Group Testing with Side Information via Generalized Approximate Message Passing
arxiv(2022)
摘要
Group testing can help maintain a widespread testing program using fewer resources amid a pandemic. In a group testing setup, we are given n samples, one per individual. Each individual is either infected or uninfected. These samples are arranged into m < n pooled samples, where each pool is obtained by mixing a subset of the n individual samples. Infected individuals are then identified using a group testing algorithm. In this paper, we incorporate side information (SI) collected from contact tracing (CT) into nonadaptive/single-stage group testing algorithms. We generate different types of possible CT SI data by incorporating different possible characteristics of the spread of the disease. These data are fed into a group testing framework based on generalized approximate message passing (GAMP). Numerical results show that our GAMP-based algorithms provide improved accuracy. Compared to a loopy belief propagation algorithm, our proposed framework can increase the success probability by 0.25 for a group testing problem of n = 500 individuals with m = 100 pooled samples.
更多查看译文
关键词
generalized approximate message,testing,group
AI 理解论文
溯源树
样例
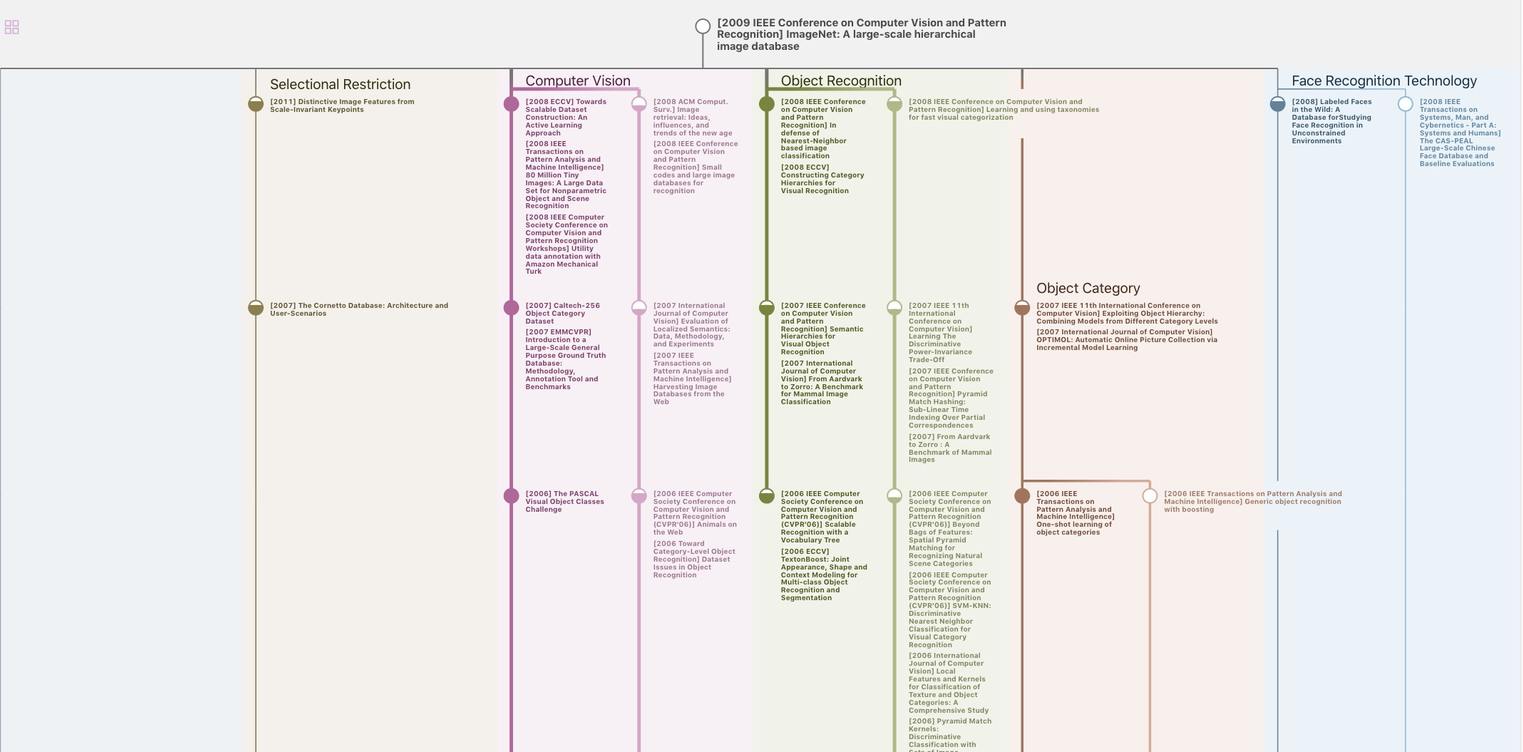
生成溯源树,研究论文发展脉络
Chat Paper
正在生成论文摘要