A generalized AIC for models with singularities and boundaries
arxiv(2022)
摘要
The Akaike information criterion (AIC) is a common tool for model selection. It is frequently used in violation of regularity conditions at parameter space singularities and boundaries. The expected AIC is generally not asymptotically equivalent to its target at singularities and boundaries, and convergence to the target at nearby parameter points may be slow. We develop a generalized AIC for candidate models with or without singularities and boundaries. We show that the expectation of this generalized form converges everywhere in the parameter space, and its convergence can be faster than that of the AIC. We illustrate the generalized AIC on example models from phylogenomics, showing that it can outperform the AIC and gives rise to an interpolated effective number of model parameters, which can differ substantially from the number of parameters near singularities and boundaries. We outline methods for estimating the often unknown generating parameter and bias correction term of the generalized AIC.
更多查看译文
关键词
generalized aic,singularities,models,boundaries
AI 理解论文
溯源树
样例
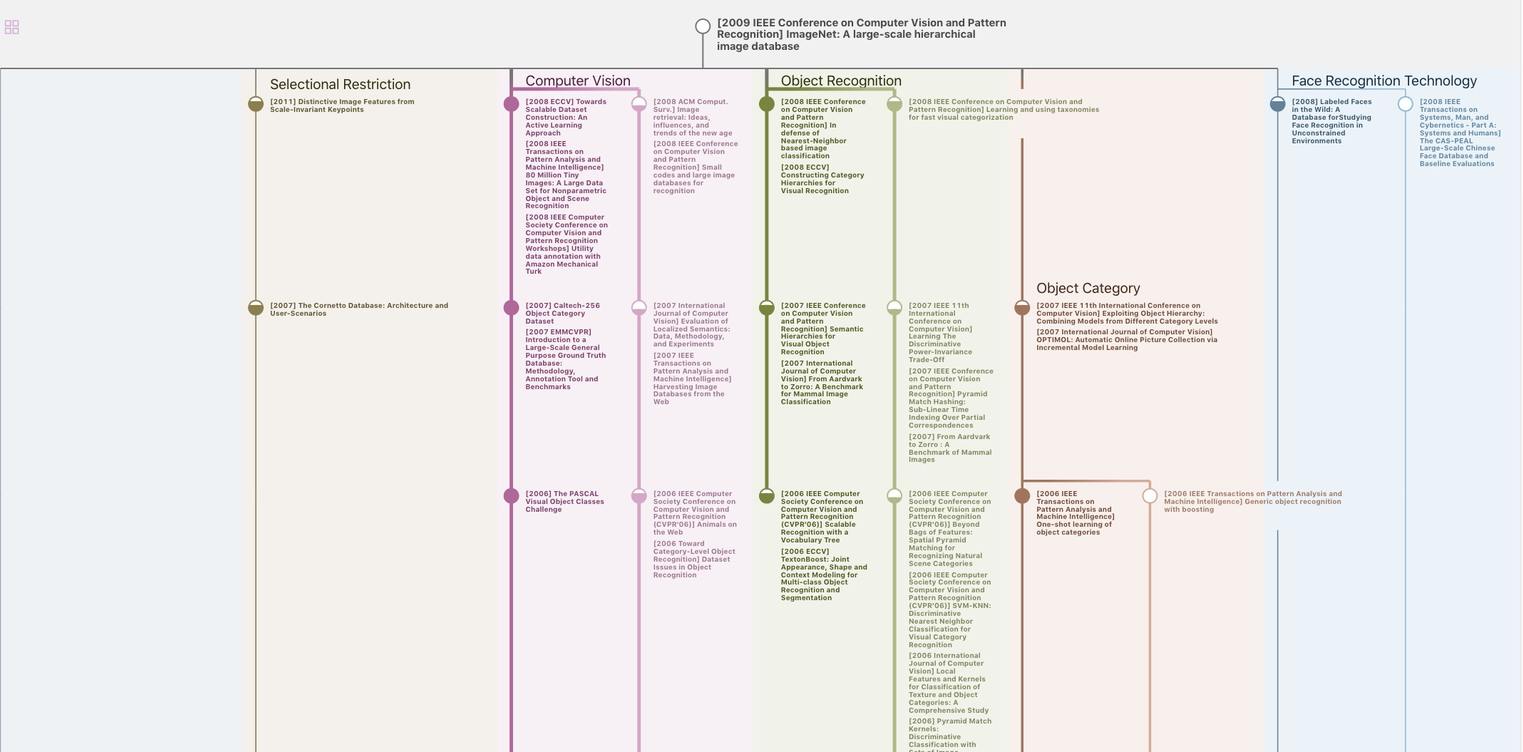
生成溯源树,研究论文发展脉络
Chat Paper
正在生成论文摘要