WalkGAN: Network Representation Learning With Sequence-Based Generative Adversarial Networks
IEEE TRANSACTIONS ON NEURAL NETWORKS AND LEARNING SYSTEMS(2022)
摘要
Network representation learning, also known as network embedding, aims to learn the low-dimensional representations of vertices while capturing and preserving the network structure. For real-world networks, the edges that represent some important relationships between the vertices of a network may be missed and may result in degenerated performance. The existing methods usually treat missing edges as negative samples, thereby ignoring the true connections between two vertices in a network. To capture the true network structure effectively, we propose a novel network representation learning method called WalkGAN, where random walk scheme and generative adversarial networks (GAN) are incorporated into a network embedding framework. Specifically, WalkGAN leverages GAN to generate the synthetic sequences of the vertices that sufficiently simulate random walk on a network and further learn vertex representations from these vertex sequences. Thus, the unobserved links between the vertices are inferred with high probability instead of treating them as nonexistence. Experimental results on the benchmark network datasets demonstrate that WalkGAN achieves significant performance improvements for vertex classification, link prediction, and visualization tasks.
更多查看译文
关键词
Generative adversarial networks,Representation learning,Neural networks,Generators,Feature extraction,Task analysis,Learning systems,Generative adversarial networks (GANs),network representation,random walk,sequence
AI 理解论文
溯源树
样例
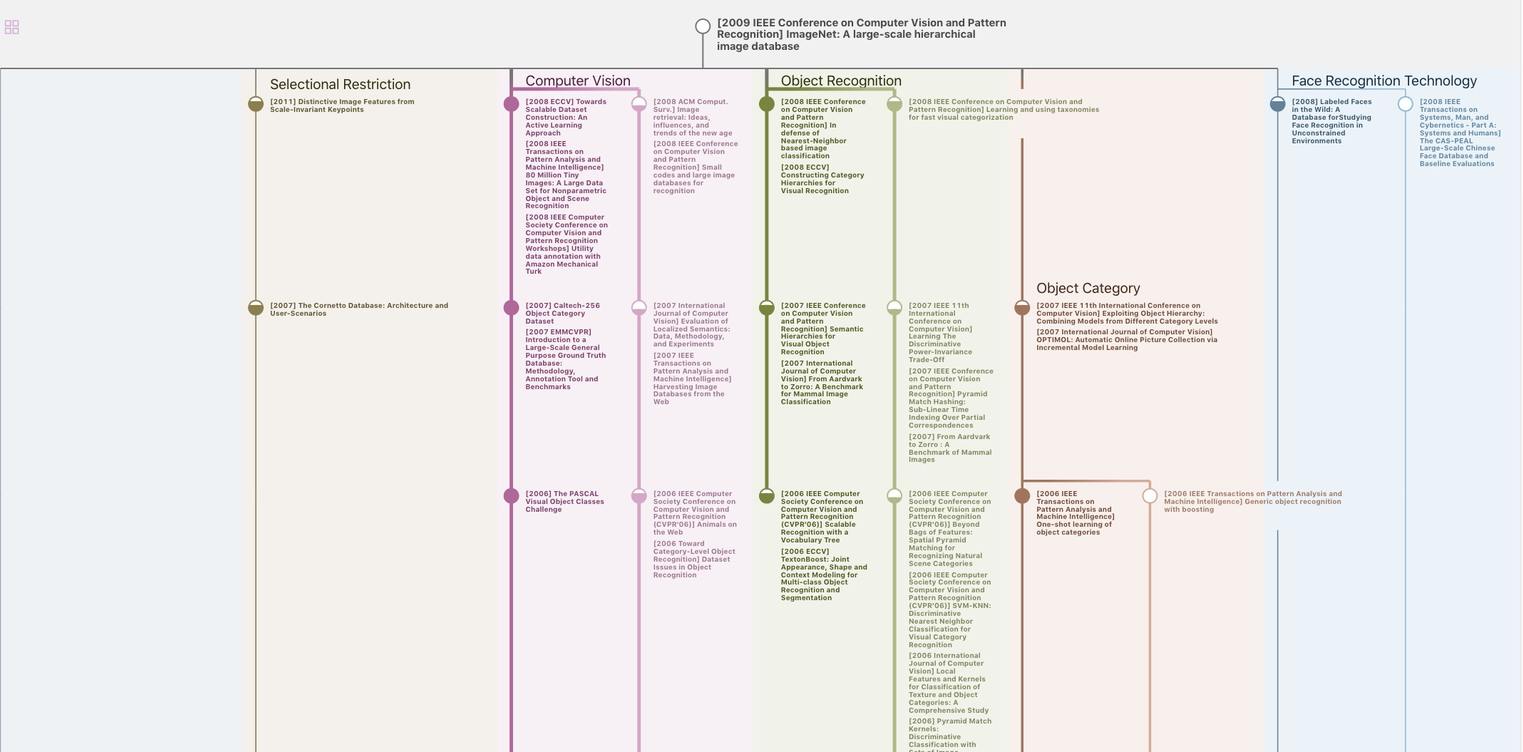
生成溯源树,研究论文发展脉络
Chat Paper
正在生成论文摘要