A Comparative Analysis of Supervised and Unsupervised Models for Detecting GPS Spoofing Attack on UAVs
2022 IEEE International Conference on Electro Information Technology (eIT)(2022)
摘要
With the increasing use of Unmanned Aerial Vehicles in military and civilian applications, the security of this technology has become one of the critical concerns. UAVs’ positioning and navigation activities are highly dependent on Global Positioning Systems as they provide accurate locations for these vehicles. However, due to the civilian GPS signals being open and unencrypted, malicious users can target them in multiple ways, including by launching Global Positioning System spoofing attacks. To address this security issue, numerous techniques have been proposed to detect and classify these attacks,including supervised machine learning techniques. However, no studies have focused on unsupervised models to detect these attacks. In this paper, we compare the performance of several supervised models with that of unsupervised models in terms of accuracy, probability of detection, probability of misdetection, probability of false alarm, processing time, training time, prediction time, and memory size. The supervised models are Gaussian Naïve Bayes, Classification and Regression Decision Tree, Logistic Regression, Random Forest, Linear-Support Vector Machine, and Artificial Neural Network. The unsupervised models are Principal Component Analysis, K-means clustering, and Autoencoder. The results show that the Classification and Regression Decision Tree model outperforms the other supervised and unsupervised models in detecting and classifying GPS spoofing attacks.
更多查看译文
关键词
classification,comparative analysis,cyber-security,cyber-attacks,clustering,GPS spoofing attacks,machine learning,neural network,spoofing detection,UAVs.
AI 理解论文
溯源树
样例
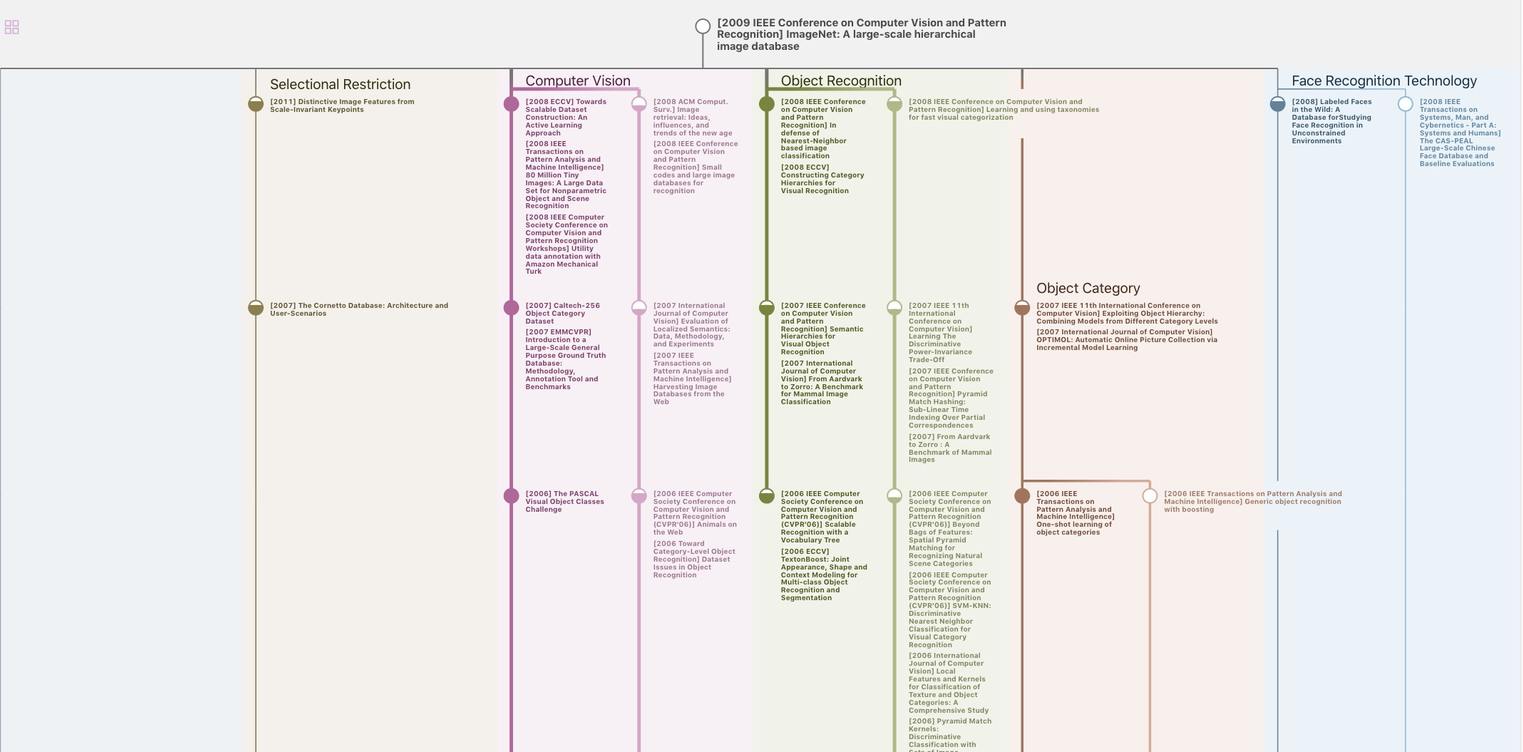
生成溯源树,研究论文发展脉络
Chat Paper
正在生成论文摘要