Harmonizing Output Imbalance for semantic segmentation on extremely-imbalanced input data
arxiv(2022)
摘要
Semantic segmentation is a high level computer vision task that assigns a label for each pixel of an image. It is challenging to deal with extremely-imbalanced data in which the ratio of target pixels to background pixels is lower than 1:1000. Such severe input imbalance leads to output imbalance for poor model training. This paper considers three issues for extremely-imbalanced data: inspired by the region-based Dice loss, an implicit measure for the output imbalance is proposed, and an adaptive algorithm is designed for guiding the output imbalance hyperparameter selection; then it is generalized to distribution-based loss for dealing with output imbalance; and finally a compound loss with our adaptive hyperparameter selection algorithm can keep the consistency of training and inference for harmonizing the output imbalance. With four popular deep architectures on our private dataset from three different input imbalance scales and three public datasets, extensive experiments demonstrate the competitive/promising performance of the proposed method.
更多查看译文
AI 理解论文
溯源树
样例
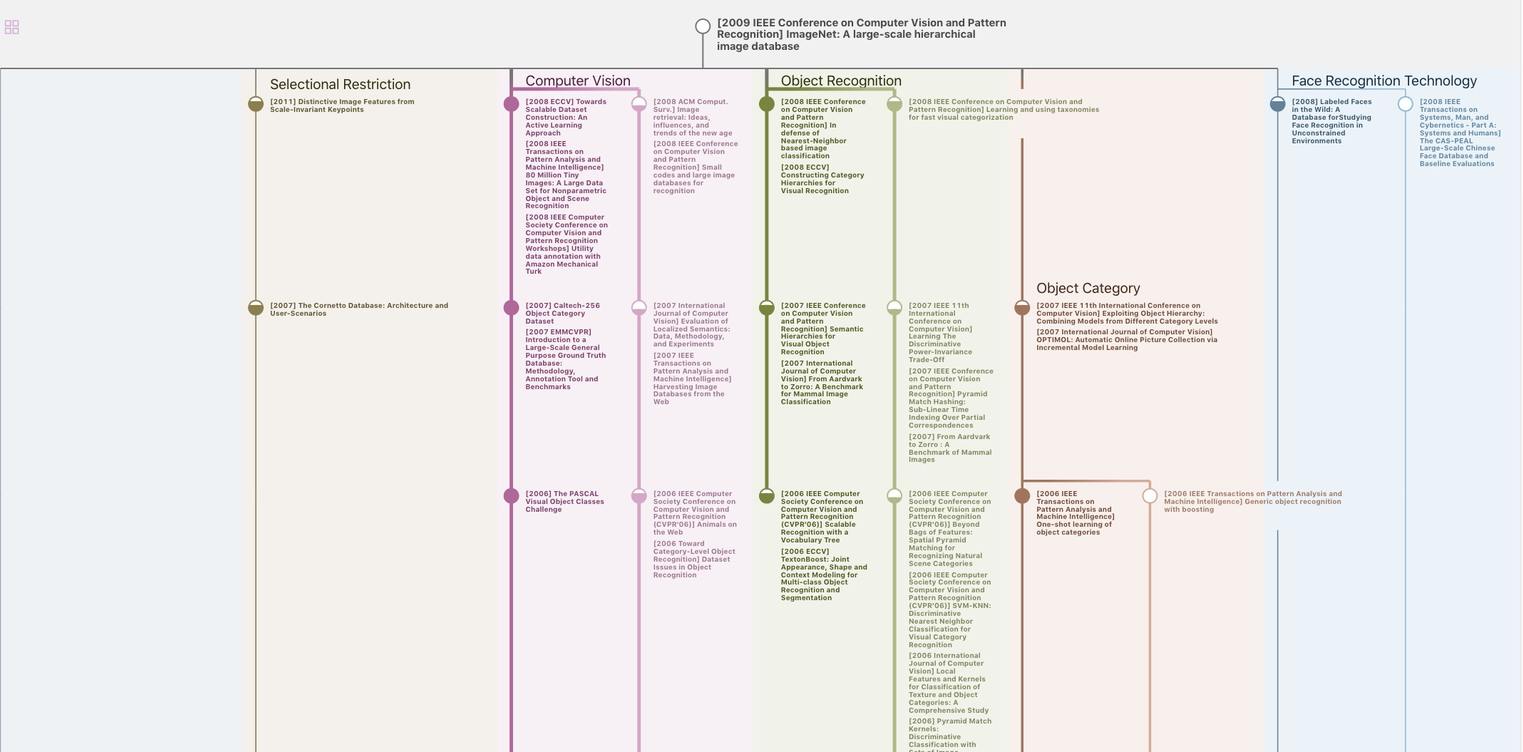
生成溯源树,研究论文发展脉络
Chat Paper
正在生成论文摘要