Coarse-to-fine Knowledge Graph Domain Adaptation based on Distantly-supervised Iterative Training
arxiv(2022)
摘要
Modern supervised learning neural network models require a large amount of
manually labeled data, which makes the construction of domain-specific
knowledge graphs time-consuming and labor-intensive. In parallel, although
there has been much research on named entity recognition and relation
extraction based on distantly supervised learning, constructing a
domain-specific knowledge graph from large collections of textual data without
manual annotations is still an urgent problem to be solved. In response, we
propose an integrated framework for adapting and re-learning knowledge graphs
from one coarse domain (biomedical) to a finer-define domain (oncology). In
this framework, we apply distant-supervision on cross-domain knowledge graph
adaptation. Consequently, no manual data annotation is required to train the
model. We introduce a novel iterative training strategy to facilitate the
discovery of domain-specific named entities and triples. Experimental results
indicate that the proposed framework can perform domain adaptation and
construction of knowledge graph efficiently.
更多查看译文
关键词
Knowledge Graph Domain Adaptation,Knowledge Graph Construction,Named Entity Recognition,Relationship Extraction
AI 理解论文
溯源树
样例
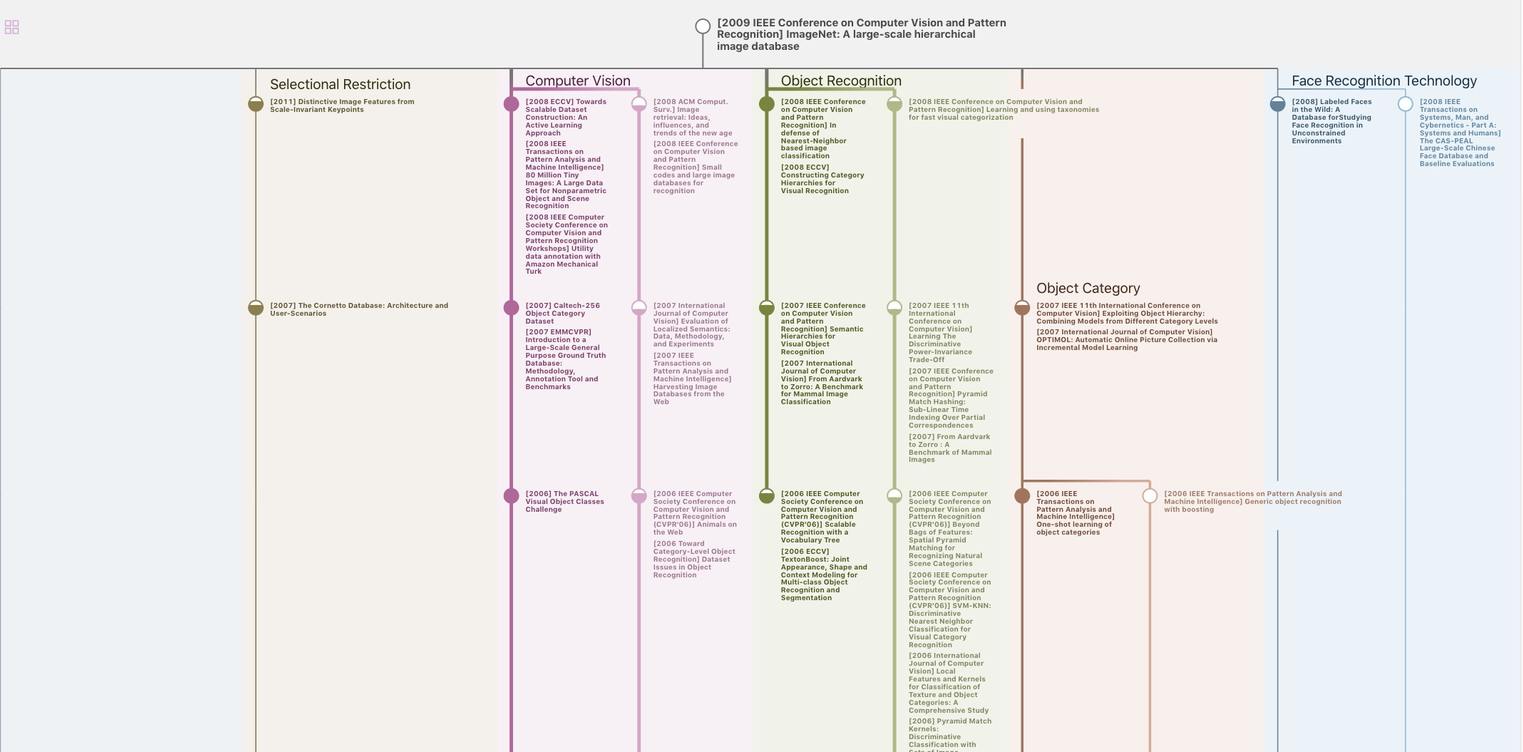
生成溯源树,研究论文发展脉络
Chat Paper
正在生成论文摘要