Word Order Matters when you Increase Masking
emnlp 2022(2022)
摘要
Word order, an essential property of natural languages, is injected in Transformer-based neural language models using position encoding. However, recent experiments have shown that explicit position encoding is not always useful, since some models without such feature managed to achieve state-of-the art performance on some tasks. To understand better this phenomenon, we examine the effect of removing position encodings on the pre-training objective itself (i.e., masked language modelling), to test whether models can reconstruct position information from co-occurrences alone. We do so by controlling the amount of masked tokens in the input sentence, as a proxy to affect the importance of position information for the task. We find that the necessity of position information increases with the amount of masking, and that masked language models without position encodings are not able to reconstruct this information on the task. These findings point towards a direct relationship between the amount of masking and the ability of Transformers to capture order-sensitive aspects of language using position encoding.
更多查看译文
关键词
order
AI 理解论文
溯源树
样例
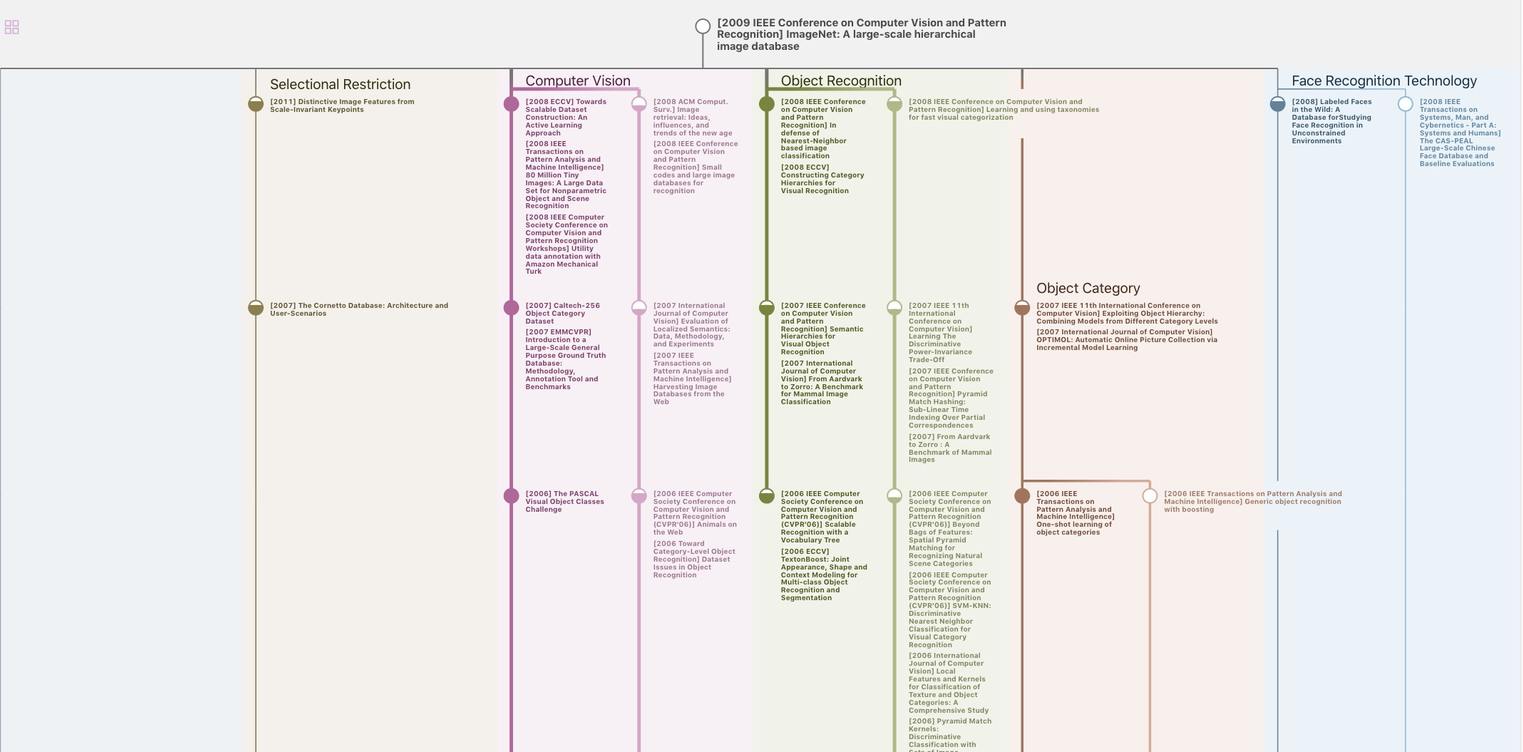
生成溯源树,研究论文发展脉络
Chat Paper
正在生成论文摘要