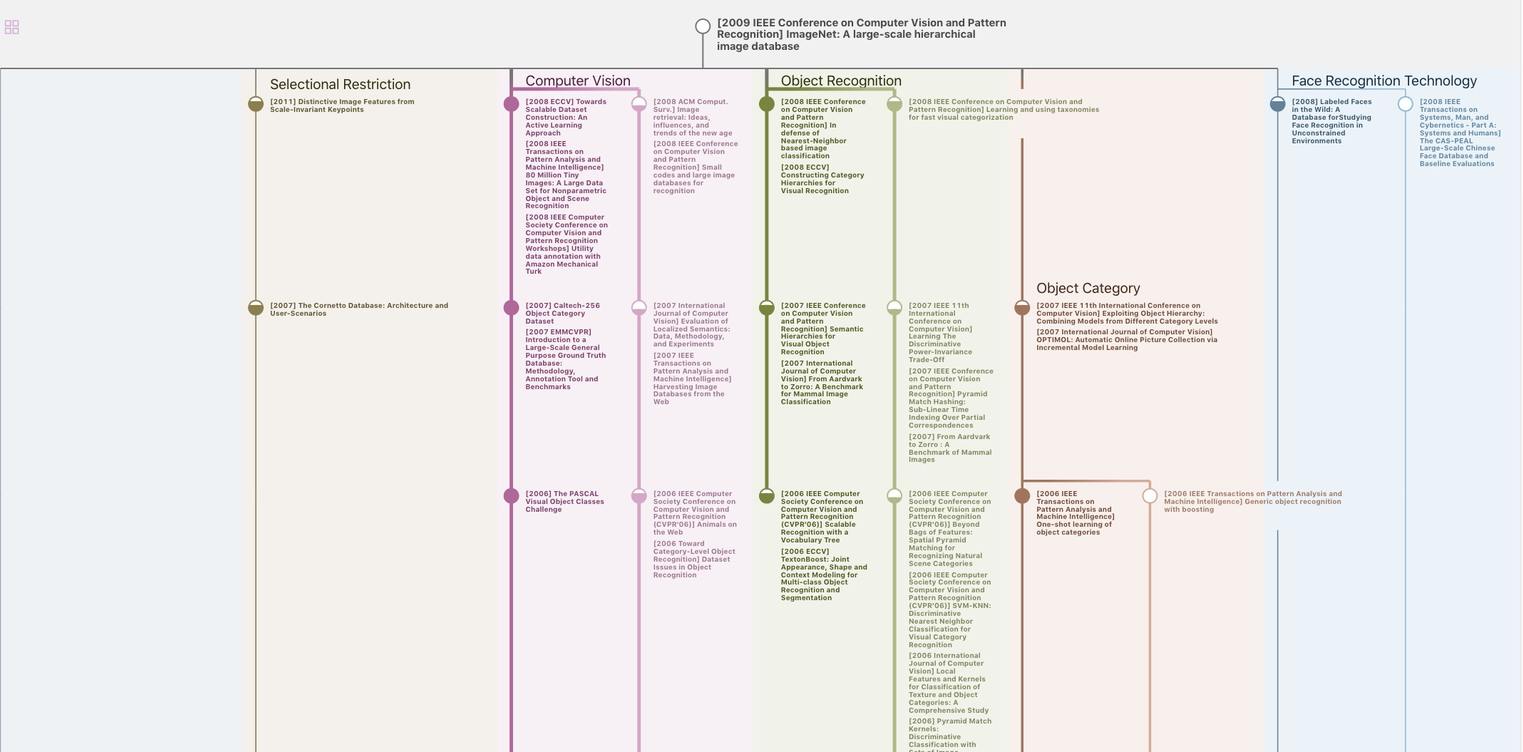
生成溯源树,研究论文发展脉络
Chat Paper
正在生成论文摘要