Stochastic Lag Time Parameterization for Markov State Models of Protein Dynamics.
The journal of physical chemistry. B(2022)
摘要
Markov state models (MSMs) play a key role in studying protein conformational dynamics. A sliding count window with a fixed lag time is widely used to sample sub-trajectories for transition counting and MSM construction. However, sub-trajectories sampled with a fixed lag time may not perform well under different selections of lag time, which requires strong prior practice and leads to less robust estimation. To alleviate it, we propose a novel stochastic method from a Poisson process to generate perturbative lag time for sub-trajectory sampling and utilize it to construct a Markov chain. Comprehensive evaluations on the double-well system, WW domain, BPTI, and RBD-ACE2 complex of SARS-CoV-2 reveal that our algorithm significantly increases the robustness and power of a constructed MSM without disturbing the Markovian properties. Furthermore, the superiority of our algorithm is amplified for slow dynamic modes in complex biological processes.
更多查看译文
关键词
stochastic lag time parameterization,markov state models,protein,dynamics
AI 理解论文
溯源树
样例
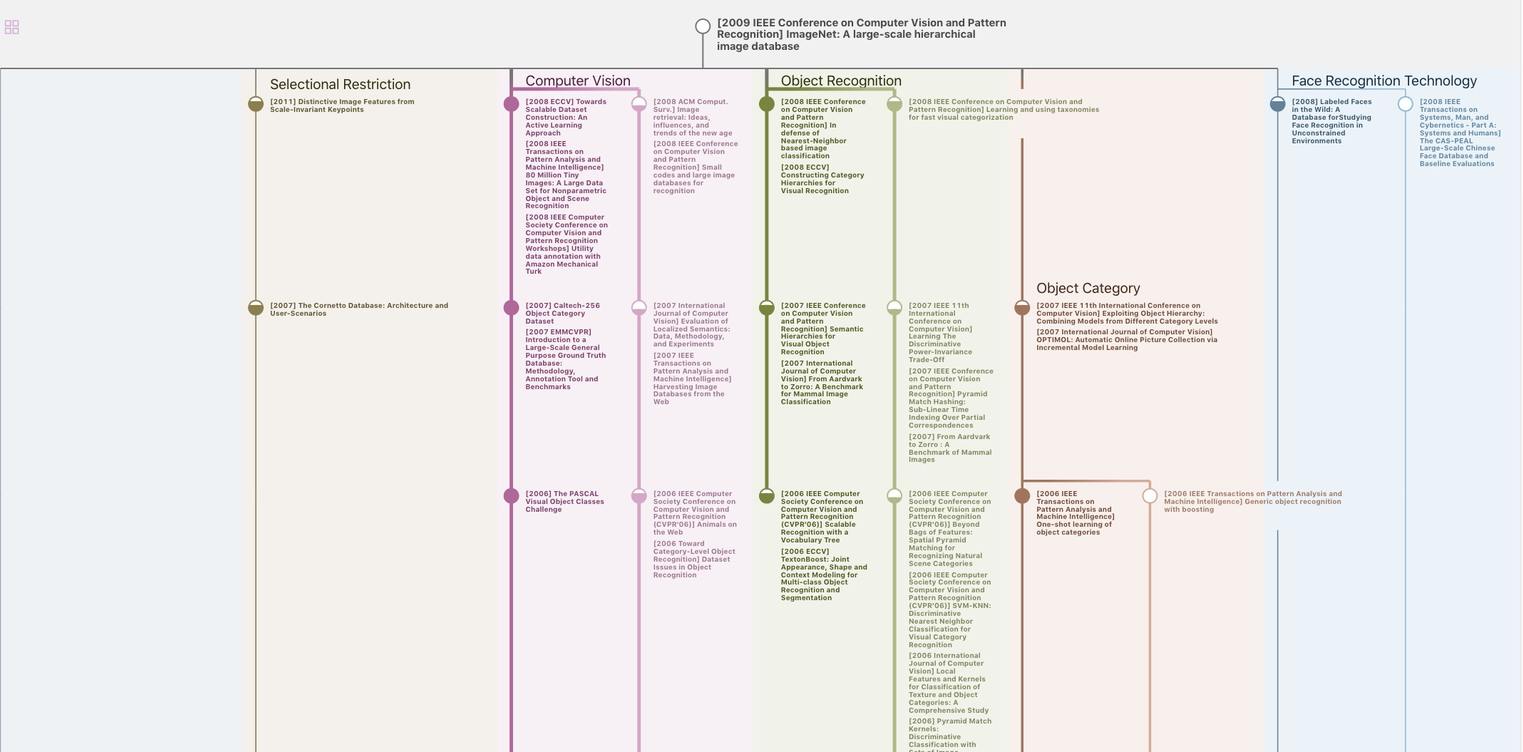
生成溯源树,研究论文发展脉络
Chat Paper
正在生成论文摘要