Continual Learning-Based Probabilistic Slow Feature Analysis for Monitoring Multimode Nonstationary Processes
IEEE TRANSACTIONS ON AUTOMATION SCIENCE AND ENGINEERING(2024)
摘要
A novel continual learning-based probabilistic slow feature analysis algorithm is introduced for monitoring multimode nonstationary processes. Multimode slow features are extracted and an elastic weight consolidation (EWC) is adopted for sequential modes. EWC was originally introduced in the setting of machine learning of sequential multi-tasks with the aim of avoiding catastrophic forgetting issue, which equally poses as a major challenge in multimode nonstationary process monitoring. When a new mode arrives, a small set of data are collected for continual learning by the proposed algorithm. A regularization term is introduced to prevent new data from significantly interfering with the learned knowledge, where the parameter importance measures are estimated. The proposed method is referred to as PSFA-EWC, which is updated continually and is capable of achieving excellent performance. PSFA-EWC furnishes backward and forward transfer ability by a single model. The significant features of previous modes are retained while consolidating new information, which may contribute to learning new relevant modes. The effectiveness of the proposed method is demonstrated via a continuous stirred tank heater and a practical coal pulverizing system.
更多查看译文
关键词
Multimode nonstationary processes,probabilistic slow feature analysis (PSFA),elastic weight consolidation (EWC),continual learning ability
AI 理解论文
溯源树
样例
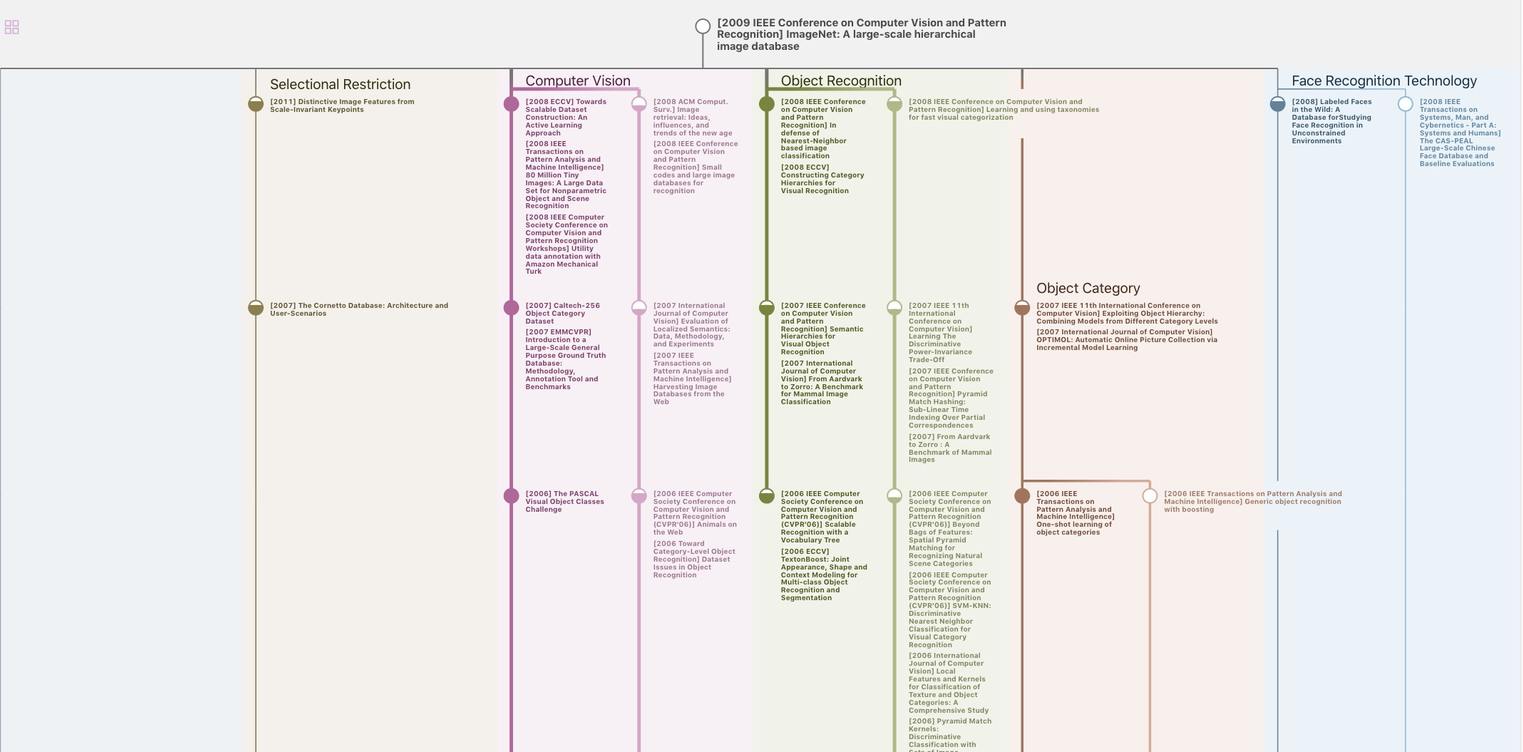
生成溯源树,研究论文发展脉络
Chat Paper
正在生成论文摘要