Mirror Segmentation via Semantic-aware Contextual Contrasted Feature Learning
ACM Transactions on Multimedia Computing, Communications, and Applications(2023)
摘要
Mirrors are everywhere in our daily lives. Existing computer vision systems do not consider mirrors, and hence may get confused by the reflected content inside a mirror, resulting in a severe performance degradation. However, separating the real content outside a mirror from the reflected content inside it is non-trivial. The key challenge is that mirrors typically reflect contents similar to their surroundings, making it very difficult to differentiate the two. In this article, we present a novel method to segment mirrors from a single RGB image. To the best of our knowledge, this is the first work to address the mirror segmentation problem with a computational approach. We make the following contributions: First, we propose a novel network, called MirrorNet+, for mirror segmentation, by modeling both contextual contrasts and semantic associations. Second, we construct the first large-scale mirror segmentation dataset, which consists of 4,018 pairs of images containing mirrors and their corresponding manually annotated mirror masks, covering a variety of daily-life scenes. Third, we conduct extensive experiments to evaluate the proposed method and show that it outperforms the related state-of-the-art detection and segmentation methods. Fourth, we further validate the effectiveness and generalization capability of the proposed semantic awareness contextual contrasted feature learning by applying MirrorNet+ to other vision tasks, i.e., salient object detection and shadow detection. Finally, we provide some applications of mirror segmentation and analyze possible future research directions. Project homepage: https://mhaiyang.github.io/TOMM2022-MirrorNet+/index.html .
更多查看译文
关键词
Mirror segmentation,contextual contrast,semantic association,dataset,reflection,deep neural network
AI 理解论文
溯源树
样例
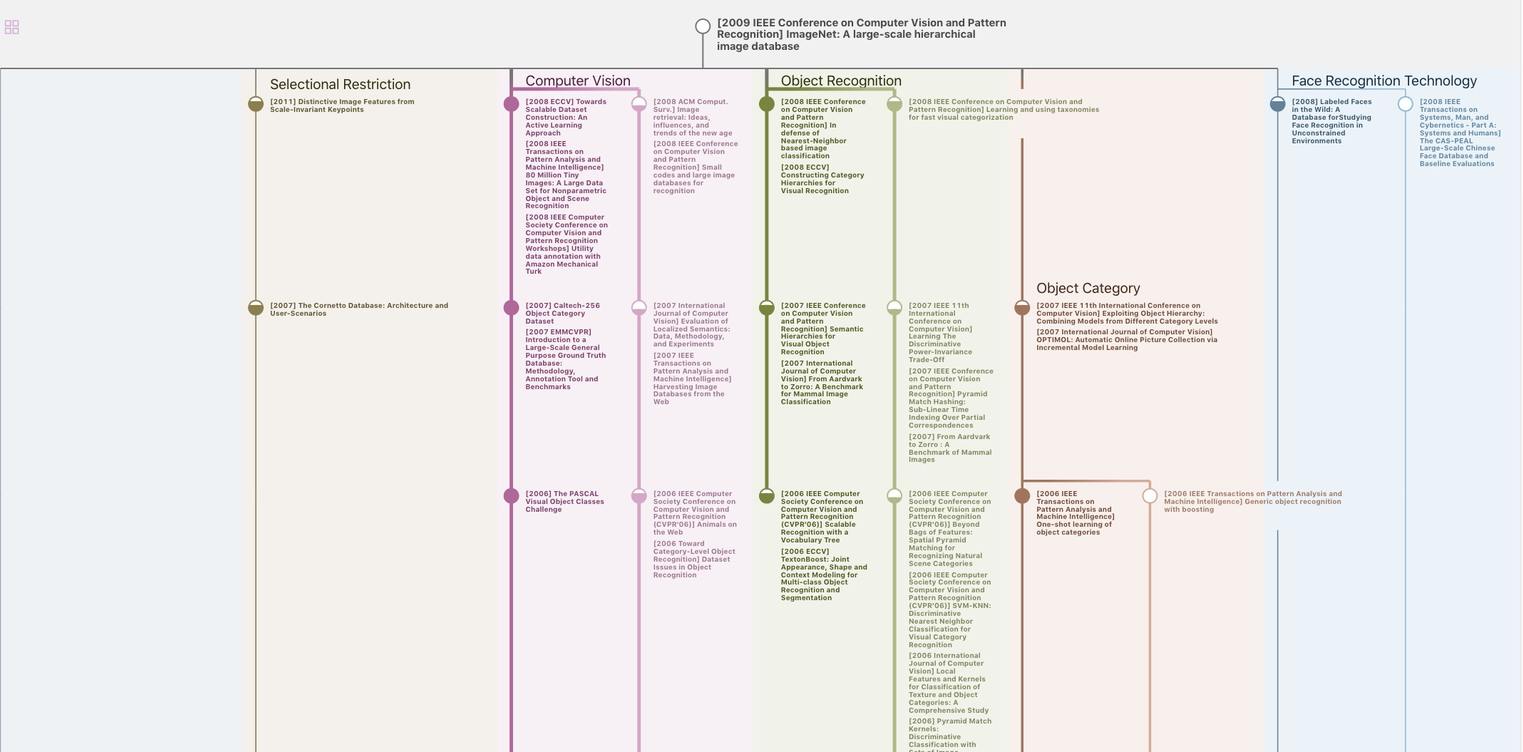
生成溯源树,研究论文发展脉络
Chat Paper
正在生成论文摘要