Causal Discovery in Linear Latent Variable Models Subject to Measurement Error
NeurIPS 2022(2022)
摘要
We focus on causal discovery in the presence of measurement error in linear systems where the mixing matrix, i.e., the matrix indicating the independent exogenous noise terms pertaining to the observed variables, is identified up to permutation and scaling of the columns. We demonstrate a somewhat surprising connection between this problem and causal discovery in the presence of unobserved parentless causes, in the sense that there is a mapping, given by the mixing matrix, between the underlying models to be inferred in these problems. Consequently, any identifiability result based on the mixing matrix for one model translates to an identifiability result for the other model. We characterize to what extent the causal models can be identified under a two-part faithfulness assumption. Under only the first part of the assumption (corresponding to the conventional definition of faithfulness), the structure can be learned up to the causal ordering among an ordered grouping of the variables but not all the edges across the groups can be identified. We further show that if both parts of the faithfulness assumption are imposed, the structure can be learned up to a more refined ordered grouping. As a result of this refinement, for the latent variable model with unobserved parentless causes, the structure can be identified. Based on our theoretical results, we propose causal structure learning methods for both models, and evaluate their performance on synthetic data.
更多查看译文
关键词
Causal discovery,Measurement error,Latent variable models,Faithfulness,Structural identification
AI 理解论文
溯源树
样例
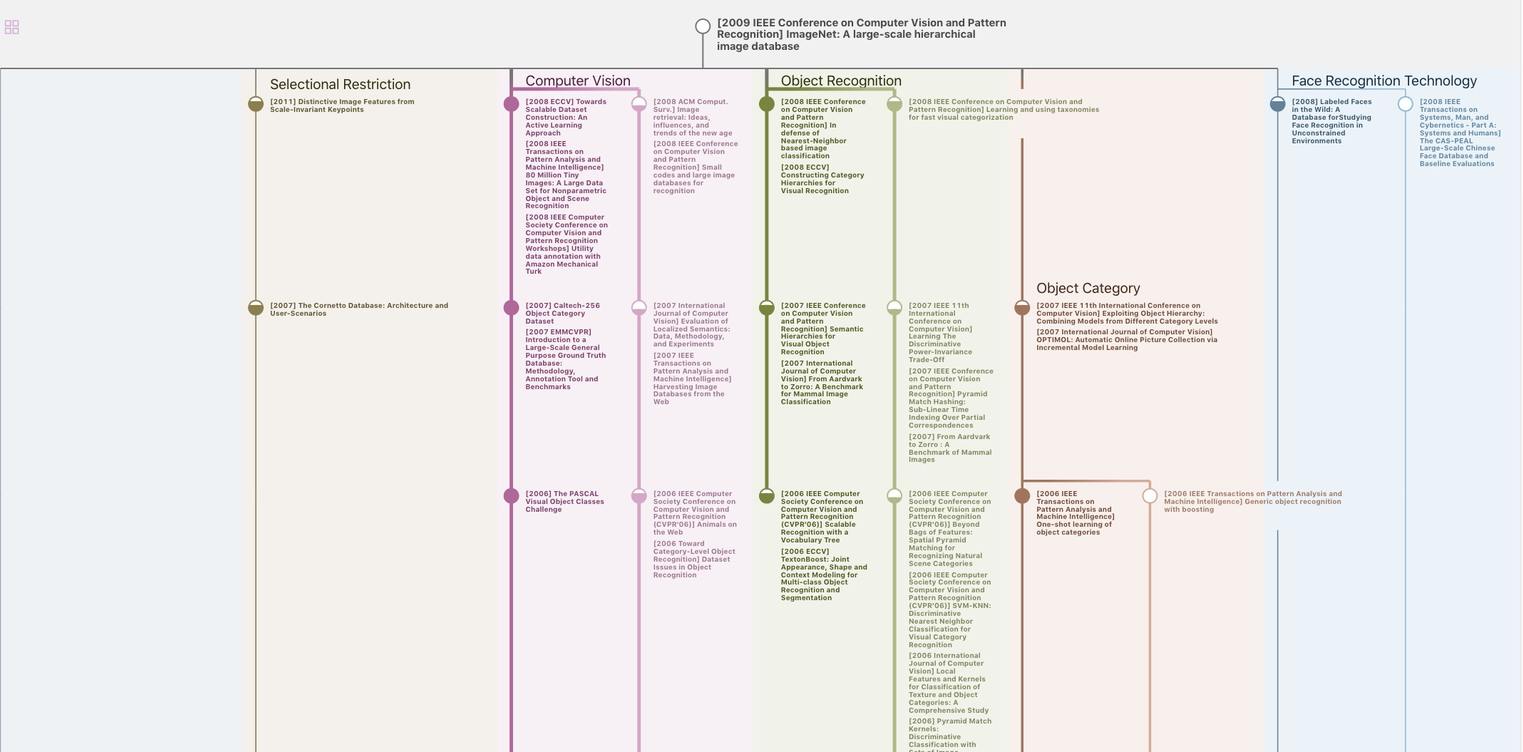
生成溯源树,研究论文发展脉络
Chat Paper
正在生成论文摘要