Attention-Based Multi-Input Multi-Output Neural Network for Plant Disease Prediction Using Multisensor System
IEEE Sensors Journal(2022)
摘要
Disease detection and prevention in plants are crucial for generating healthy crops and securing the livelihood of farmers. Leaf wetness duration (LWD), ambient temperature, and relative humidity (RH) are essential parameters that lead to the germination of fungal diseases in plants. In this work, an in-house-developed leaf wetness sensor (LWS) is used to capture LWD, and commercial temperature and humidity sensors are used to record the ambient temperature and humidity, respectively. Subsequently, these sensors are interfaced with an in-house-developed Internet of Things (IoT)-enabled electronics and deployed (three sensor nodes) in the field. We have proposed an attention-based multi-input multi-output neural network (A-MIMONN) to predict diseases using self-collected data. The data for training the model are collected from the three sensors nodes, each comprising of temperature, humidity, and LWSs. The designed network is an ensemble of various submodels, trained individually using data from different sensor nodes. The findings of these individually trained networks are then combined to give the final output. The network is designed to achieve better results by employing the attention mechanism, reinforcing the influence of the most important feature on the predicted results. The average accuracy of the model was found to be about 94%. The model displayed a high average precision of 96% and a high average recall value of 97%. The average F1 score of 97% indicated an excellent balance of precision and accuracy.
更多查看译文
关键词
Agricultural applications,attention-based,leaf wetness sensor (LWS),multi-input multi-output (MIMO),plant disease management,synthetic time-series data,time generative adversarial network (TimeGAN)
AI 理解论文
溯源树
样例
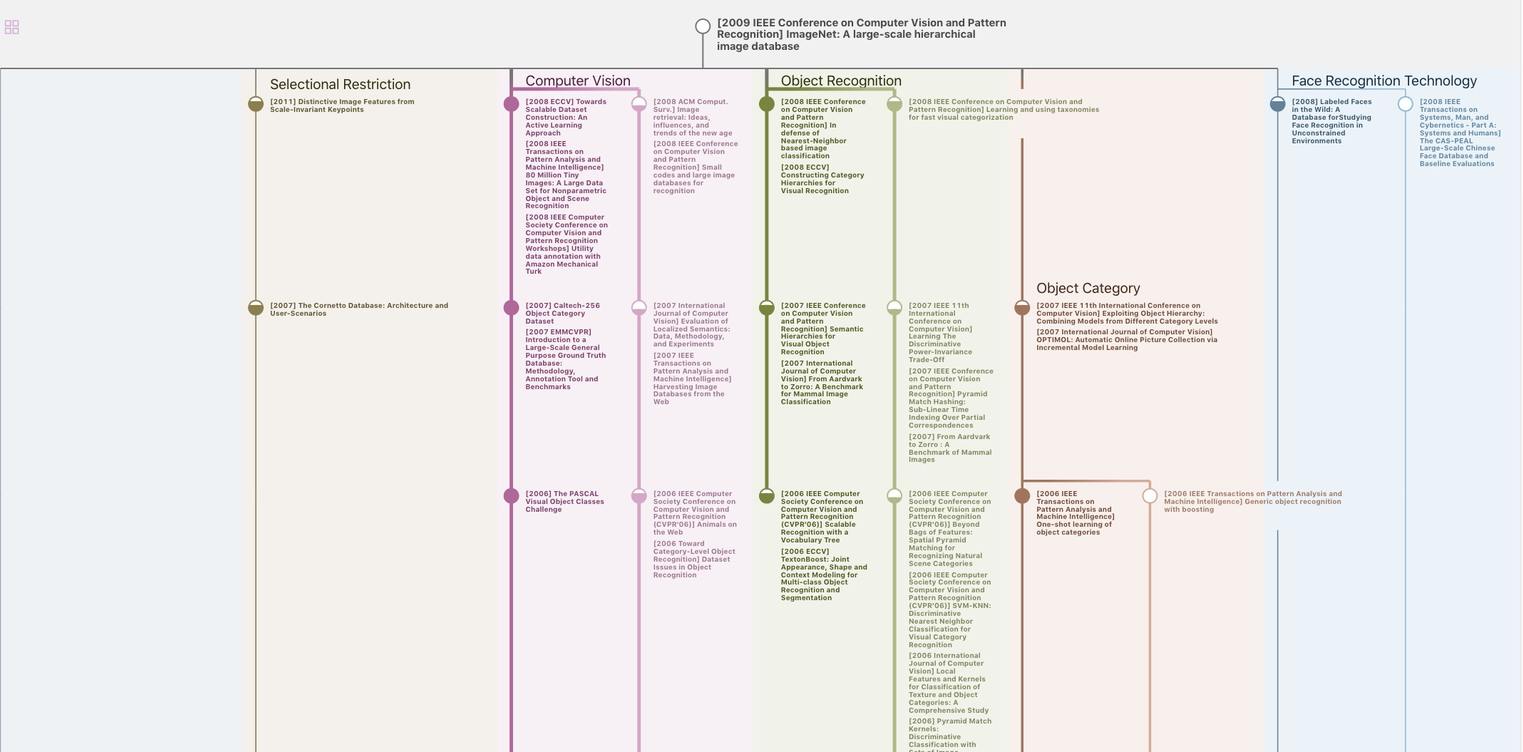
生成溯源树,研究论文发展脉络
Chat Paper
正在生成论文摘要