Grid-Based Clustering Using Boundary Detection.
Entropy(2022)
摘要
Clustering can be divided into five categories: partitioning, hierarchical, model-based, density-based, and grid-based algorithms. Among them, grid-based clustering is highly efficient in handling spatial data. However, the traditional grid-based clustering algorithms still face many problems: (1) Parameter tuning: density thresholds are difficult to adjust; (2) Data challenge: clusters with overlapping regions and varying densities are not well handled. We propose a new grid-based clustering algorithm named GCBD that can solve the above problems. Firstly, the density estimation of nodes is defined using the standard grid structure. Secondly, GCBD uses an iterative boundary detection strategy to distinguish core nodes from boundary nodes. Finally, two clustering strategies are combined to group core nodes and assign boundary nodes. Experiments on 18 datasets demonstrate that the proposed algorithm outperforms 6 grid-based competitors.
更多查看译文
关键词
boundary detection,density-based clustering,grid-based clustering
AI 理解论文
溯源树
样例
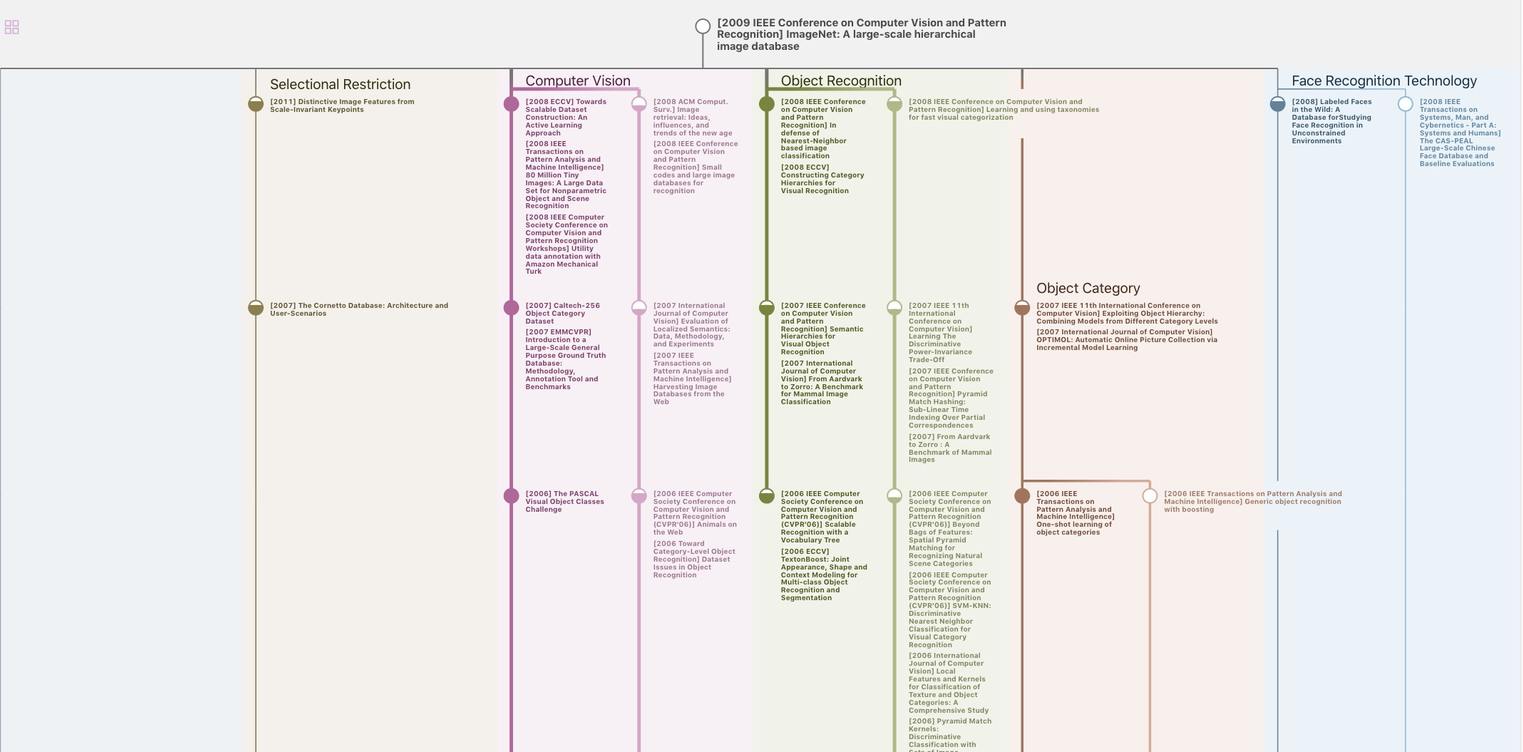
生成溯源树,研究论文发展脉络
Chat Paper
正在生成论文摘要