A Lightweight Depthwise Separable Convolution Neural Network for Screening Covid-19 Infection from Chest CT and X-ray Images
2022 18th International Conference on Distributed Computing in Sensor Systems (DCOSS)(2022)
摘要
Because Covid-19 spreads swiftly in the community, an automatic detection system is required to prevent Covid-19 from spreading among humans as a rapid diagnostic tool. In this paper, we propose to employ Convolution Neural Networks to detect coronavirus-infected patients using Computed Tomography and X-ray images. In addition, we look into the transfer learning of a deep CNN model, DenseNet201 for detecting infection from CT and X-ray scans. Grid Search optimization is utilized to select ideal values for hyper-parameters, while image augmentation is employed to increase the model’s capacity to generalize. We further modify DenseNet architecture to incorporate a depthwise separable convolution for detecting coronavirus-infected patients utilizing CT and X-ray images. Interestingly, all of the proposed models scored greater than 94% accuracy, which is equivalent to or higher than the accuracy of earlier deep learning models. Further, we demonstrate that depthwise separable convolution reduces the training time and computation complexity.
更多查看译文
关键词
Covid-19,CT Images,Convolution Neural Networks,DenseNet201,Transfer learning,Grid Search Optimization,Depthwise separable convolution
AI 理解论文
溯源树
样例
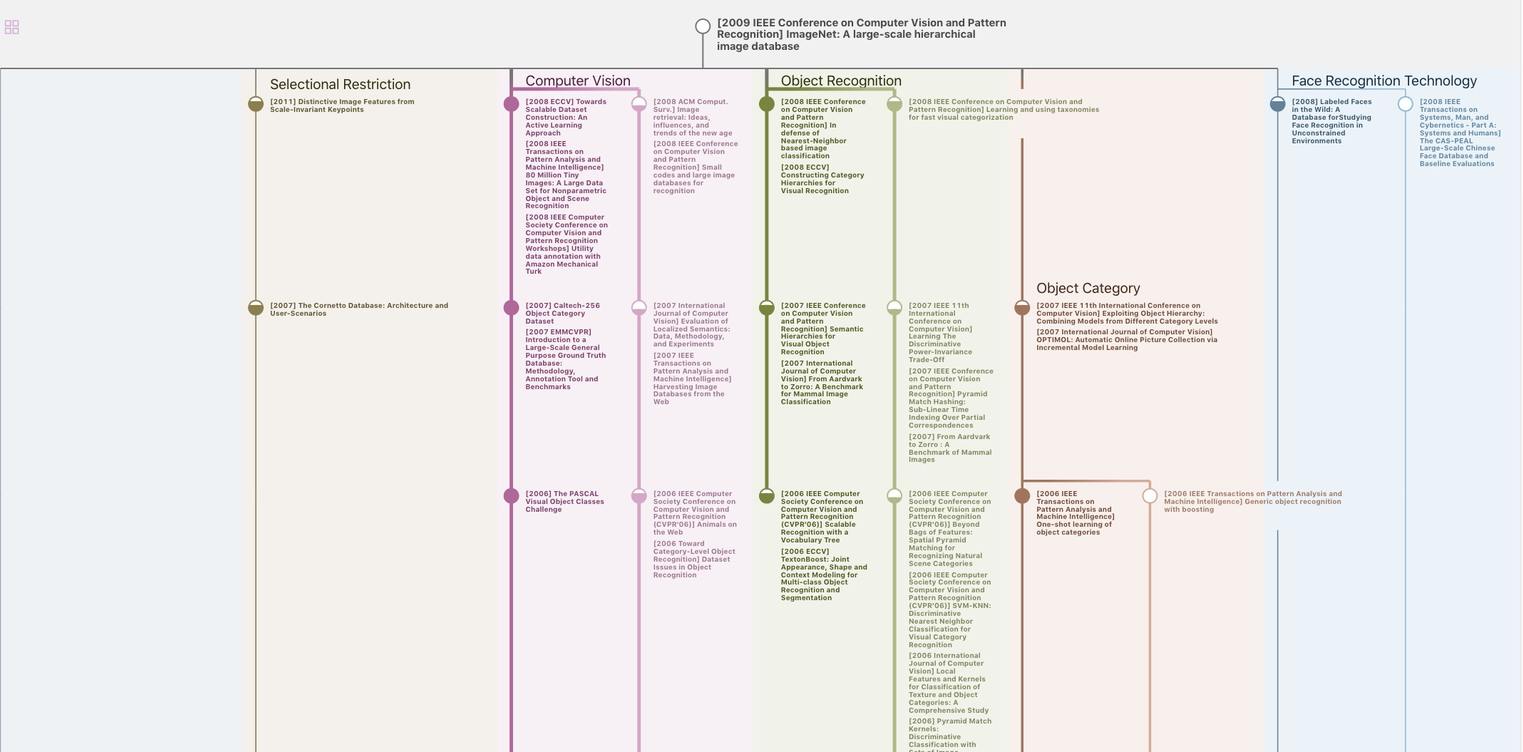
生成溯源树,研究论文发展脉络
Chat Paper
正在生成论文摘要