Achieving small-batch accuracy with large-batch scalability via Hessian-aware learning rate adjustment
NEURAL NETWORKS(2023)
摘要
We consider synchronous data-parallel neural network training with a fixed large batch size. While the large batch size provides a high degree of parallelism, it degrades the generalization performance due to the low gradient noise scale. We propose a general learning rate adjustment framework and three critical heuristics that tackle the poor generalization issue. The key idea is to adjust the learning rate based on geometric information of loss landscape and encourage the model to converge into a flat minimum that is known to better generalize to the unknown data. Our empirical study demonstrates that the Hessian-aware learning rate schedule remarkably improves the generalization performance in large-batch training. For CIFAR-10 classification with ResNet20, our method achieves 92.31% accuracy using 16,384 batch size, which is close to 92.83% achieved using 128 batch size, at a negligible extra computational cost. (c) 2022 Elsevier Ltd. All rights reserved.
更多查看译文
关键词
Deep learning,Large -batch training,Hessian information,Learning rate adjustment
AI 理解论文
溯源树
样例
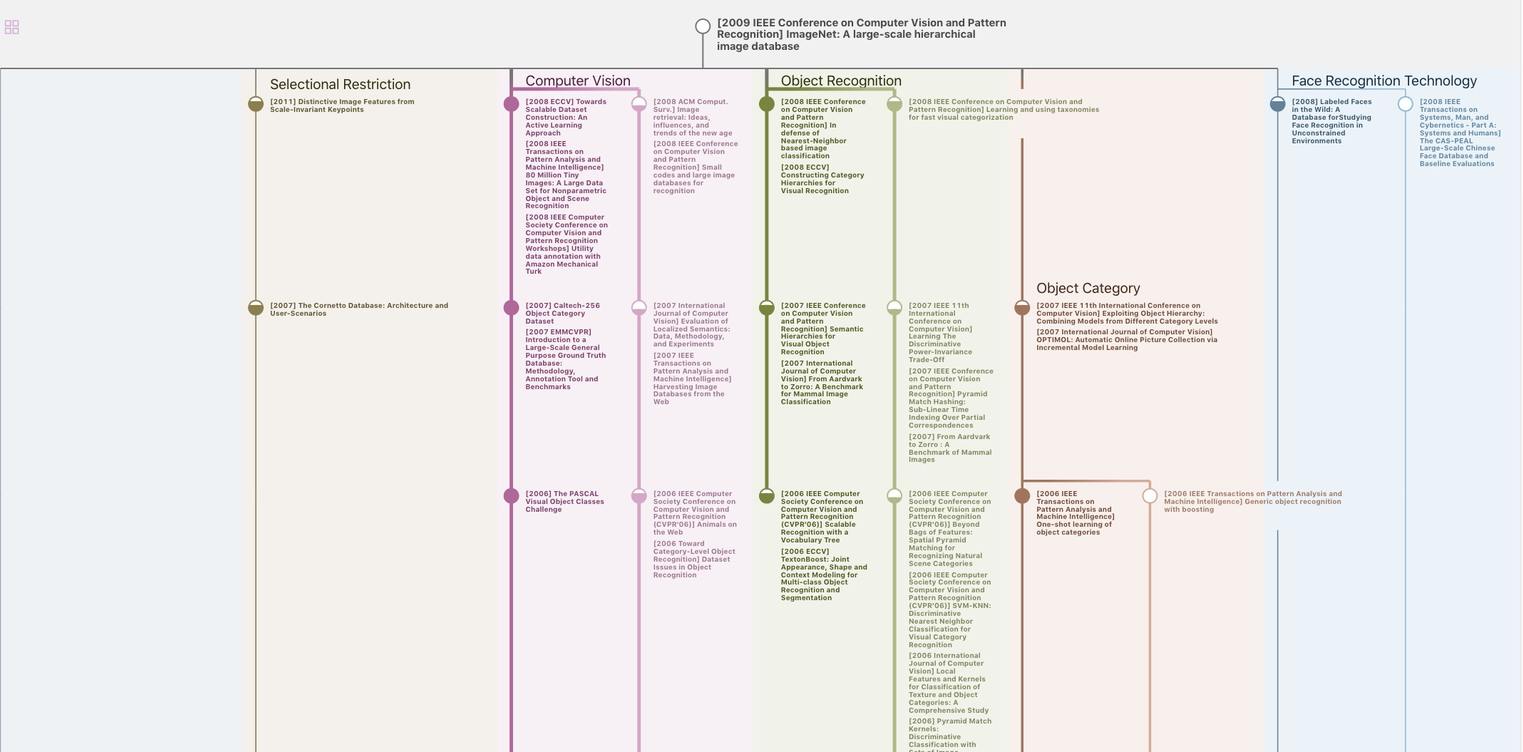
生成溯源树,研究论文发展脉络
Chat Paper
正在生成论文摘要