Edge Based Priority-Aware Dynamic Resource Allocation for Internet of Things Networks
ENTROPY(2022)
摘要
The exponential growth of the edge-based Internet-of-Things (IoT) services and its ecosystems has recently led to a new type of communication network, the Low Power Wide Area Network (LPWAN). This standard enables low-power, long-range, and low-data-rate communications. Long Range Wide Area Network (LoRaWAN) is a recent standard of LPWAN that incorporates LoRa wireless into a networked infrastructure. Consequently, the consumption of smart End Devices (EDs) is a major challenge due to the highly dense network environment characterised by limited battery life, spectrum coverage, and data collisions. Intelligent and efficient service provisioning is an urgent need of a network to streamline the networks and solve these problems. This paper proposes a Dynamic Reinforcement Learning Resource Allocation (DRLRA) approach to allocate efficient resources such as channel, Spreading Factor (SF), and Transmit Power (Tp) to EDs that ultimately improve the performance in terms of consumption and reliability. The proposed model is extensively simulated and evaluated with the currently implemented algorithms such as Adaptive Data Rate (ADR) and Adaptive Priority-aware Resource Allocation (APRA) using standard and advanced evaluation metrics. The proposed work is properly cross validated to show completely unbiased results.
更多查看译文
关键词
LPWAN,LoRaWAN,QoS,network,scalability,resource allocation,congestion,channel,Internet of Things,5G
AI 理解论文
溯源树
样例
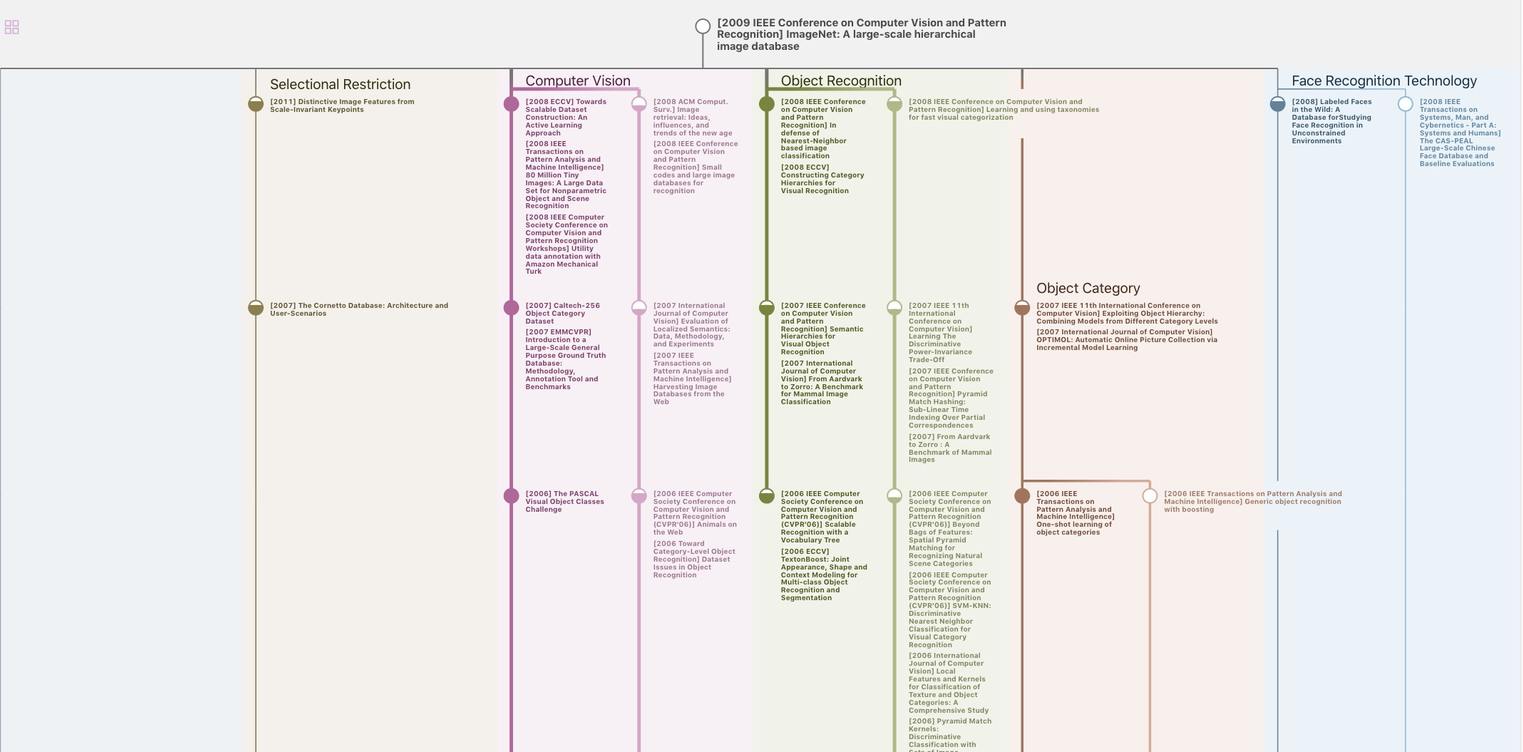
生成溯源树,研究论文发展脉络
Chat Paper
正在生成论文摘要