Artificial Prognosis of Cardiac Disease using an NN: A Data-scientific Approach in Outlier Handling
2019 4th International Conference on Electrical Information and Communication Technology (EICT)(2019)
摘要
Cardiovascular diseases cause approximately one third of all yearly global deaths. Addictives and physical inactivity trigger in humans discernible syndromes such as elevated blood pressure, increased blood sugar, unhealthy levels of cholesterol, portliness, chest-pain etc. which are signs of the existence of a probable heart disease. There being multiple predictive indicators of cardiac diseases, this poses a machine-learning (ML) problem. This paper proposes a three-layer, NN-based binary classifier to prognosticate presence of heart disease utilizing UCI (University of California, Irvine) ML Heart Disease repository. The feature-space has been assembled using filtering methods that employed univariate and bivariate exploratory data analysis (EDA). Extracted features have been corroborated by inferential statistics, applying two-sample t-tests and Chi-squared ( χ
2
) independence tests. The research exploits different data engineering techniques-eventually raising the accuracy to an average 88.33% and to a highest 91.66%-namely, distribution specific division, natural and unnatural handling of anomalous outliers. The consistent, stepwise improvement in performance has been k-fold cross-validated and signified by performing one sample t-tests and ANOVA-tests on k=5 runs of the techniques each. The simplistic model outperformed traditional ML and provided comparable accuracy achieved by hybrid approaches in much lower computational cost by applying statistical preprocessing on data.
更多查看译文
关键词
cardiovascular disease,exploratory data analysis,univariate and bivariate analysis,inferential statistics,t-test,Chi-squared ($\chi^{2}$) independence test,ANOVA-test
AI 理解论文
溯源树
样例
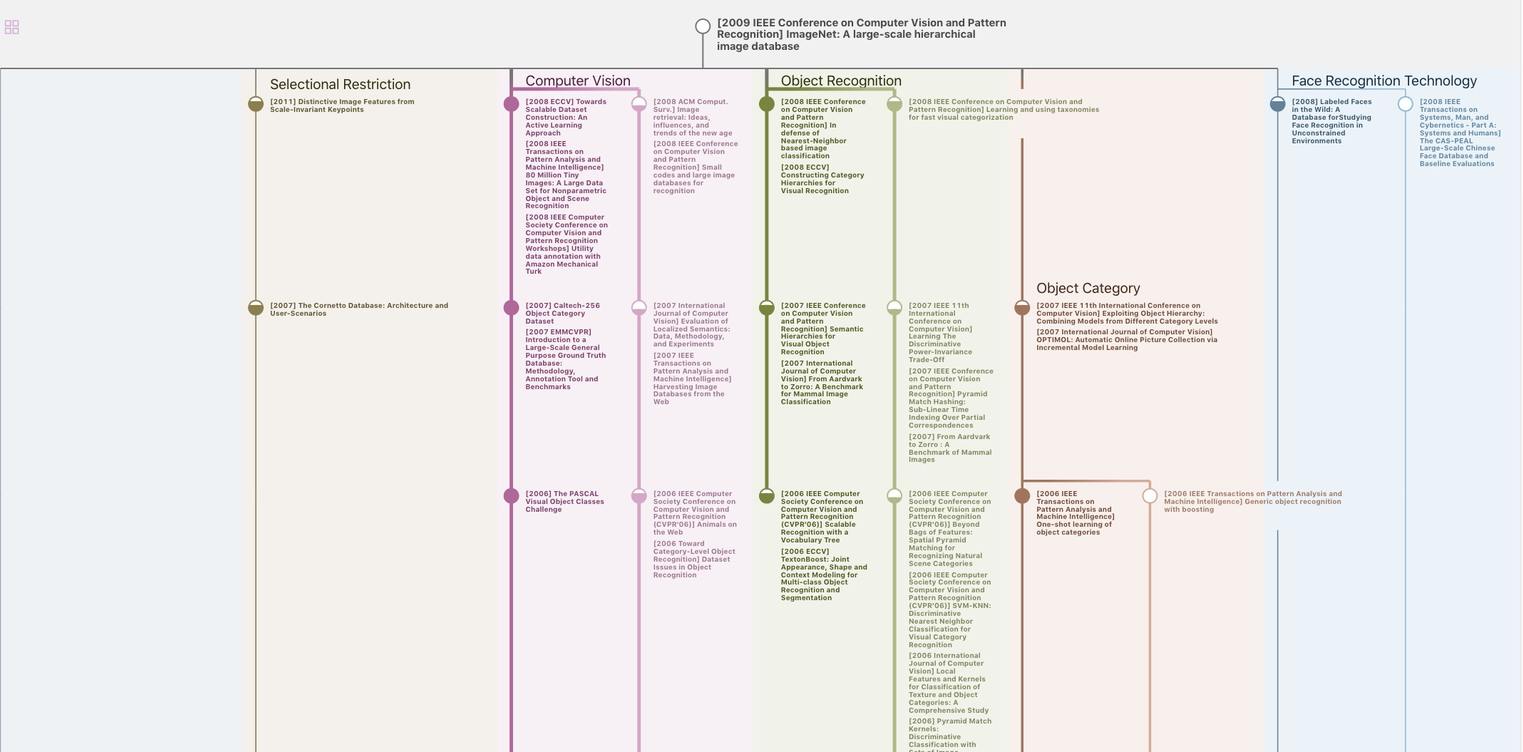
生成溯源树,研究论文发展脉络
Chat Paper
正在生成论文摘要