ABC: Adversarial Behavioral Cloning for Offline Mode-Seeking Imitation Learning
arxiv(2022)
摘要
Given a dataset of expert agent interactions with an environment of interest, a viable method to extract an effective agent policy is to estimate the maximum likelihood policy indicated by this data. This approach is commonly referred to as behavioral cloning (BC). In this work, we describe a key disadvantage of BC that arises due to the maximum likelihood objective function; namely that BC is mean-seeking with respect to the state-conditional expert action distribution when the learner's policy is represented with a Gaussian. To address this issue, we introduce a modified version of BC, Adversarial Behavioral Cloning (ABC), that exhibits mode-seeking behavior by incorporating elements of GAN (generative adversarial network) training. We evaluate ABC on toy domains and a domain based on Hopper from the DeepMind Control suite, and show that it outperforms standard BC by being mode-seeking in nature.
更多查看译文
关键词
adversarial behavioral cloning,mode-seeking
AI 理解论文
溯源树
样例
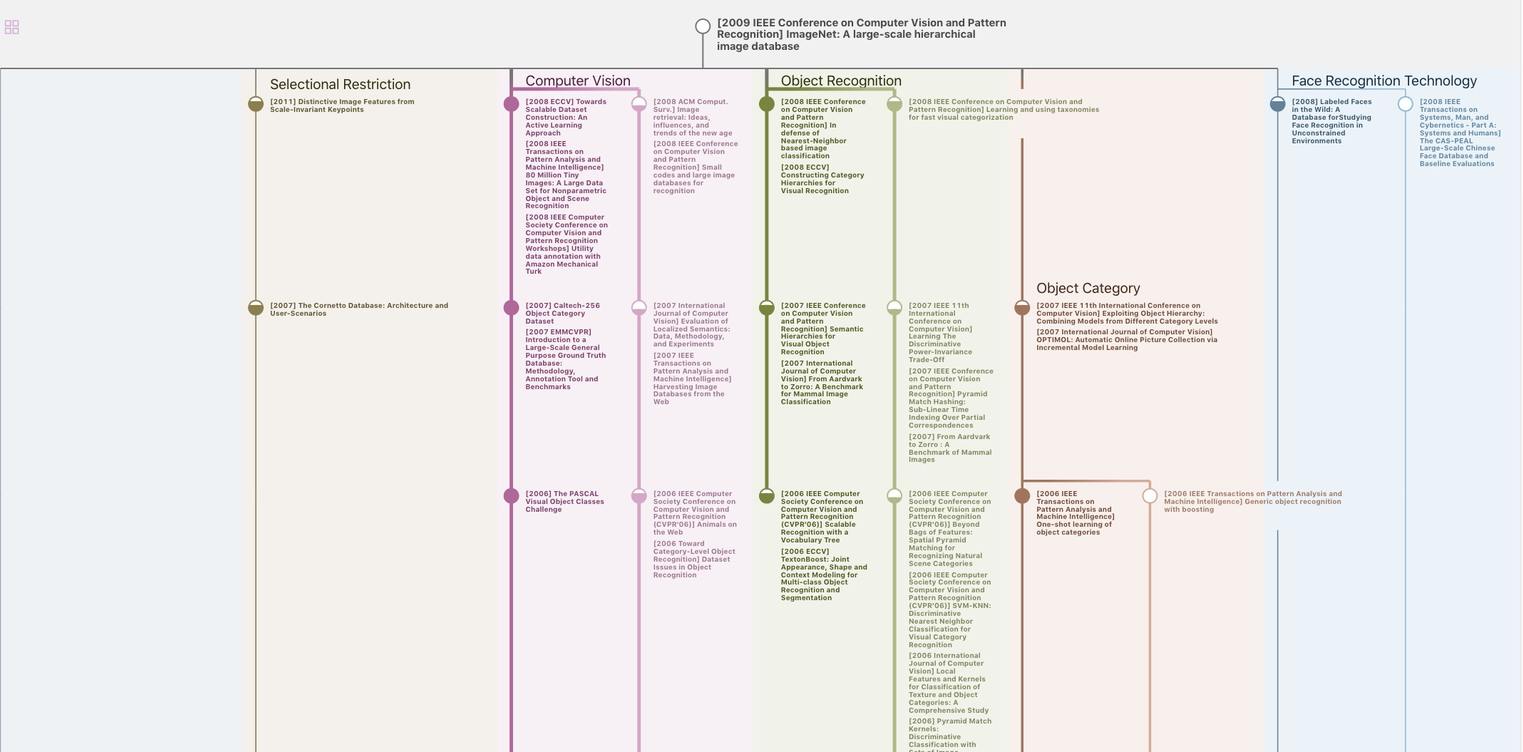
生成溯源树,研究论文发展脉络
Chat Paper
正在生成论文摘要