Deconfounded Imitation Learning
arxiv(2022)
摘要
Standard imitation learning can fail when the expert demonstrators have different sensory inputs than the imitating agent. This is because partial observability gives rise to hidden confounders in the causal graph. We break down the space of confounded imitation learning problems and identify three settings with different data requirements in which the correct imitation policy can be identified. We then introduce an algorithm for deconfounded imitation learning, which trains an inference model jointly with a latent-conditional policy. At test time, the agent alternates between updating its belief over the latent and acting under the belief. We show in theory and practice that this algorithm converges to the correct interventional policy, solves the confounding issue, and can under certain assumptions achieve an asymptotically optimal imitation performance.
更多查看译文
关键词
imitation,learning
AI 理解论文
溯源树
样例
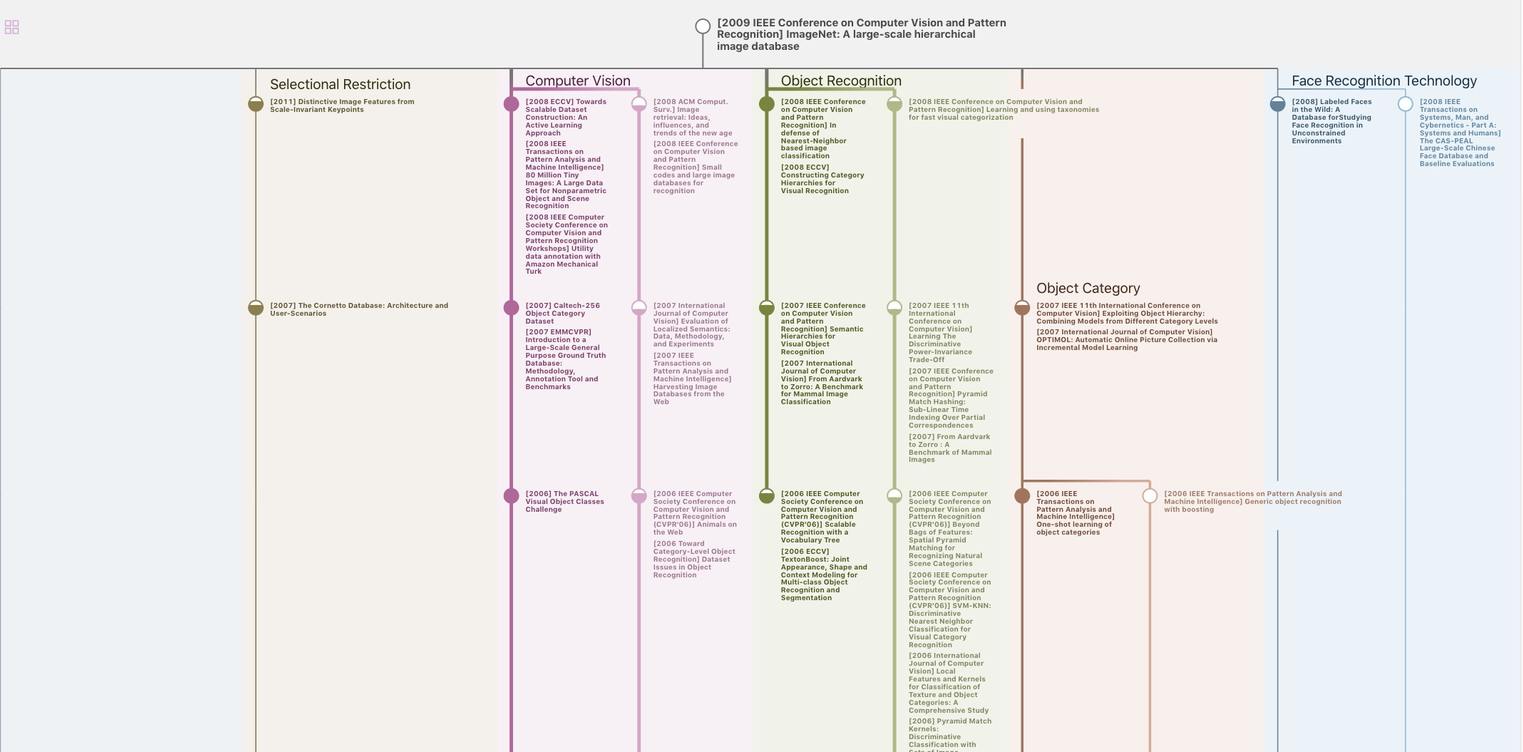
生成溯源树,研究论文发展脉络
Chat Paper
正在生成论文摘要