Approximate backwards differentiation of gradient flow
arxiv(2022)
摘要
The gradient flow (GF) is an ODE for which its explicit Euler's discretization is the gradient descent method. In this work, we investigate a family of methods derived from \emph{approximate implicit discretizations} of (\GF), drawing the connection between larger stability regions and less sensitive hyperparameter tuning. We focus on the implicit $\tau$-step backwards differentiation formulas (BDFs), approximated in an inner loop with a few iterations of vanilla gradient descent, and give their convergence rate when the objective function is convex, strongly convex, or nonconvex. Numerical experiments show the wide range of effects of these different methods on extremely poorly conditioned problems, especially those brought about in training deep neural networks.
更多查看译文
AI 理解论文
溯源树
样例
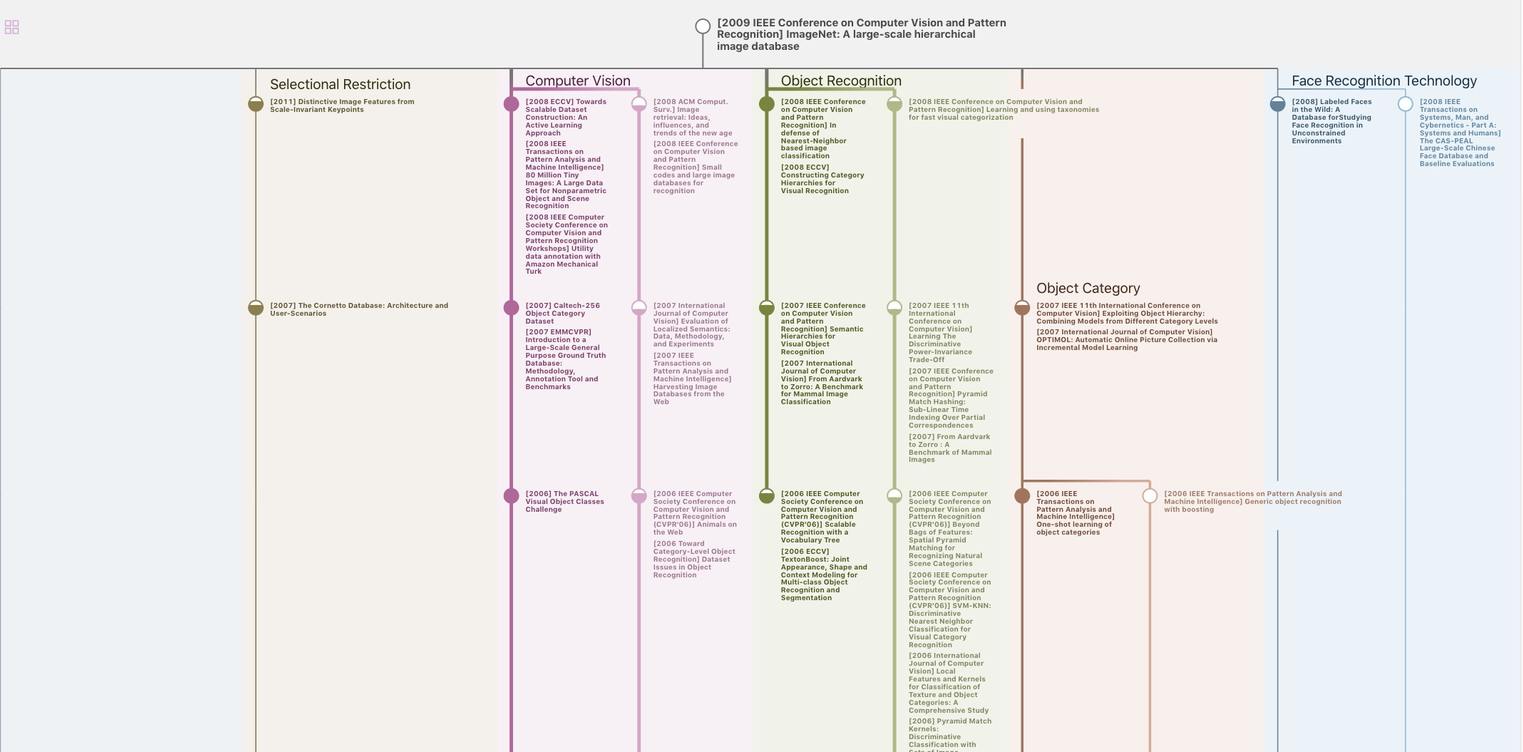
生成溯源树,研究论文发展脉络
Chat Paper
正在生成论文摘要