Quantitative Brain Morphometry of Portable Low-Field-Strength MRI Using Super-Resolution Machine Learning
Radiology(2023)
摘要
Background: Portable, low-field-strength (0.064-T) MRI has the potential to transform neuroimaging but is limited by low spatial resolution and low signal-to-noise ratio. Purpose: To implement a machine learning super-resolution algorithm that synthesizes higher spatial resolution images (1-mm isotropic) from lower resolution T1-weighted and T2-weighted portable brain MRI scans, making them amenable to automated quantitative morphometry.Materials and Methods: An external high-field-strength MRI data set (1-mm isotropic scans from the Open Access Series of Imaging Studies data set) and segmentations for 39 regions of interest (ROIs) in the brain were used to train a super-resolution convolu-tional neural network (CNN). Secondary analysis of an internal test set of 24 paired low-and high-field-strength clinical MRI scans in participants with neurologic symptoms was performed. These were part of a prospective observational study (August 2020 to December 2021) at Massachusetts General Hospital (exclusion criteria: inability to lay flat, body habitus preventing low-field -strength MRI, presence of MRI contraindications). Three well-established automated segmentation tools were applied to three sets of scans: high-field-strength (1.5-3 T, reference standard), low-field-strength (0.064 T), and synthetic high-field-strength images generated from the low-field-strength data with the CNN. Statistical significance of correlations was assessed with Student t tests. Correlation coefficients were compared with Steiger Z tests.Results: Eleven participants (mean age, 50 years +/- 14; seven men) had full cerebrum coverage in the images without motion arti-facts or large stroke lesion with distortion from mass effect. Direct segmentation of low-field-strength MRI yielded nonsignificant correlations with volumetric measurements from high field strength for most ROIs (P > .05). Correlations largely improved when segmenting the synthetic images: P values were less than .05 for all ROIs (eg, for the hippocampus [r = 0.85; P < .001], thalamus [r = 0.84; P = .001], and whole cerebrum [r = 0.92; P < .001]). Deviations from the model (z score maps) visually correlated with pathologic abnormalities.Conclusion: This work demonstrated proof-of-principle augmentation of portable MRI with a machine learning super-resolution algorithm, which yielded highly correlated brain morphometric measurements to real higher resolution images.(c) RSNA, 2022
更多查看译文
关键词
mri,brain,low-field-strength,super-resolution
AI 理解论文
溯源树
样例
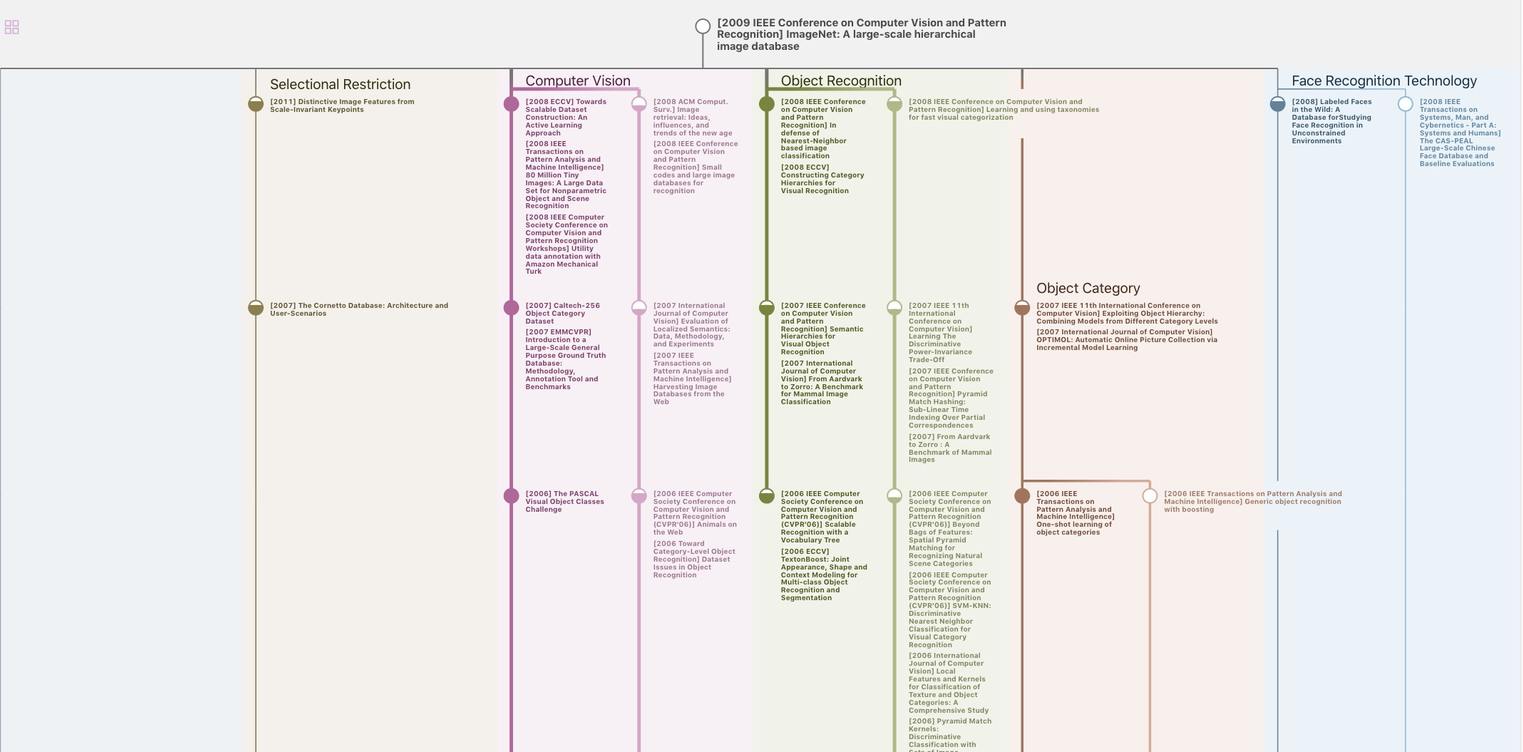
生成溯源树,研究论文发展脉络
Chat Paper
正在生成论文摘要