CT image quality evaluation in the age of deep learning: trade-off between functionality and fidelity
EUROPEAN RADIOLOGY(2022)
摘要
Objective To quantitatively compare DLIR and ASiR-V with realistic anatomical images. Methods CT scans of an anthropomorphic phantom were acquired using three routine protocols (brain, chest, and abdomen) at four dose levels, with images reconstructed at five levels of ASiR-V and three levels of DLIR. Noise power spectrum (NPS) was estimated using a difference image by subtracting two matching images from repeated scans. Using the max-dose FBP reconstruction as the ground truth, the structure similarity index (SSIM) and gradient magnitude (GM) of difference images were evaluated. Image noise magnitude (σ), frequency location of the NPS peak (f peak ), mean SSIM (MSSIM), and mean GM (MGM) were used as quantitative metrics to compare image quality, for each anatomical region, protocol, algorithm, dose level, and slice thickness. Results Image noise had a strong ( R 2 > 0.99) power law relationship with dose for all algorithms. For the abdomen and chest, f peak shifted from 0.3 (FBP) down to 0.15 mm -1 (ASiR-V 100%) with increasing ASiR-V strength but remained 0.3 mm -1 for all DLIR levels. f peak shifted down for the brain protocol with increasing DLIR levels. Three levels of DLIR produced similar image noise levels as ASiR-V 40%, 80%, and 100%, respectively. DLIR had lower MSSIM but higher MGM than ASiR-V while matching imaging noise. Conclusion Compared to ASiR-V, DLIR presents trade-offs between functionality and fidelity: it has a noise texture closer to FBP and more edge enhancement, but reduced structure similarity. These trade-offs and unique protocol-dependent behaviors of DLIR should be considered during clinical implementation and deployment. Key Points • DLIR reconstructed images demonstrate closer noise texture and lower structure similarity to FBP while producing equivalent noise levels comparable to ASiR-V. • DLIR has additional edge enhancement as compared to ASiR-V. • DLIR has unique protocol-dependent behaviors that should be considered for clinical implementation.
更多查看译文
关键词
Computed tomography,X-ray,Deep learning,Algorithms
AI 理解论文
溯源树
样例
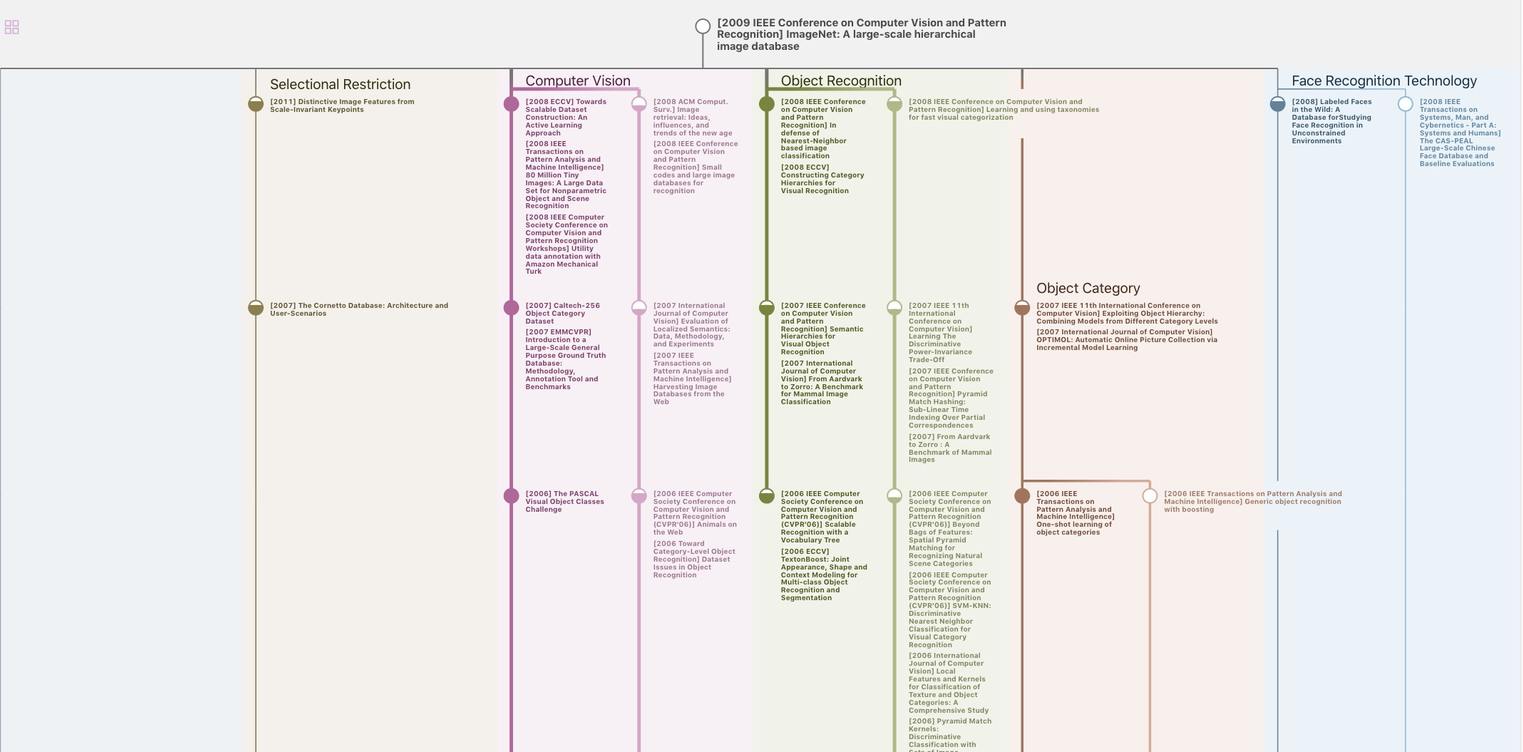
生成溯源树,研究论文发展脉络
Chat Paper
正在生成论文摘要