Generating Photospheric Vector Magnetograms of Solar Active Regions for SOHO/MDI Using SDO/HMI and BBSO Data with Deep Learning
arxiv(2023)
摘要
Solar activity is often caused by the evolution of solar magnetic fields. Magnetic field parameters derived from photospheric vector magnetograms of solar active regions (ARs) have been used to analyze and forecast eruptive events, such as solar flares and coronal mass ejections. Unfortunately, the most recent Solar Cycle 24 was relatively weak with few large flares, though it is the only solar cycle in which consistent time-sequence vector magnetograms have been available through the Helioseismic and Magnetic Imager (HMI) on board the Solar Dynamics Observatory (SDO) since its launch in 2010. In this work, we look into another major instrument, namely the Michelson Doppler Imager (MDI) on board the Solar and Heliospheric Observatory (SOHO) from 1996 to 2010. The data archive of SOHO/MDI covers a more active Solar Cycle 23 with many large flares. However, SOHO/MDI only has line-of-sight (LOS) magnetograms. We propose a new deep learning method, named MagNet, to learn from combined LOS magnetograms, B_x and B_y , taken by SDO/HMI, along with H α observations collected by the Big Bear Solar Observatory (BBSO), and to generate synthetic vector components B_x' and B_y' of ARs. These generated vector components, together with observational LOS data, would form vector magnetograms for SOHO/MDI. In this way, we can expand the availability of vector magnetograms to the period from 1996 to present. Experimental results demonstrate the good performance of the MagNet method. To our knowledge, this is the first time that deep learning has been used to generate photospheric vector magnetograms of ARs for SOHO/MDI using SDO/HMI and H α data.
更多查看译文
关键词
Magnetic fields,Photosphere,Neural networks,Data analysis
AI 理解论文
溯源树
样例
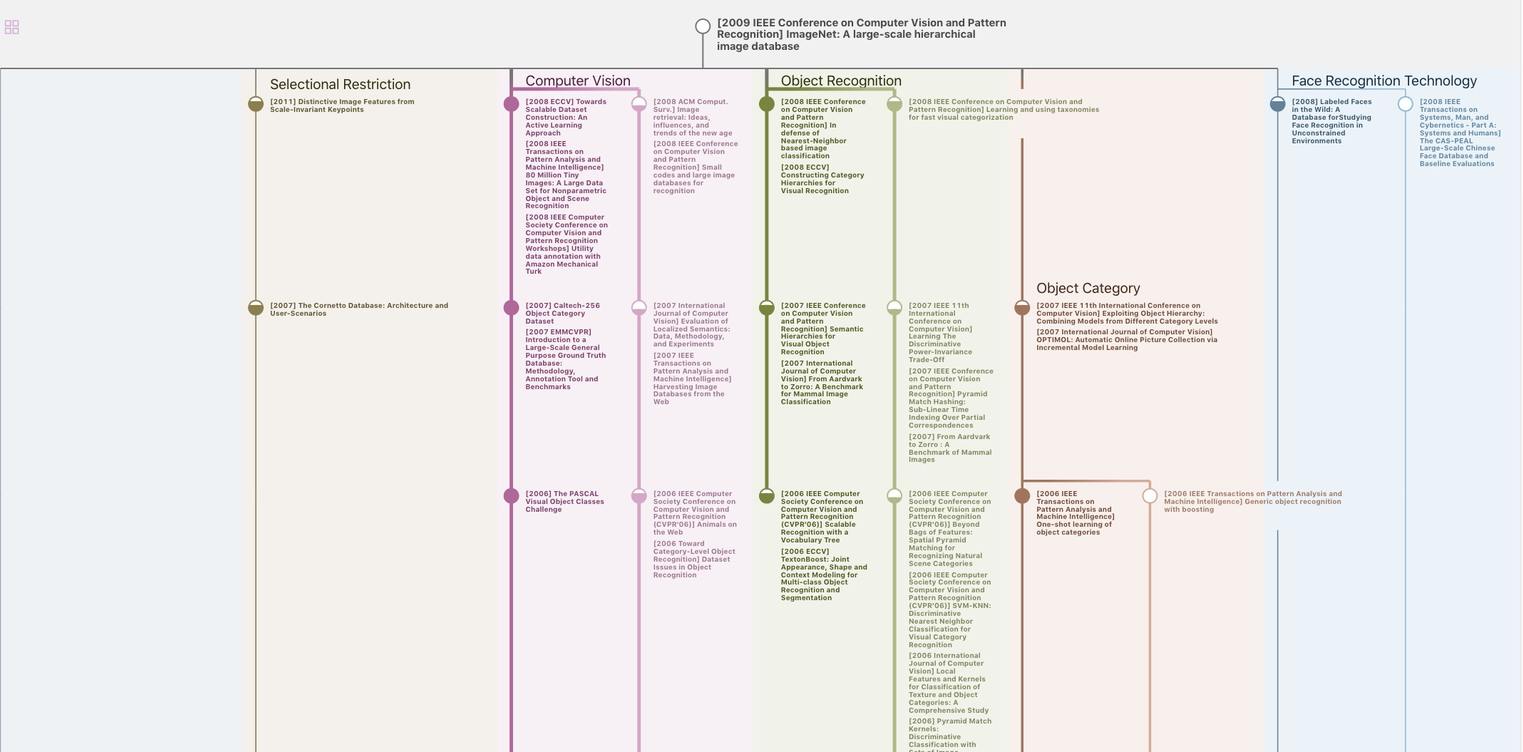
生成溯源树,研究论文发展脉络
Chat Paper
正在生成论文摘要