Dynamic Network Slicing using Deep Reinforcement Learning
2021 IEEE International Conference on Advanced Networks and Telecommunications Systems (ANTS)(2021)
摘要
5G network slicing provides service flexibility and enables the operator to optimize network resources and provide differentiated services at scale. Slicing in real-time is a challenge due to a large number of connected devices with wide-ranging quality of service requirements. A dynamic slicing method is needed to provide the operator the flexibility to alter the number of slices and change the resource allocation in RAN for each slice. We developed a fast and efficient deep reinforcement learning model to do dynamic network slicing that optimizes the service quality in real-time. Our solution is able to cater to a large number of users with different service requirements due to the use of neural networks to carry out the state-action mapping. Our model uses prioritized experience replay to reduce the training time which will allow the operator to update the model at frequent intervals. To prove the feasibility of the proposed model, we trained the learning agent using our model to carry out the network slicing task in VRAN. Our simulations show that the model learns the characteristics of the network slices and our novel reward mechanism enables the base station to make intelligent decisions to maximize network utility.
更多查看译文
关键词
Network Slicing,Network Function Virtualization,Software Defined Networks,Reinforcement Learning
AI 理解论文
溯源树
样例
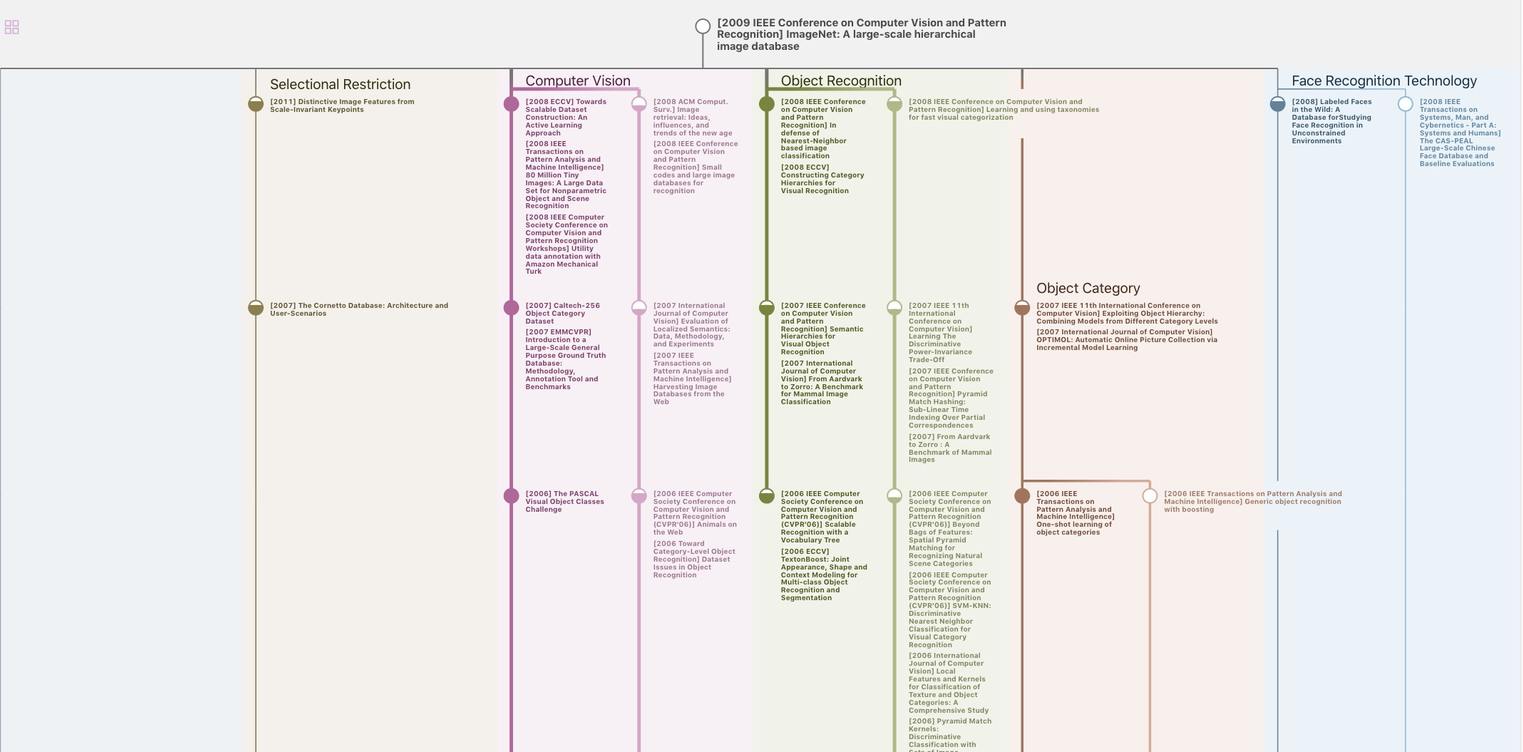
生成溯源树,研究论文发展脉络
Chat Paper
正在生成论文摘要