Class-Balanced Contrastive Learning for Fine-Grained Airplane Detection
IEEE GEOSCIENCE AND REMOTE SENSING LETTERS(2022)
摘要
Airplane detection and fine-grained recognition in remote sensing images are challenging due to class imbalance and high interclass indistinction. To alleviate these issues, we propose a class-balanced contrastive learning (CBCL) approach for airplane detection to exploit the correlation between samples in different images, which is rarely explored in previous research. Specifically, we first dynamically build class-balanced memory queues during training, which mitigates class imbalance by memorizing training samples. Upon class-balanced memory queues, hard triplet contrastive learning is introduced to increase the interclass discriminability, which enforces the maximum distance of the positive sample pair to be smaller than the minimum distance of the negative sample pair. We integrate the proposed CBCL strategy into oriented object detection frameworks for fine-grained airplane detection. The experimental results on the FAIR1M dataset reveal that several state-of-the-art algorithms with CBCL achieve significantly improvements.
更多查看译文
关键词
Airplane detection,contrastive learning,fine-grained recognition,remote sensing images
AI 理解论文
溯源树
样例
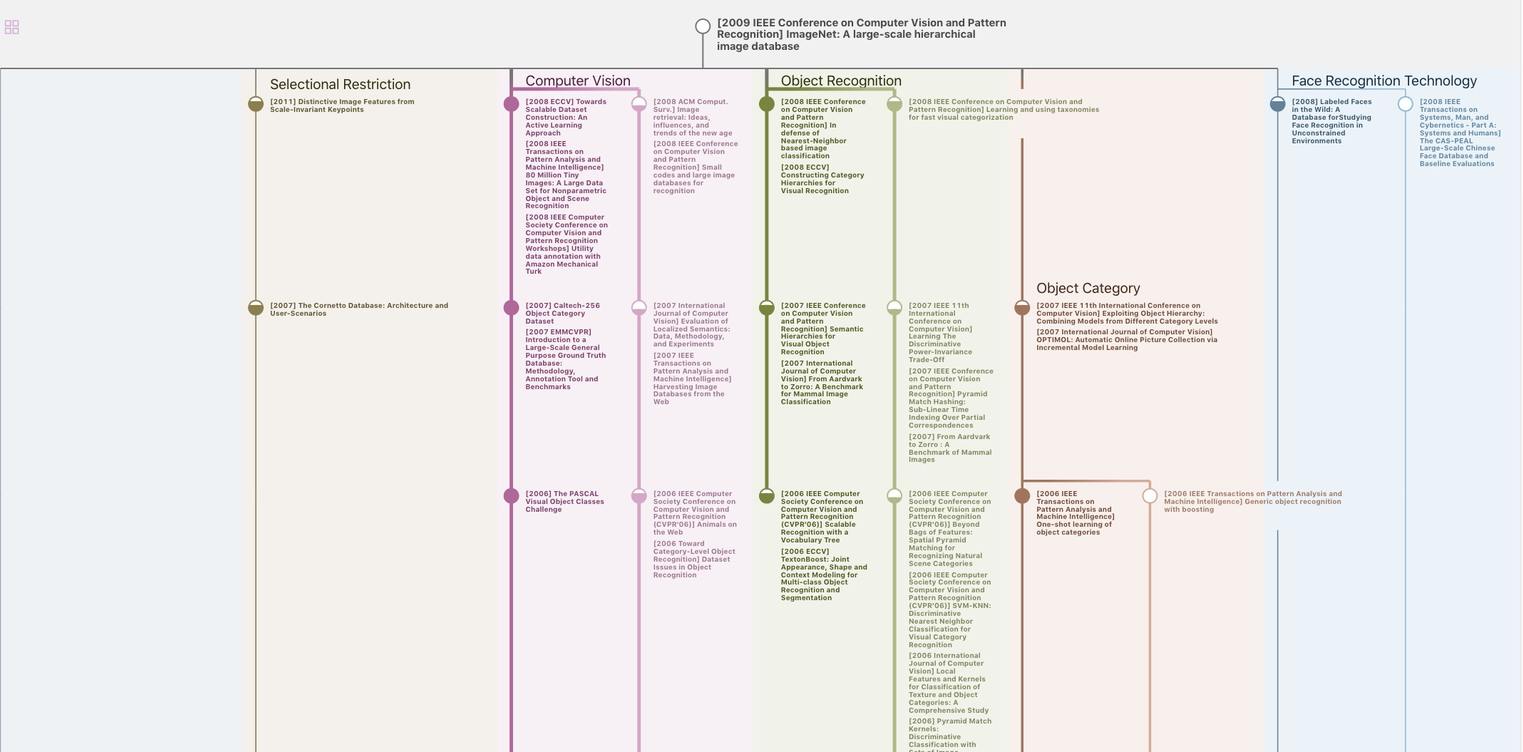
生成溯源树,研究论文发展脉络
Chat Paper
正在生成论文摘要