Performance Estimation for Efficient Image Segmentation Training of Weather Radar Algorithms
2022 IEEE High Performance Extreme Computing Conference (HPEC)(2022)
摘要
Deep Learning has a dramatically increasing demand for compute resources and a corresponding increase in the energy required to develop, explore, and test model architectures for various applications. Parameter tuning for networks customarily involves training multiple models in a search over a grid of parameter choices either randomly or exhaustively, and strategies applying complex search methods to identify candidate model architectures require significant computation for each possible architecture sampled in the model spaces. However, these approaches of extensively training many individual models in order to choose a single best performing model for future inference can seem unnecessarily wasteful at a time when energy efficiency and minimizing computing's environmental impact are increasingly important. Techniques or algorithms that reduce the computational budget to identify and train accurate deep networks among many options are of great need. This work considers one recently proposed approach, Training Speed Estimation, alongside deep learning approaches for a common hydrometeor classification problem, hail prediction through semantic image segmentation. We apply this method to the training of a variety of segmentation models and evaluate its effectiveness as a performance tracking approach for energy-aware neural network applications. This approach, together with early-stopping, offers a straightforward strategy for minimizing energy expenditure. By measuring consumption and estimating the level of energy savings, we are able to characterize this strategy as a practical method for minimizing deep learning's energy and carbon impact.
更多查看译文
关键词
performance estimation,image segmentation training,weather radar algorithms,parameter choices,complex search methods,model spaces,single best performing model,energy efficiency,training speed estimation,deep learning approaches,semantic image segmentation,segmentation models,energy-aware neural network applications,energy expenditure,energy savings,hydrometeor classification problem
AI 理解论文
溯源树
样例
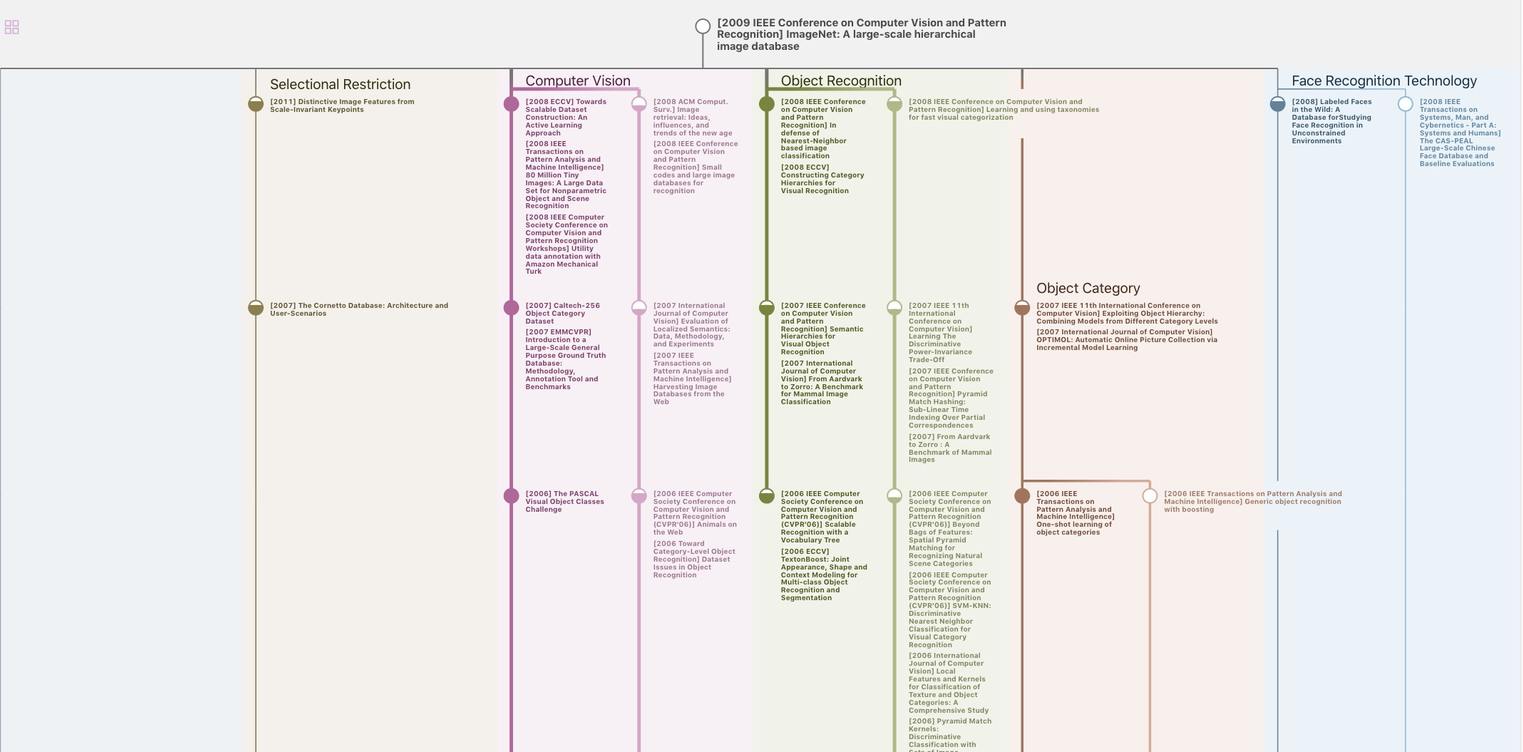
生成溯源树,研究论文发展脉络
Chat Paper
正在生成论文摘要