On the Privacy Risks of Algorithmic Recourse
arxiv(2022)
摘要
As predictive models are increasingly being employed to make consequential decisions, there is a growing emphasis on developing techniques that can provide algorithmic recourse to affected individuals. While such recourses can be immensely beneficial to affected individuals, potential adversaries could also exploit these recourses to compromise privacy. In this work, we make the first attempt at investigating if and how an adversary can leverage recourses to infer private information about the underlying model's training data. To this end, we propose a series of novel membership inference attacks which leverage algorithmic recourse. More specifically, we extend the prior literature on membership inference attacks to the recourse setting by leveraging the distances between data instances and their corresponding counterfactuals output by state-of-the-art recourse methods. Extensive experimentation with real world and synthetic datasets demonstrates significant privacy leakage through recourses. Our work establishes unintended privacy leakage as an important risk in the widespread adoption of recourse methods.
更多查看译文
关键词
privacy risks
AI 理解论文
溯源树
样例
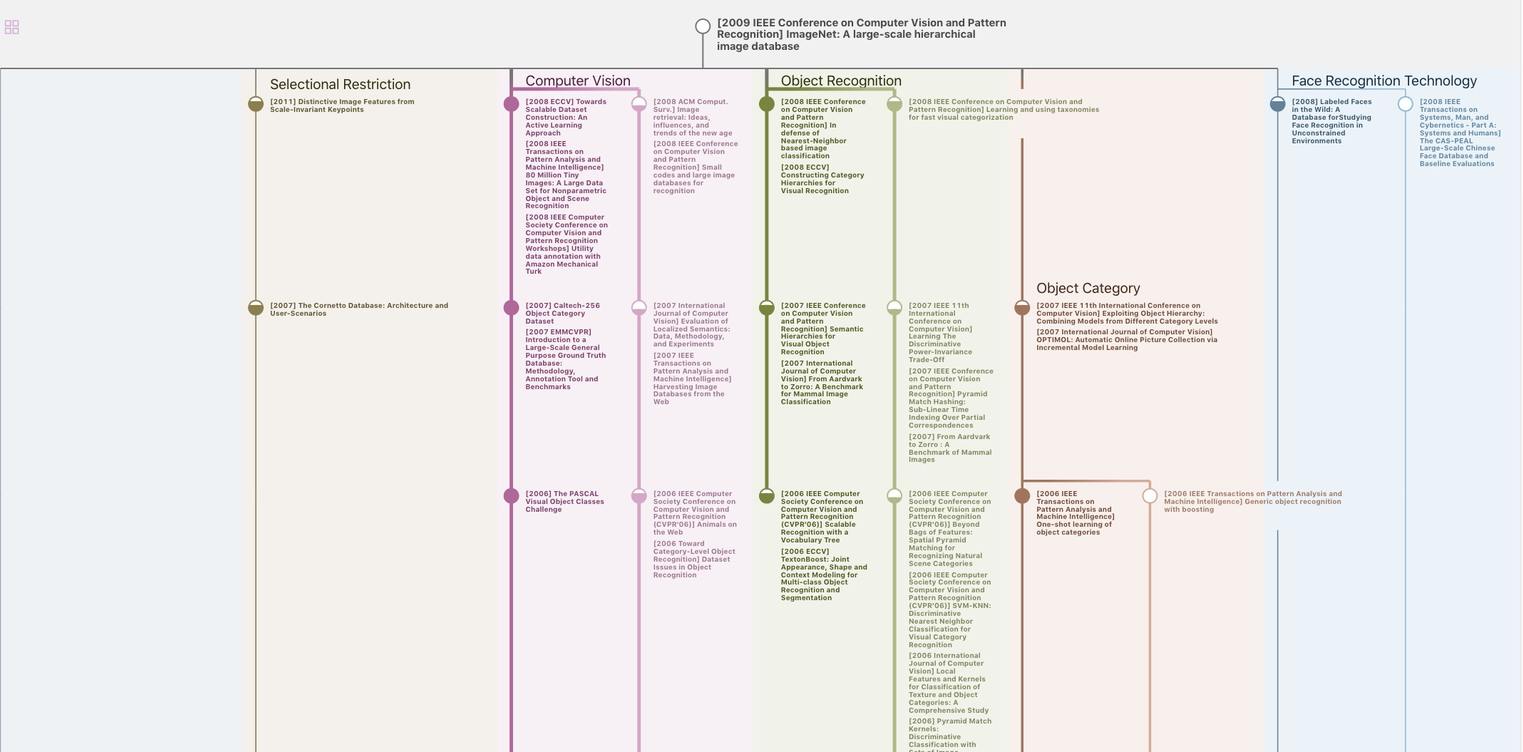
生成溯源树,研究论文发展脉络
Chat Paper
正在生成论文摘要