From Distance to Dependency: A Paradigm Shift of Full-reference Image Quality Assessment
arxiv(2022)
摘要
Deep learning-based full-reference image quality assessment (FR-IQA) models typically rely on the feature distance between the reference and distorted images. However, the underlying assumption of these models that the distance in the deep feature domain could quantify the quality degradation does not scientifically align with the invariant texture perception, especially when the images are generated artificially by neural networks. In this paper, we bring a radical shift in inferring the quality with learned features and propose the Deep Image Dependency (DID) based FR-IQA model. The feature dependency facilitates the comparisons of deep learning features in a high-order manner with Brownian distance covariance, which is characterized by the joint distribution of the features from reference and test images, as well as their marginal distributions. This enables the quantification of the feature dependency against nonlinear transformation, which is far beyond the computation of the numerical errors in the feature space. Experiments on image quality prediction, texture image similarity, and geometric invariance validate the superior performance of our proposed measure.
更多查看译文
关键词
image
AI 理解论文
溯源树
样例
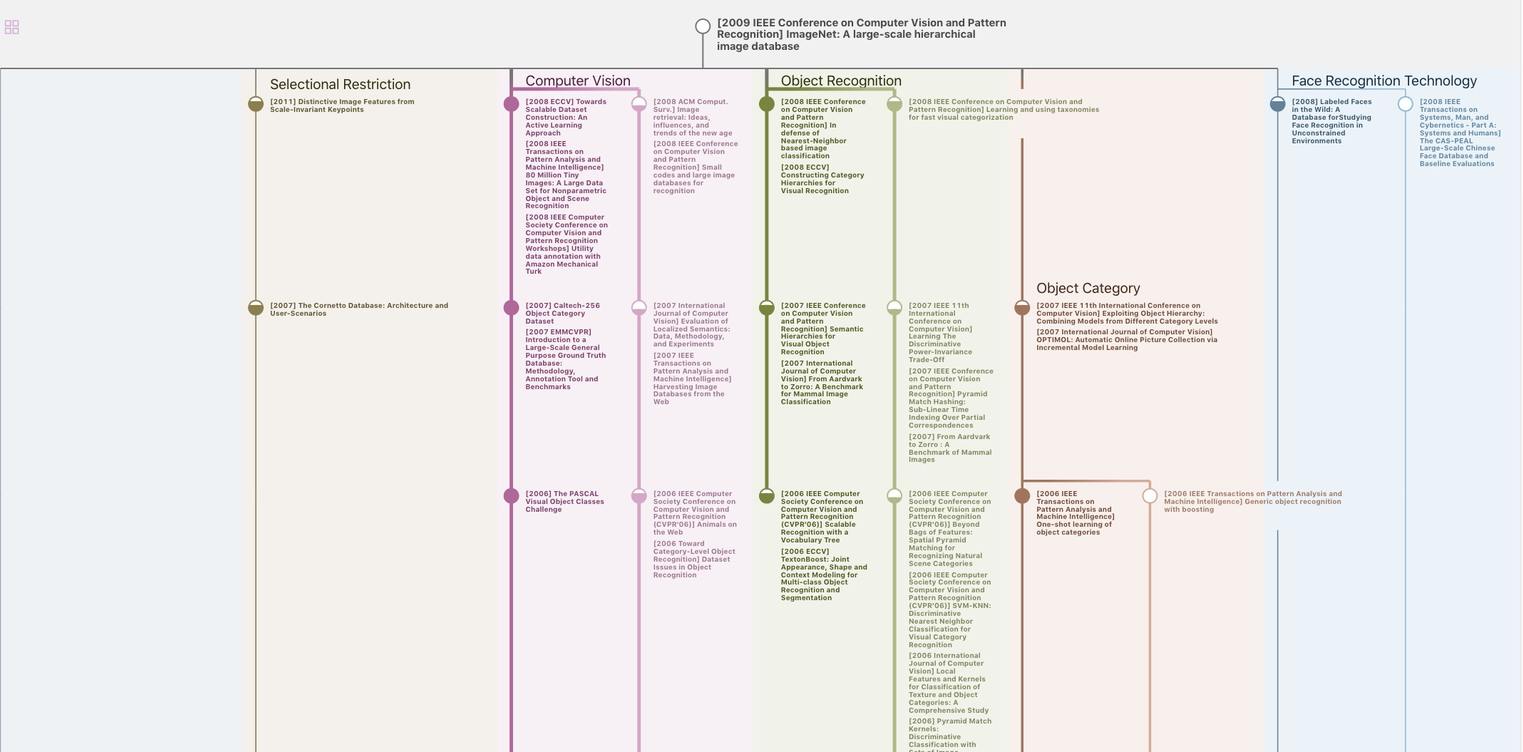
生成溯源树,研究论文发展脉络
Chat Paper
正在生成论文摘要