On the Algorithmic Stability and Generalization of Adaptive Optimization Methods
arxiv(2022)
摘要
Despite their popularity in deep learning and machine learning in general, the theoretical properties of adaptive optimizers such as Adagrad, RMSProp, Adam or AdamW are not yet fully understood. In this paper, we develop a novel framework to study the stability and generalization of these optimization methods. Based on this framework, we show provable guarantees about such properties that depend heavily on a single parameter $\beta_2$. Our empirical experiments support our claims and provide practical insights into the stability and generalization properties of adaptive optimization methods.
更多查看译文
关键词
adaptive optimization
AI 理解论文
溯源树
样例
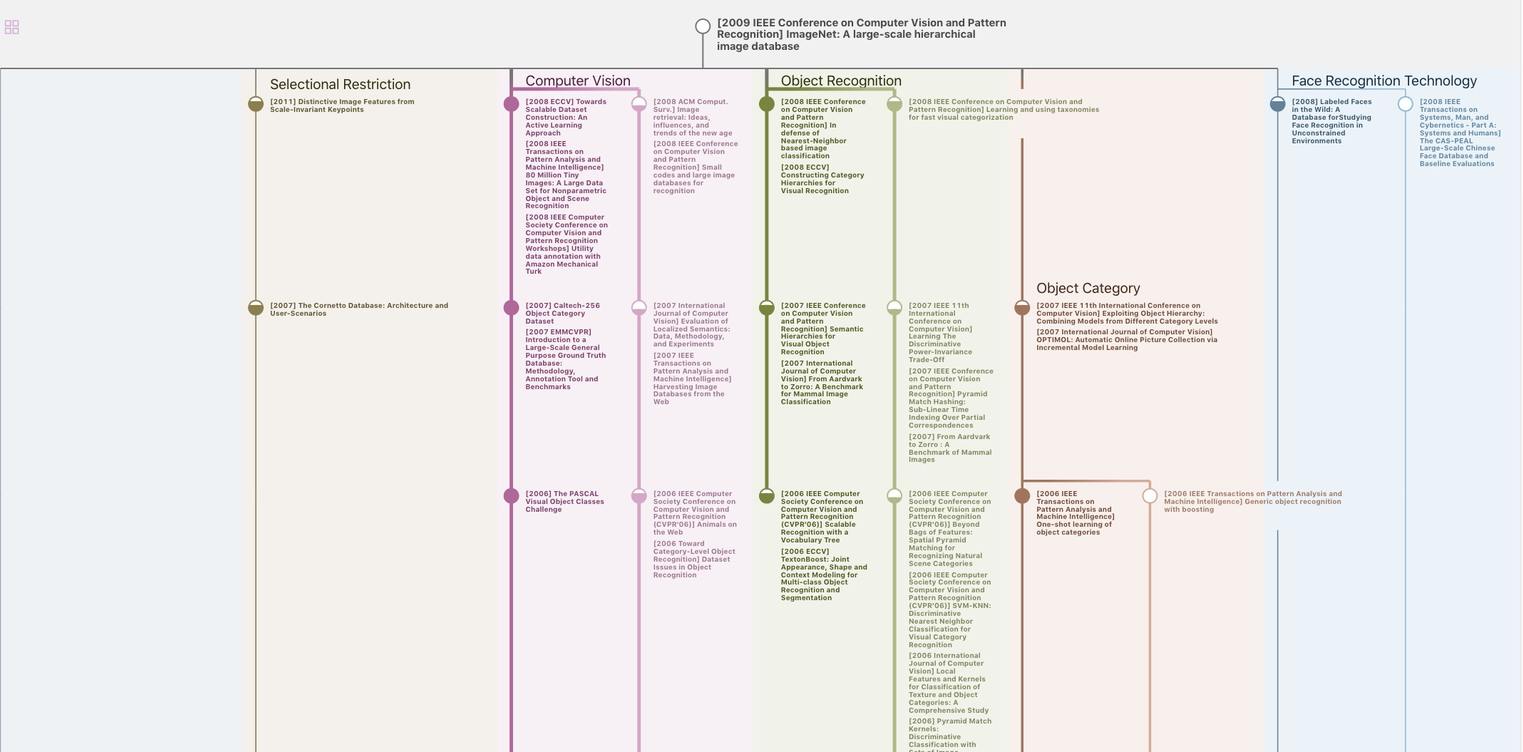
生成溯源树,研究论文发展脉络
Chat Paper
正在生成论文摘要