New patch-based strategy for COVID-19 automatic identification using chest x-ray images
HEALTH AND TECHNOLOGY(2022)
摘要
Purpose The development of a robust model for automatic identification of COVID-19 based on chest x-rays has been a widely addressed topic over the last couple of years; however, the scarcity of good quality images sets, and their limited size, have proven to be an important obstacle to obtain reliable models. In fact, models proposed so far have suffered from over-fitting erroneous features instead of learning lung features, a phenomenon known as shortcut learning. In this research, a new image classification methodology is proposed that attempts to mitigate this problem. Methods To this end, annotation by expert radiologists of a set of images was performed. The lung region was then segmented and a new classification strategy based on a patch partitioning that improves the resolution of the convolution neural network is proposed. In addition, a set of native images, used as an external evaluation set, is released. Results The best results were obtained for the 6-patch splitting variant with 0.887 accuracy, 0.85 recall and 0.848 F1score on the external validation set. Conclusion The results show that the proposed new strategy maintains similar values between internal and external validation, which gives our model generalization power, making it available for use in hospital settings.
更多查看译文
关键词
Chest X-Rays,COVID-19,Automatic classification
AI 理解论文
溯源树
样例
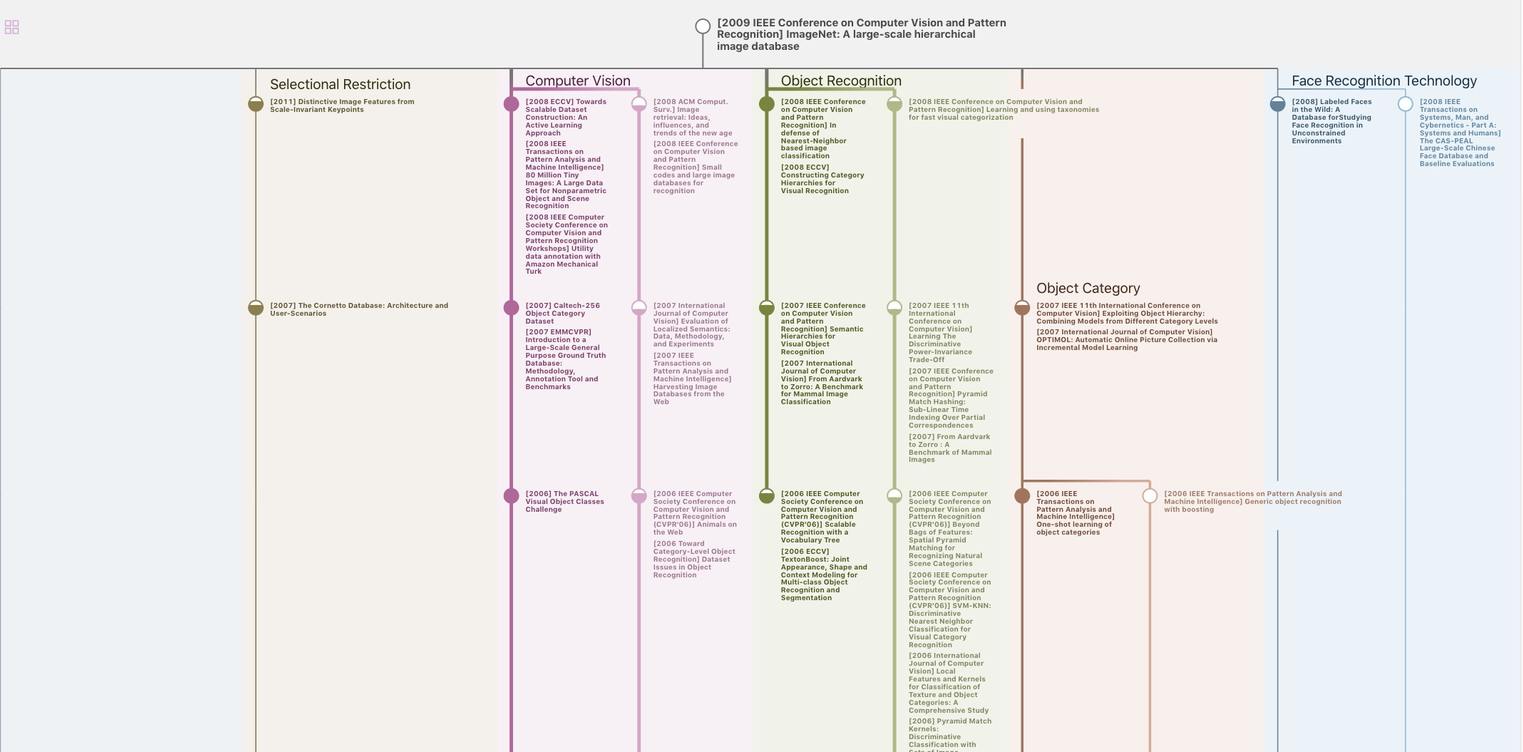
生成溯源树,研究论文发展脉络
Chat Paper
正在生成论文摘要