Coupling Convolution, Transformer and Graph Embedding for Motor Imagery Brain-Computer Interfaces
2022 IEEE International Symposium on Circuits and Systems (ISCAS)(2022)
摘要
Over the past ten years, convolution neural network (CNN) and self-attention based models (e.g., transformer) have shown extremely competitive performance in the classification of motor imagery (MI) tasks based on electroencephalogram (EEG) signals. CNN exploits local features effectively, while self-attention based models are good at capturing long-distance feature dependencies. In this paper, we propose a hybrid network structure, termed TransEEG, that takes advantage of convolutional operations and self-attention mechanisms to model both local and global dependencies for EEG signal processing. Specifically, EEG channel relationships are exploited to build a graph embedding that further improves signal classification accuracy. We evaluated the performance of TransEEG on two datasets performed MI movements. Experiments have shown that the TransEEG significantly outperformed the previous MI classification methods and achieved state-of-the-art accuracy in subject-specifical scenario.
更多查看译文
关键词
convolutional neural network (CNN),electroencephalogram (EEG),graph embedding,self-attention,transformer
AI 理解论文
溯源树
样例
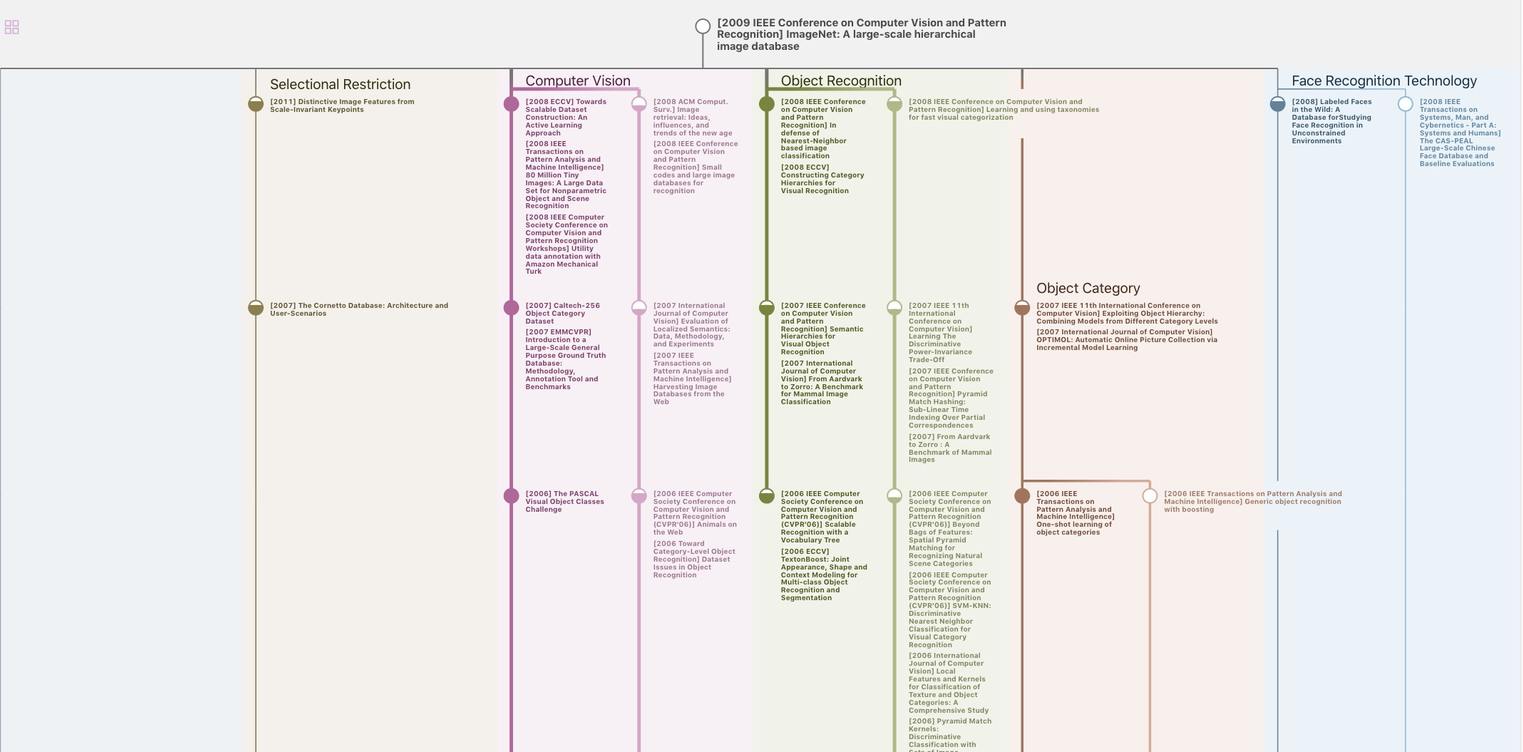
生成溯源树,研究论文发展脉络
Chat Paper
正在生成论文摘要