Bayesian learning of Causal Structure and Mechanisms with GFlowNets and Variational Bayes
arxiv(2023)
摘要
Bayesian causal structure learning aims to learn a posterior distribution over directed acyclic graphs (DAGs), and the mechanisms that define the relationship between parent and child variables. By taking a Bayesian approach, it is possible to reason about the uncertainty of the causal model. The notion of modelling the uncertainty over models is particularly crucial for causal structure learning since the model could be unidentifiable when given only a finite amount of observational data. In this paper, we introduce a novel method to jointly learn the structure and mechanisms of the causal model using Variational Bayes, which we call Variational Bayes-DAG-GFlowNet (VBG). We extend the method of Bayesian causal structure learning using GFlowNets to learn not only the posterior distribution over the structure, but also the parameters of a linear-Gaussian model. Our results on simulated data suggest that VBG is competitive against several baselines in modelling the posterior over DAGs and mechanisms, while offering several advantages over existing methods, including the guarantee to sample acyclic graphs, and the flexibility to generalize to non-linear causal mechanisms.
更多查看译文
关键词
causal structure,bayesian learning,gflownets
AI 理解论文
溯源树
样例
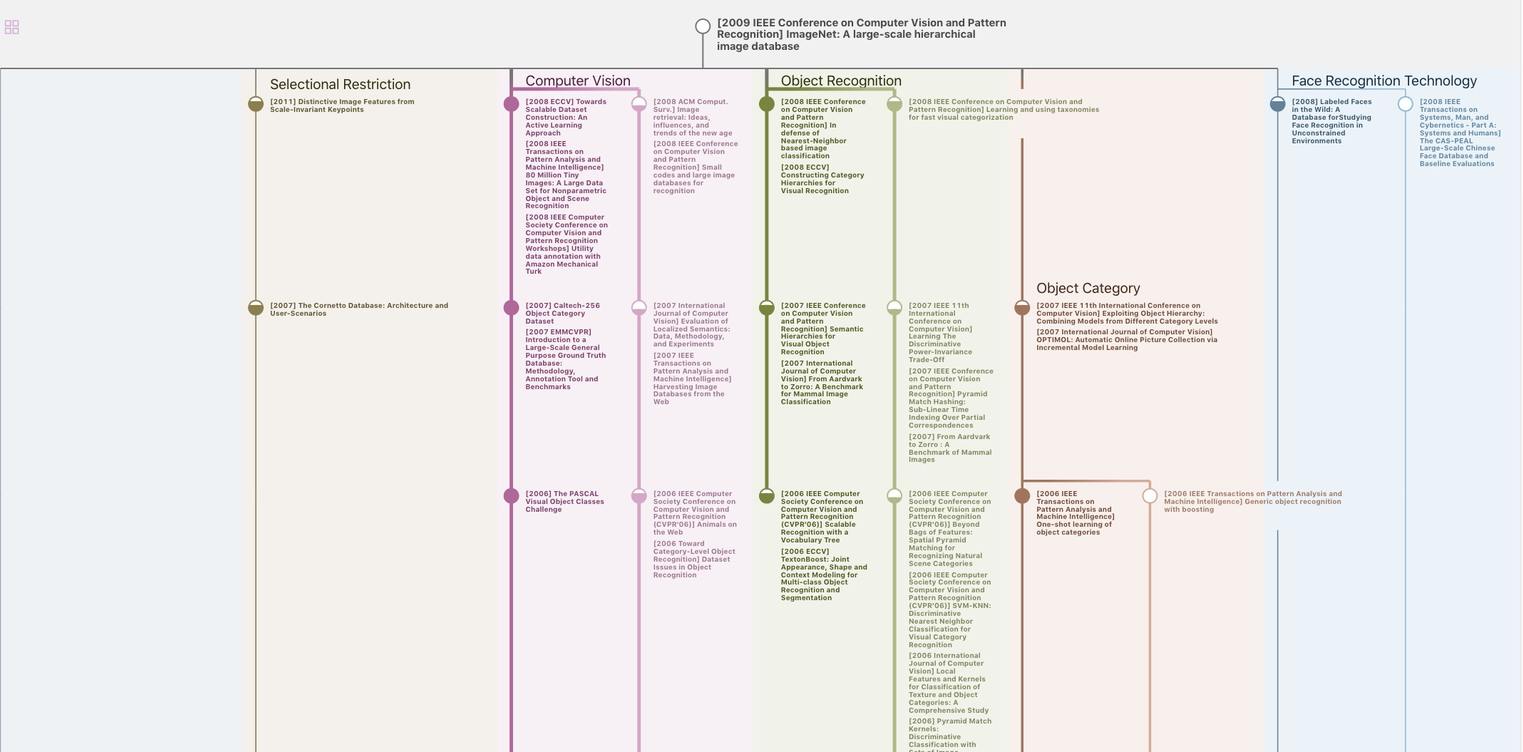
生成溯源树,研究论文发展脉络
Chat Paper
正在生成论文摘要