miCSE: Mutual Information Contrastive Learning for Low-shot Sentence Embeddings
conf_acl(2022)
摘要
This paper presents miCSE, a mutual information-based Contrastive learning framework that significantly advances the state-of-the-art in few-shot sentence embedding. The proposed approach imposes alignment between the attention pattern of different views during contrastive learning. Learning sentence embeddings with miCSE entails enforcing the syntactic consistency across augmented views for every single sentence, making contrastive self-supervised learning more sample efficient. As a result, the proposed approach shows strong performance in the few-shot learning domain. While it achieves superior results compared to state-of-the-art methods on multiple benchmarks in few-shot learning, it is comparable in the full-shot scenario. The proposed approach is conceptually simple, easy to implement and optimize, yet empirically powerful. This study opens up avenues for efficient self-supervised learning methods that are more robust than current contrastive methods for sentence embedding.
更多查看译文
关键词
mutual information contrastive learning,embeddings,low-shot
AI 理解论文
溯源树
样例
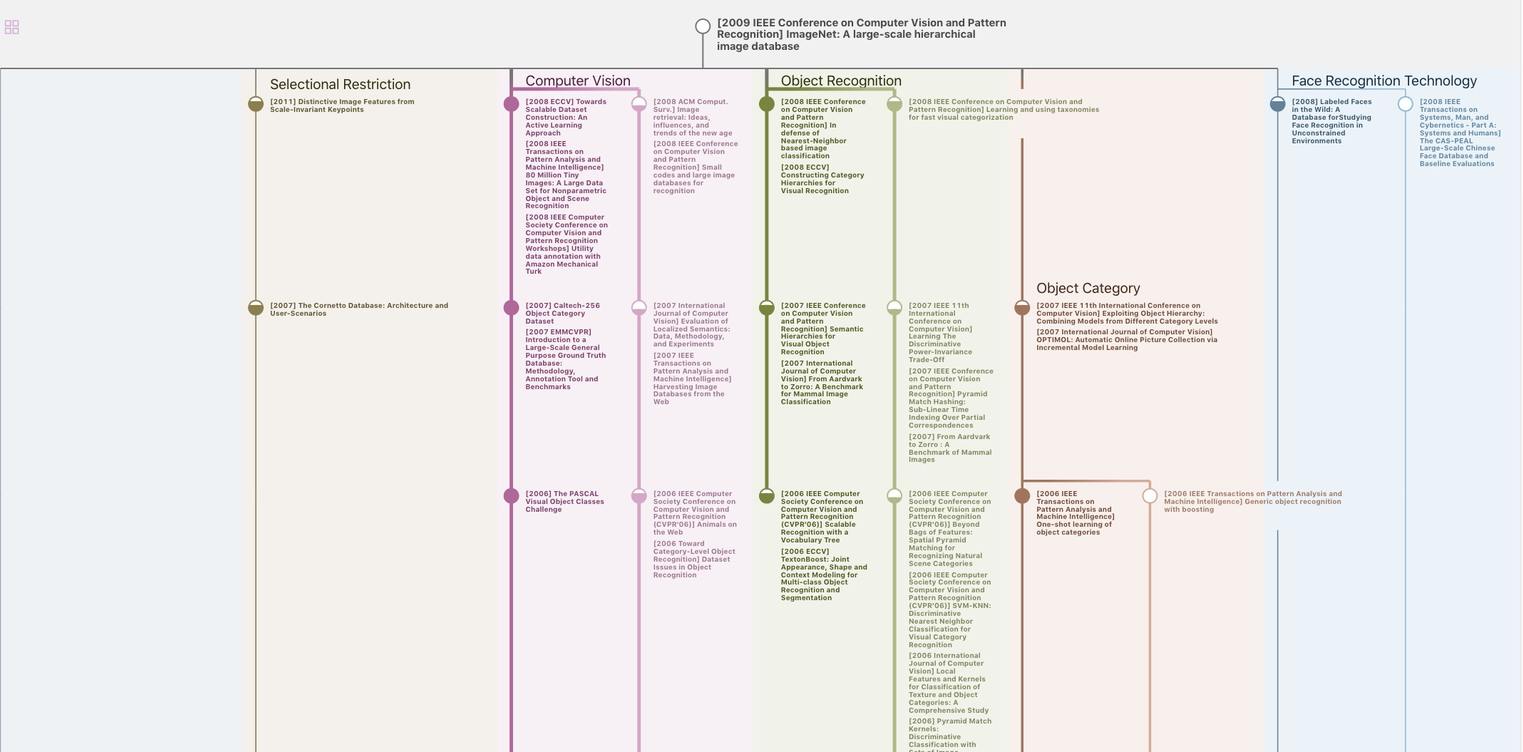
生成溯源树,研究论文发展脉络
Chat Paper
正在生成论文摘要