Fitting a collider in a quantum computer: tackling the challenges of quantum machine learning for big datasets
FRONTIERS IN ARTIFICIAL INTELLIGENCE(2023)
摘要
Current quantum systems have significant limitations affecting the processing of large datasets with high dimensionality, typical of high energy physics. In the present paper, feature and data prototype selection techniques were studied to tackle this challenge. A grid search was performed and quantum machine learning models were trained and benchmarked against classical shallow machine learning methods, trained both in the reduced and the complete datasets. The performance of the quantum algorithms was found to be comparable to the classical ones, even when using large datasets. Sequential Backward Selection and Principal Component Analysis techniques were used for feature's selection and while the former can produce the better quantum machine learning models in specific cases, it is more unstable. Additionally, we show that such variability in the results is caused by the use of discrete variables, highlighting the suitability of Principal Component analysis transformed data for quantum machine learning applications in the high energy physics context.
更多查看译文
关键词
high energy physics,quantum computing,quantum machine learning,K-means,principal component analysis,data reduction
AI 理解论文
溯源树
样例
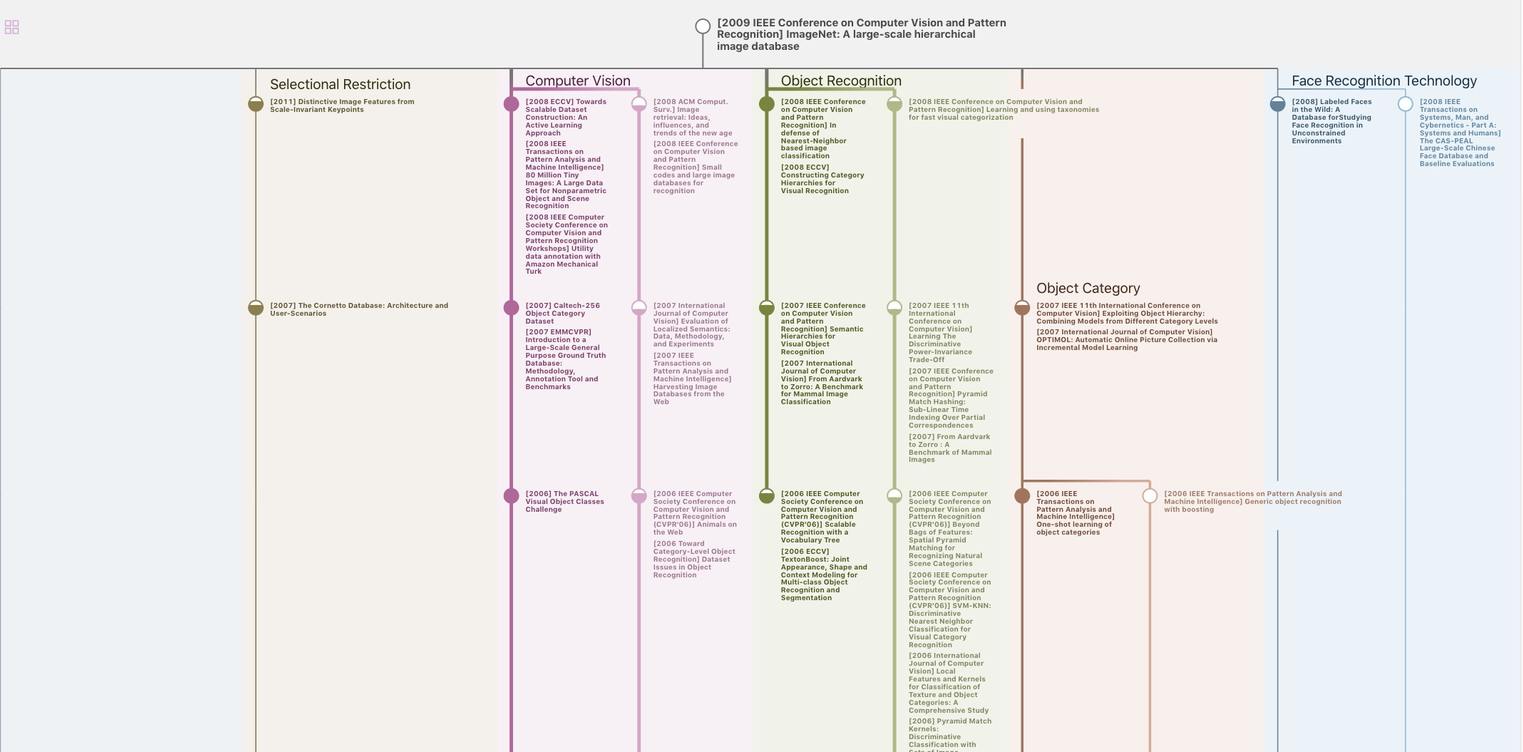
生成溯源树,研究论文发展脉络
Chat Paper
正在生成论文摘要